Your cart is currently empty!
Advancements in Deep Learning: The Impact of Recurrent Neural Networks
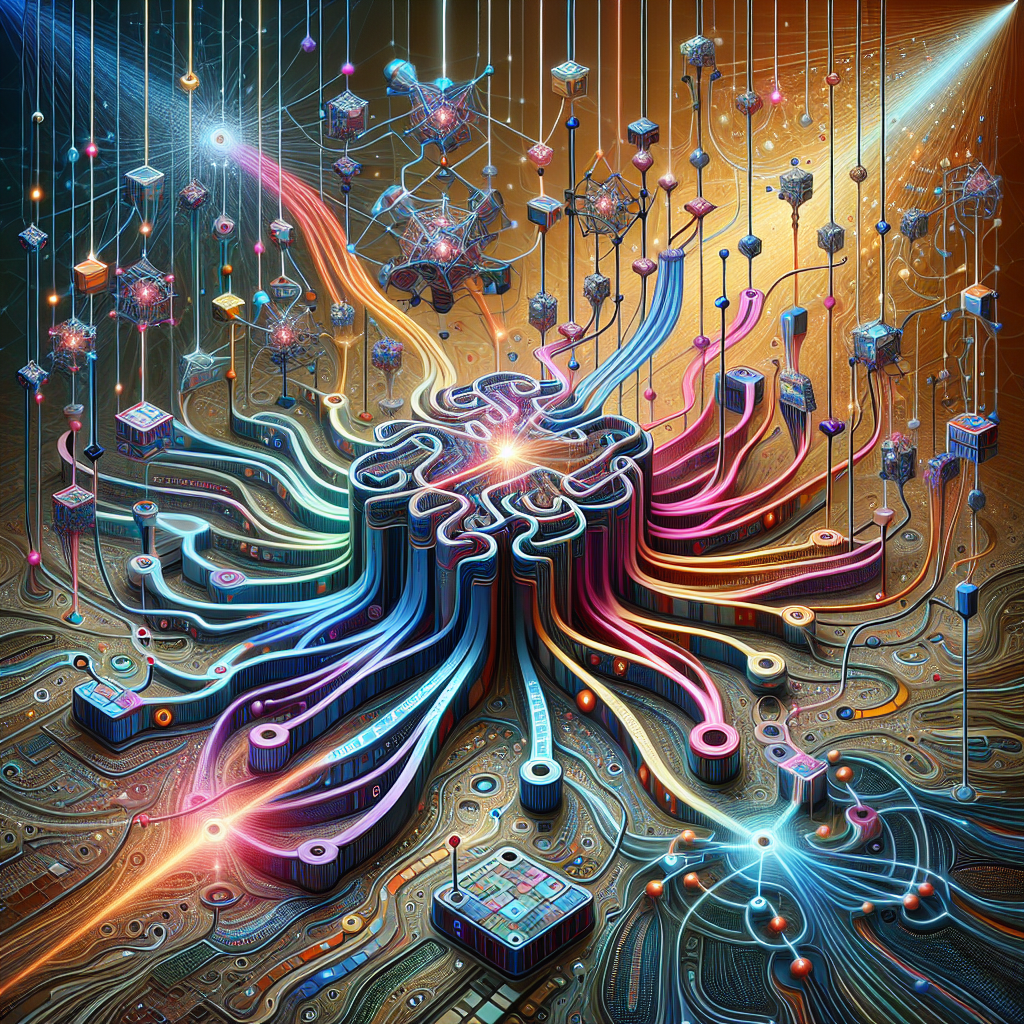
Deep learning has revolutionized the field of artificial intelligence, enabling machines to learn from vast amounts of data and make decisions without human intervention. One of the most significant advancements in deep learning is the development of recurrent neural networks (RNNs), which have greatly improved the ability of machines to process sequential data.
RNNs are a type of neural network that is designed to handle sequential data, such as time series data or natural language. Unlike traditional neural networks, which process input data in a static manner, RNNs have loops within their architecture that allow them to retain information about past inputs. This enables RNNs to learn patterns and relationships in sequential data, making them particularly well-suited for tasks such as speech recognition, language translation, and time series forecasting.
One of the key features of RNNs is their ability to remember and use information from previous time steps. This is achieved through the use of hidden states, which store information about past inputs and can be updated at each time step. By incorporating information from previous time steps, RNNs are able to capture long-range dependencies in sequential data, allowing them to make more accurate predictions and generate more coherent outputs.
The impact of RNNs on deep learning has been significant, leading to breakthroughs in a wide range of applications. For example, in natural language processing, RNNs have been used to improve the accuracy of language translation systems, enabling machines to generate more fluent and natural-sounding translations. In speech recognition, RNNs have been employed to improve the accuracy of voice recognition systems, making it easier for machines to understand and respond to spoken commands.
In addition to their applications in natural language processing and speech recognition, RNNs have also been used in time series forecasting, where they have been shown to outperform traditional forecasting methods. By capturing the complex relationships in sequential data, RNNs can make more accurate predictions about future trends, helping businesses and organizations make better decisions based on data.
Overall, the advancements in deep learning enabled by recurrent neural networks have had a profound impact on the field of artificial intelligence. By enabling machines to process sequential data more effectively, RNNs have opened up new possibilities for applications in areas such as natural language processing, speech recognition, and time series forecasting. As research in deep learning continues to advance, we can expect to see even more innovations and improvements in the capabilities of recurrent neural networks, further expanding the potential of artificial intelligence to transform industries and improve our daily lives.
#Advancements #Deep #Learning #Impact #Recurrent #Neural #Networks,rnn
Leave a Reply