Your cart is currently empty!
Advancing Language Understanding with GANs in Natural Language Processing
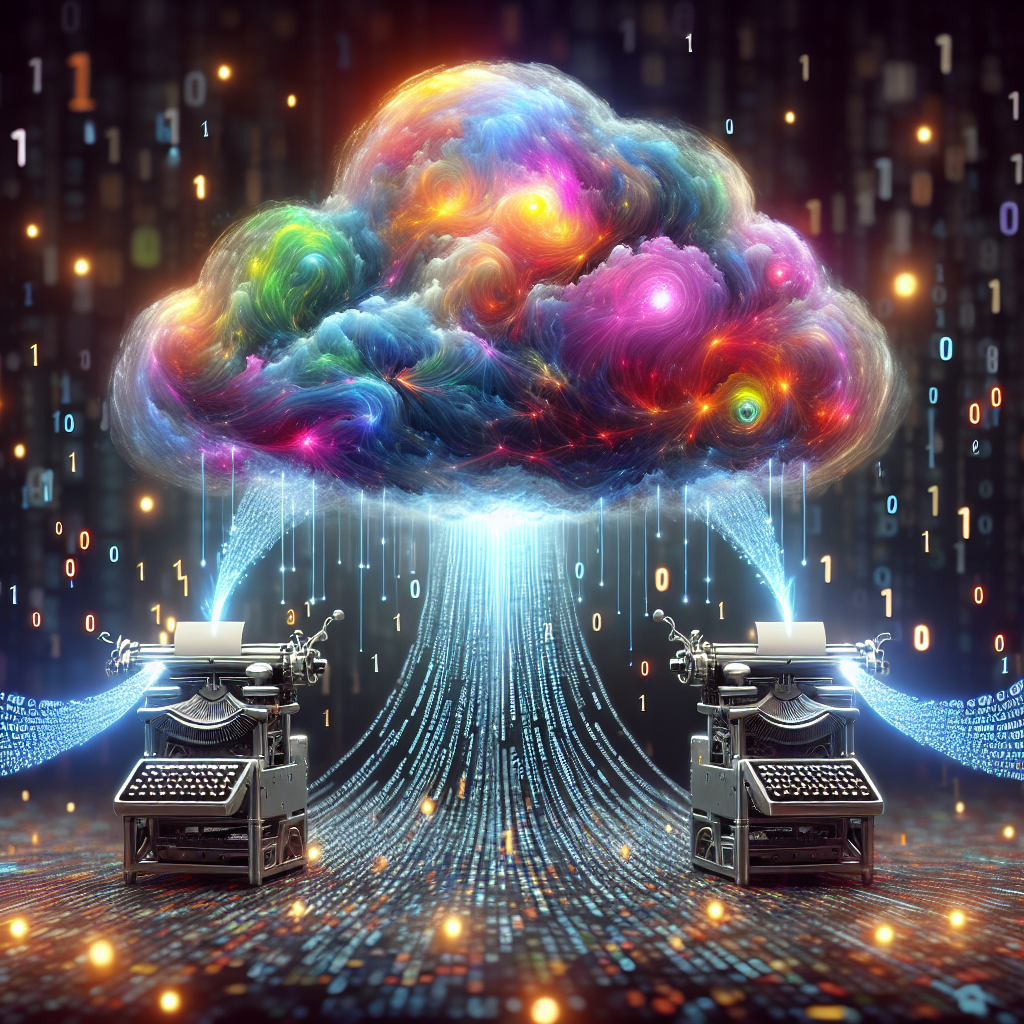
Advancing Language Understanding with GANs in Natural Language Processing
Natural Language Processing (NLP) is a field of artificial intelligence that focuses on the interaction between computers and humans through natural language. With the rise of deep learning techniques, NLP has seen significant advancements in recent years, enabling machines to understand and generate human language more effectively. One of the most promising techniques in this field is the use of Generative Adversarial Networks (GANs) to enhance language understanding.
GANs are a type of neural network architecture that consists of two networks: a generator and a discriminator. The generator creates new data samples, while the discriminator evaluates whether the generated samples are real or fake. Through a process of competition, the generator learns to produce increasingly realistic samples, while the discriminator becomes more adept at distinguishing between real and generated data.
In the context of NLP, GANs have been used to improve various language processing tasks, such as text generation, machine translation, and sentiment analysis. One of the key advantages of using GANs in NLP is their ability to generate diverse and realistic text samples, which can help in training language models to better understand and generate human language.
For example, researchers have used GANs to generate coherent and contextually relevant responses in conversational agents, also known as chatbots. By training a GAN on a large corpus of human conversations, the generator can learn to produce natural-sounding responses that are more engaging and realistic than traditional rule-based approaches.
GANs have also been applied to machine translation tasks, where they can help improve the fluency and accuracy of translated text. By training a GAN on parallel corpora of source and target languages, the generator can learn to produce translations that are more natural and idiomatic, leading to better overall translation quality.
In sentiment analysis, GANs can be used to generate synthetic text samples that capture the nuances and subtleties of human emotions. By training a GAN on labeled sentiment data, the generator can learn to produce text samples that convey different sentiments, such as positive, negative, or neutral, with greater accuracy and diversity.
Overall, GANs hold great promise for advancing language understanding in NLP by enabling machines to generate more realistic and contextually relevant text. By leveraging the power of adversarial training, researchers can train language models that are better equipped to understand and generate human language in a way that is both accurate and engaging. As the field of NLP continues to evolve, GANs are likely to play a key role in shaping the future of language processing technologies.
#Advancing #Language #Understanding #GANs #Natural #Language #Processing,gan)
to natural language processing (nlp) pdf
Leave a Reply