Your cart is currently empty!
Communication Efficient Federated Learning for Wireless Networks
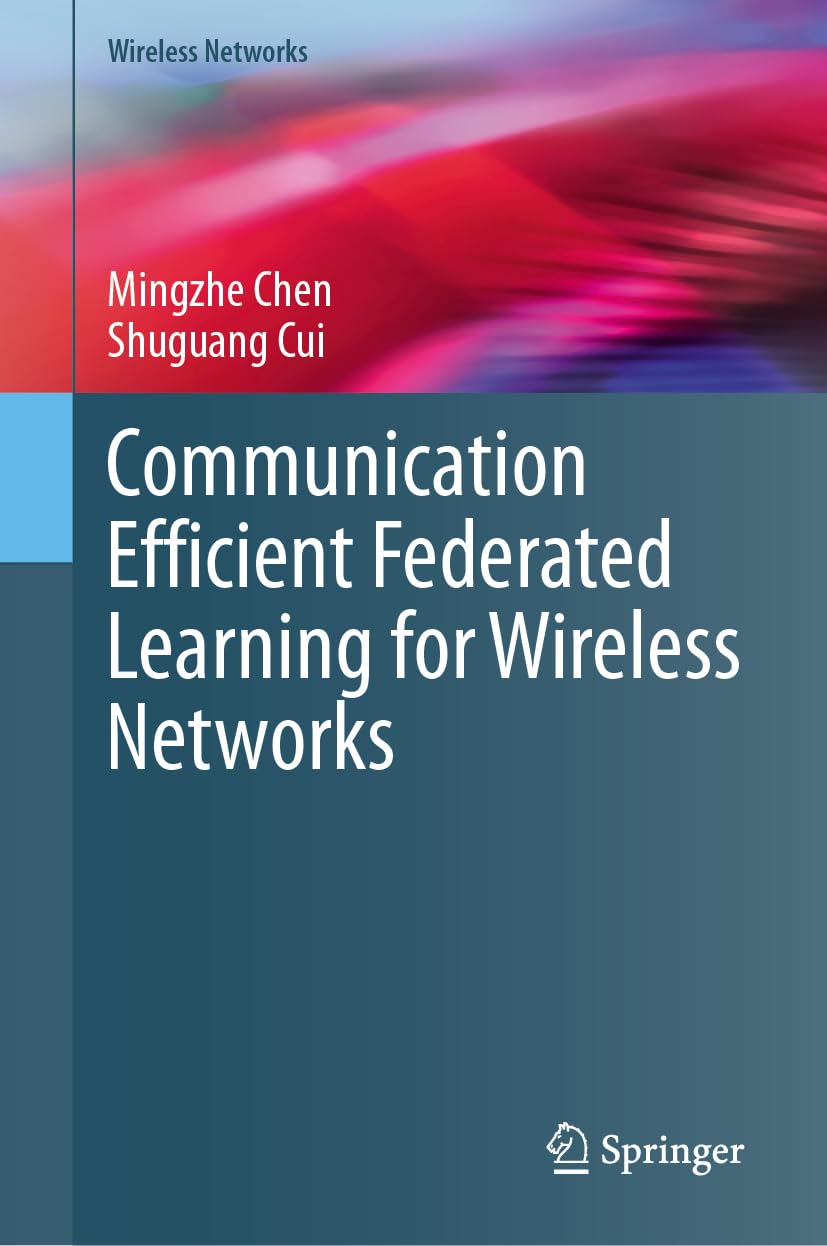
Price: $169.99 – $129.98
(as of Dec 15,2024 22:29:56 UTC – Details)
Publisher : Springer; 1st ed. 2024 edition (February 20, 2024)
Language : English
Hardcover : 190 pages
ISBN-10 : 3031512650
ISBN-13 : 978-3031512650
Item Weight : 15.8 ounces
Dimensions : 6.14 x 0.5 x 9.21 inches
Communication Efficient Federated Learning for Wireless Networks
Federated learning has emerged as a promising approach to train machine learning models across multiple decentralized devices, such as smartphones and IoT devices. However, in wireless networks where communication resources are limited, efficient communication is crucial for the success of federated learning.
In this post, we will discuss how communication can be optimized for federated learning in wireless networks to improve efficiency and reduce latency.
1. Model Compression: One way to reduce communication overhead in federated learning is to compress the model before sending it to the central server for aggregation. Techniques like quantization, pruning, and knowledge distillation can be used to compress the model without compromising its performance.
2. Differential Privacy: Privacy-preserving techniques like differential privacy can be used to add noise to the gradients before sending them to the central server. This helps protect the privacy of the individual devices’ data while still allowing for effective model training.
3. Adaptive Communication: Adaptive communication strategies, such as prioritizing communication with devices that have more relevant data or using different communication protocols based on the network conditions, can help reduce latency and improve efficiency in federated learning.
4. Edge Computing: By performing model training and aggregation at the edge devices instead of sending all the data to a central server, communication overhead can be reduced. Edge computing can also help in reducing latency and improving the overall performance of federated learning in wireless networks.
Overall, communication efficient federated learning is essential for enabling machine learning on resource-constrained devices in wireless networks. By implementing techniques like model compression, differential privacy, adaptive communication, and edge computing, we can optimize communication in federated learning and make it more efficient and scalable.
#Communication #Efficient #Federated #Learning #Wireless #Networks
Leave a Reply