Your cart is currently empty!
Advancements in NLP and Speech Recognition through Deep Learning Techniques
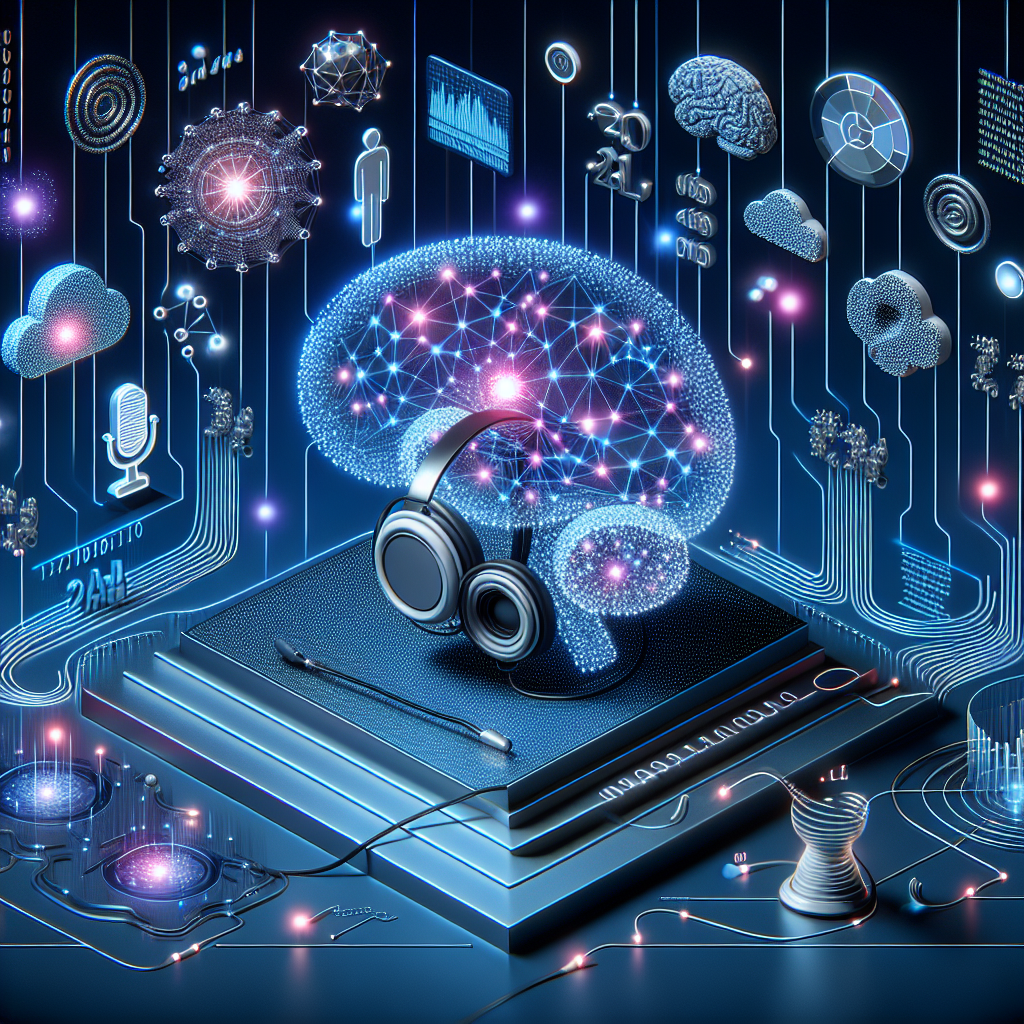
In recent years, there have been significant advancements in the field of Natural Language Processing (NLP) and Speech Recognition, thanks to the adoption of deep learning techniques. Deep learning, a subset of machine learning, has revolutionized the way computers process and understand human language, making NLP and Speech Recognition more accurate and efficient than ever before.
One of the key advancements in NLP through deep learning techniques is the development of neural networks, specifically Recurrent Neural Networks (RNNs) and Long Short-Term Memory (LSTM) networks. These networks are designed to process sequential data, making them ideal for tasks such as language modeling, sentiment analysis, and machine translation. By feeding large amounts of text data into these networks, they can learn the underlying patterns and structures of language, enabling them to generate more human-like responses and understand context better.
Another significant advancement in NLP is the use of transformer models, such as BERT (Bidirectional Encoder Representations from Transformers) and GPT (Generative Pre-trained Transformer). These models have achieved state-of-the-art results in various NLP tasks, including text classification, question answering, and language generation. By pre-training these models on large text corpora, they can capture rich semantic information and context, allowing them to perform well on a wide range of NLP tasks.
Speech Recognition has also seen major improvements through the use of deep learning techniques, particularly with the rise of deep neural networks and convolutional neural networks. These networks are trained on large speech datasets to learn the acoustic features of speech signals, enabling them to transcribe spoken language with high accuracy. Additionally, the development of end-to-end speech recognition systems, which integrate feature extraction, acoustic modeling, and language modeling into a single neural network architecture, has further improved the performance of speech recognition systems.
Overall, the advancements in NLP and Speech Recognition through deep learning techniques have significantly improved the capabilities of these technologies, making them more accurate, efficient, and capable of handling complex language tasks. As researchers continue to push the boundaries of deep learning in these fields, we can expect even more exciting developments in the future, paving the way for more intelligent and interactive AI systems.
#Advancements #NLP #Speech #Recognition #Deep #Learning #Techniques,deep learning for nlp and speech recognition
Leave a Reply