Your cart is currently empty!
Bridging the Gap Between GANs and NLP: Innovations for Language Processing
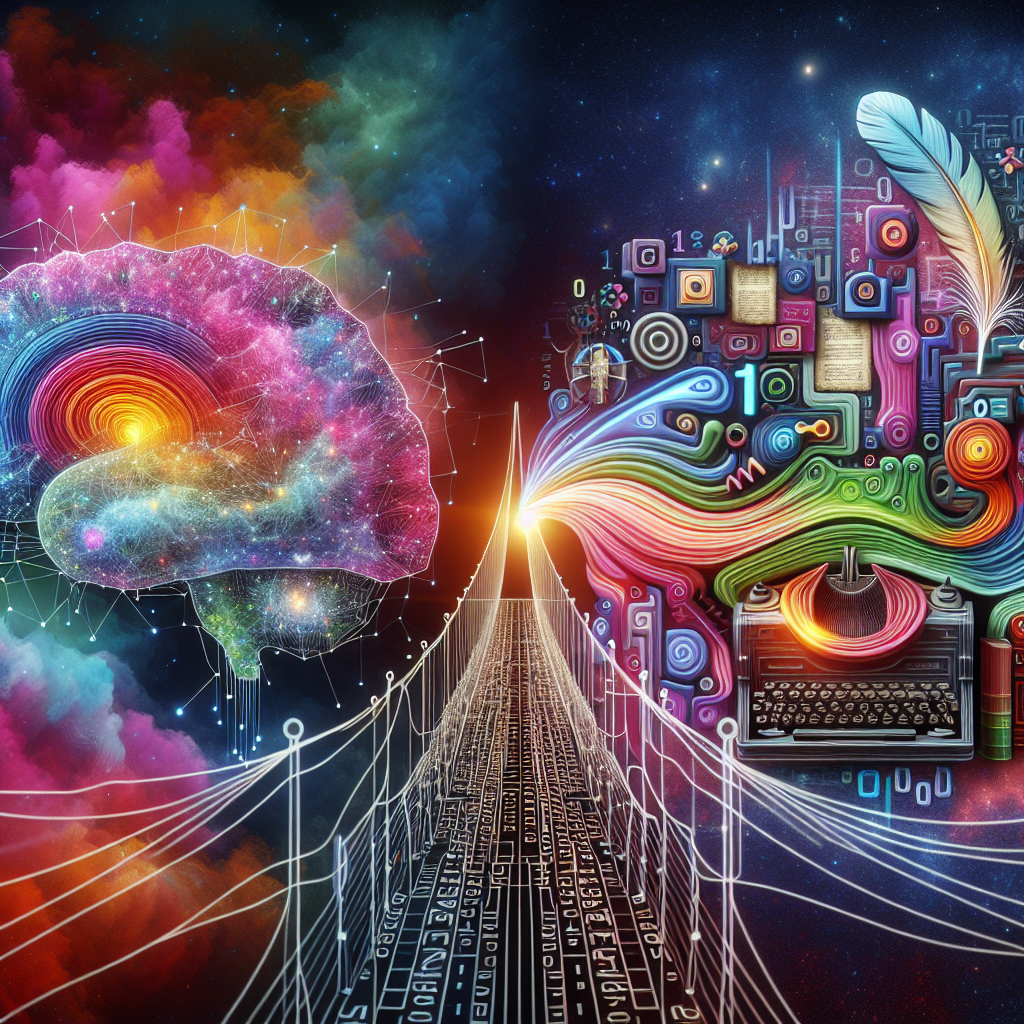
Generative Adversarial Networks (GANs) have gained widespread attention in the field of artificial intelligence for their ability to generate realistic data, such as images and text, using a combination of two neural networks: a generator and a discriminator. While GANs have been primarily used in image generation tasks, researchers are now exploring ways to bridge the gap between GANs and Natural Language Processing (NLP) to improve language processing capabilities.
One of the key challenges in applying GANs to NLP tasks is the discrete nature of text data, which is different from the continuous data typically used in image generation. However, recent advancements in GAN architectures and training techniques have enabled researchers to overcome this challenge and develop innovative solutions for language processing.
One of the most promising approaches to bridging the gap between GANs and NLP is the use of conditional GANs, where the generator is conditioned on a given input, such as a sentence or a sequence of words. This allows the generator to generate text that is coherent and relevant to the input, improving the quality of generated text.
Another innovative technique is the use of reinforcement learning to train GANs for language generation tasks. By using a reward signal to guide the generator towards generating more realistic and informative text, researchers have been able to improve the performance of GANs in NLP tasks such as text summarization and machine translation.
Furthermore, researchers are also exploring the use of GANs for data augmentation in NLP tasks, where synthetic data generated by GANs is used to supplement the training data and improve the performance of NLP models. This approach has been shown to be effective in tasks such as sentiment analysis and named entity recognition, where the availability of diverse and realistic data is crucial for model performance.
Overall, the bridging of GANs and NLP holds great promise for advancing the field of language processing. By leveraging the capabilities of GANs for generating realistic text data, researchers are able to develop more robust and accurate NLP models that can handle a wide range of language processing tasks. As research in this area continues to evolve, we can expect to see even more innovative applications of GANs in NLP, leading to significant advancements in natural language understanding and generation.
#Bridging #Gap #GANs #NLP #Innovations #Language #Processing,gan)
to natural language processing (nlp) pdf
Leave a Reply