Your cart is currently empty!
Breaking Barriers with GANs: How Generative Adversarial Networks are Transforming Natural Language Processing
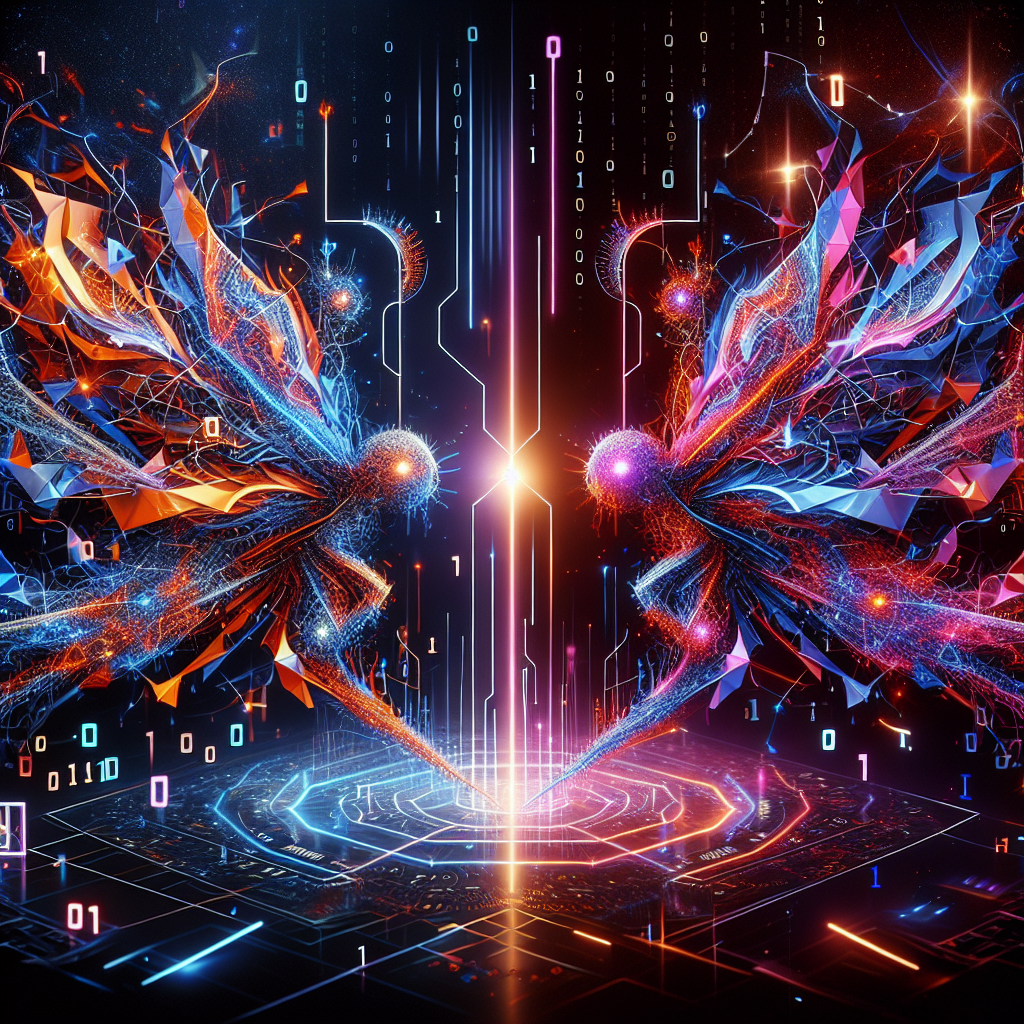
Generative Adversarial Networks (GANs) have been making waves in the field of artificial intelligence, particularly in the realm of image generation. However, recent advancements have shown that GANs are not just limited to creating realistic images – they are also transforming the way we process and generate natural language.
Natural Language Processing (NLP) is a subfield of artificial intelligence that focuses on the interaction between computers and human language. It encompasses a wide range of tasks, such as language translation, sentiment analysis, and text generation. Traditionally, NLP models have relied on statistical methods and rule-based systems to process and generate text. However, these approaches often struggle to capture the nuances and complexities of human language.
This is where GANs come in. GANs consist of two neural networks – a generator and a discriminator – that are pitted against each other in a game-like scenario. The generator creates samples of data (in this case, text) while the discriminator tries to distinguish between real and generated samples. Through this adversarial training process, the generator improves its ability to create realistic text, while the discriminator becomes better at detecting fake text.
One of the key benefits of using GANs for NLP tasks is their ability to generate more diverse and contextually relevant text. Traditional language models often produce generic or repetitive responses, whereas GANs can generate text that is more engaging and human-like. This is particularly useful for tasks such as dialogue generation, where the goal is to create natural-sounding conversations.
GANs have also been used to enhance existing NLP models. For example, researchers have combined GANs with recurrent neural networks (RNNs) to improve the fluency and coherence of text generation models. By training the generator to produce text that is more consistent with the input data, these hybrid models are able to generate more accurate and contextually relevant responses.
In addition to text generation, GANs are also being used to address other challenges in NLP, such as data augmentation and style transfer. Data augmentation involves generating additional training data to improve the performance of NLP models, while style transfer involves transferring the writing style of one text to another. GANs have shown promising results in both of these areas, demonstrating their versatility and potential in NLP applications.
Overall, GANs are breaking barriers in NLP by enabling more advanced and effective text generation models. As researchers continue to explore the capabilities of GANs in natural language processing, we can expect to see even more innovative applications and advancements in this field. With GANs leading the way, the future of NLP looks bright and full of possibilities.
#Breaking #Barriers #GANs #Generative #Adversarial #Networks #Transforming #Natural #Language #Processing,gan)
to natural language processing (nlp) pdf
Leave a Reply