Your cart is currently empty!
Advancing NLP with GANs: A Review of State-of-the-Art Approaches and Applications
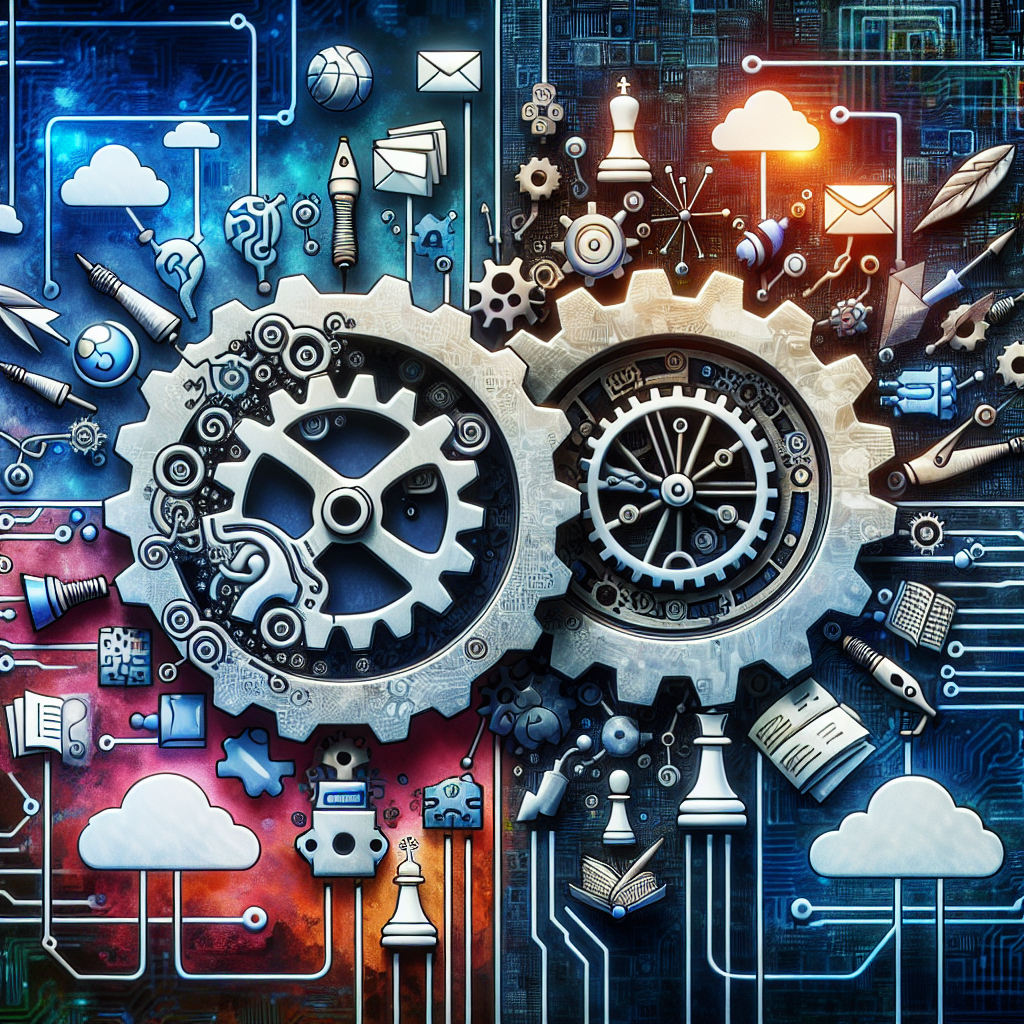
Advancing NLP with GANs: A Review of State-of-the-Art Approaches and Applications
Natural Language Processing (NLP) has seen significant advancements in recent years, thanks to the development of Generative Adversarial Networks (GANs). GANs, a type of artificial intelligence algorithm, have revolutionized the field of NLP by enabling the generation of realistic and coherent text. In this article, we will review the state-of-the-art approaches and applications of GANs in NLP.
One of the key advantages of using GANs in NLP is their ability to generate text that closely resembles human-written language. This has applications in various areas, such as machine translation, text summarization, and dialogue systems. GANs have also been used to improve the performance of existing NLP models by generating additional training data or fine-tuning model parameters.
One of the most popular approaches to using GANs in NLP is the text generation model, where a generator network generates text samples and a discriminator network evaluates the generated text for realism. Through an adversarial training process, the generator network learns to produce text that is indistinguishable from human-written text, while the discriminator network learns to distinguish between real and generated text.
Another approach is the conditional text generation model, where the generator network takes a given input and generates text based on that input. This approach has been used in tasks such as image captioning, where the input is an image and the generator generates a caption for that image.
GANs have also been used in style transfer tasks, where the style of a given text is changed to match a different style. This has applications in generating text in different writing styles or languages, or adapting text to a specific target audience.
In addition to text generation tasks, GANs have also been used in text classification tasks, where the discriminator network is trained to classify text into different categories. This has applications in sentiment analysis, topic modeling, and spam detection.
Overall, GANs have shown great promise in advancing NLP by enabling the generation of realistic and coherent text. With further research and development, GANs are likely to play an increasingly important role in NLP applications in the future.
#Advancing #NLP #GANs #Review #StateoftheArt #Approaches #Applications,gan)
to natural language processing (nlp) pdf
Leave a Reply