Your cart is currently empty!
Advancements in NLP through GANs: A Deep Dive into Generative Adversarial Networks and their Impact
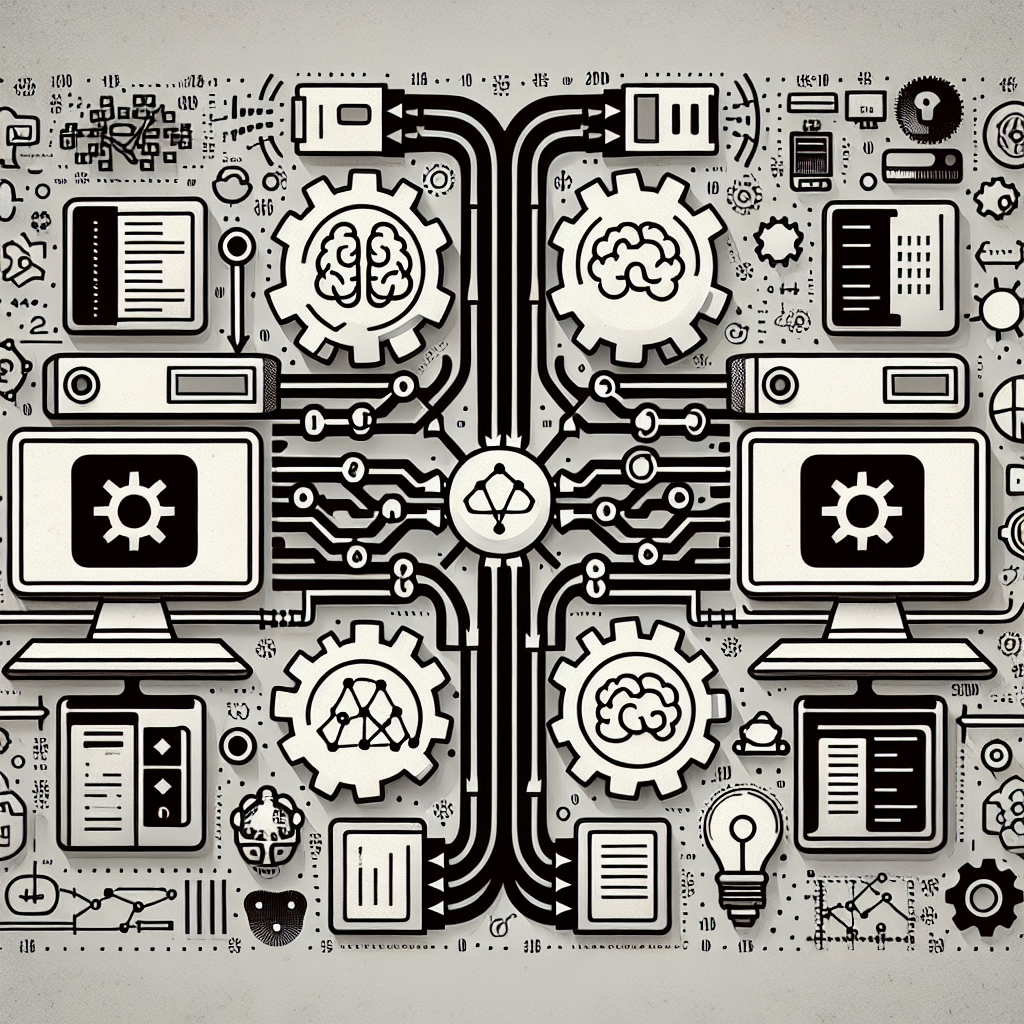
In recent years, advancements in natural language processing (NLP) have been driven by the development and application of generative adversarial networks (GANs). GANs, a type of deep learning model, have shown great potential in improving the performance of NLP tasks such as language generation, translation, and summarization.
Generative adversarial networks consist of two neural networks – a generator and a discriminator – that are trained simultaneously in a competitive manner. The generator generates new data samples, in this case, text, while the discriminator evaluates the generated samples and provides feedback to the generator on how to improve.
One of the key advantages of using GANs in NLP is their ability to generate more realistic and diverse text compared to traditional models. GANs can capture the underlying distribution of language data and produce more coherent and natural language outputs. This has led to significant improvements in tasks such as text generation, where GANs can generate more fluent and contextually relevant text.
Another major impact of GANs in NLP is their ability to improve data augmentation and synthesis. GANs can generate synthetic data samples that can be used to augment training data for NLP models, leading to better generalization and performance on various tasks. This is particularly useful in scenarios where labeled data is scarce or expensive to obtain.
Furthermore, GANs have also been applied to improve the quality of machine translation systems. By generating more diverse and natural translations, GANs have shown to enhance the fluency and accuracy of translated text, making them more human-like and easier to understand.
Overall, the advancements in NLP through GANs have opened up new possibilities for improving the performance and capabilities of language processing systems. By leveraging the power of generative adversarial networks, researchers and developers can continue to push the boundaries of what is possible in NLP and create more sophisticated and effective language models. As GANs continue to evolve and improve, we can expect even more exciting developments in the field of NLP in the years to come.
#Advancements #NLP #GANs #Deep #Dive #Generative #Adversarial #Networks #Impact,gan)
to natural language processing (nlp) pdf
Leave a Reply