Your cart is currently empty!
Data Observability for Data Engineering: Proactive strategies for ensuring data accuracy and addressing broken data pipelines
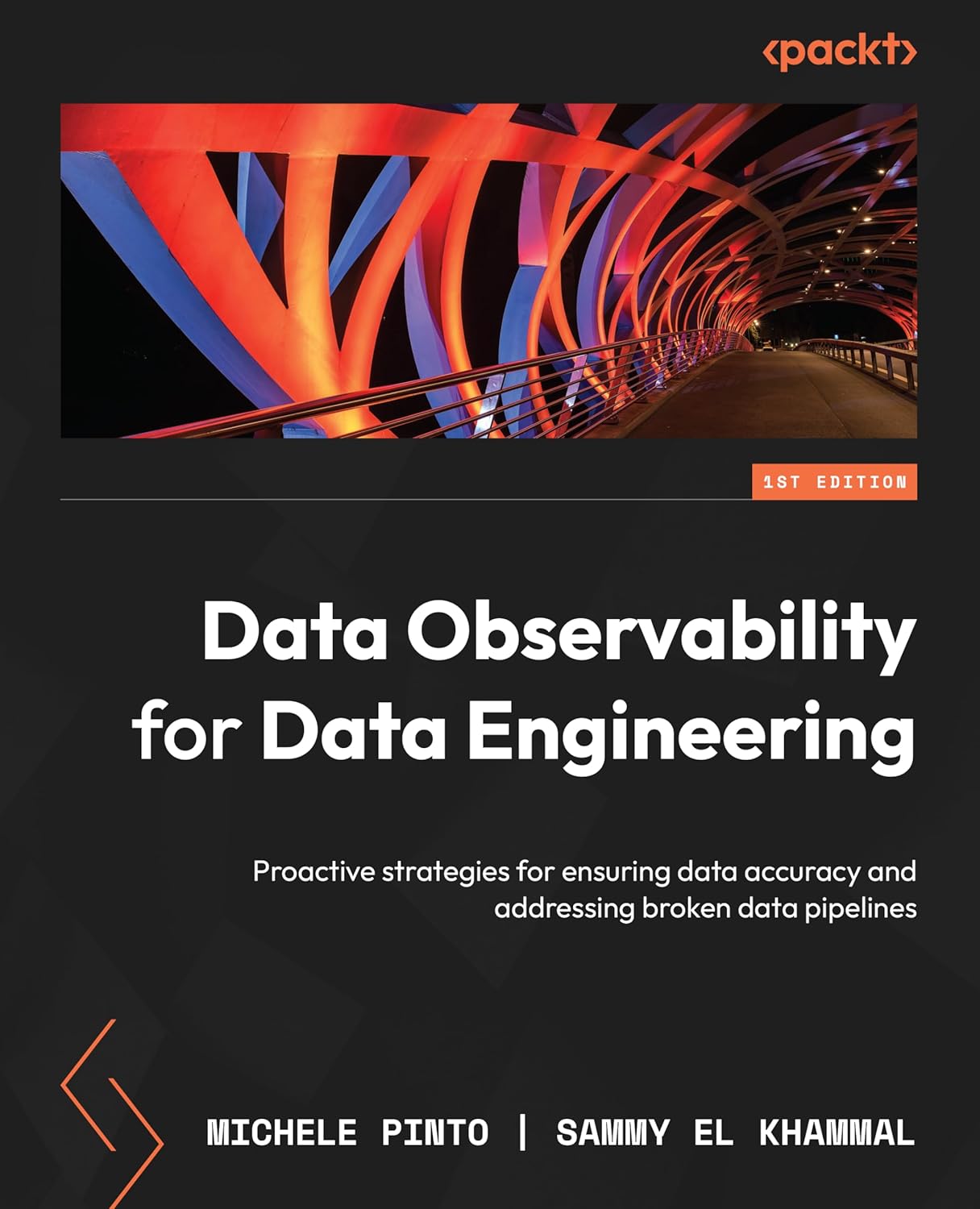
Price: $34.67
(as of Dec 27,2024 18:57:44 UTC – Details)
ASIN : B0B71HNZHR
Publisher : Packt Publishing; 1st edition (December 29, 2023)
Publication date : December 29, 2023
Language : English
File size : 8242 KB
Text-to-Speech : Enabled
Screen Reader : Supported
Enhanced typesetting : Enabled
X-Ray : Not Enabled
Word Wise : Not Enabled
Print length : 363 pages
Data observability is a critical component of any data engineering strategy, as it involves monitoring, analyzing, and troubleshooting data pipelines to ensure data accuracy and reliability. In today’s data-driven world, organizations rely on data to make informed decisions and drive business outcomes. However, without proper data observability practices in place, data engineers may face challenges in detecting and addressing issues in data pipelines, leading to inaccuracies, delays, and potentially costly errors.
To proactively address these challenges, data engineers can implement a set of strategies for ensuring data observability. Here are some key proactive strategies for data observability in data engineering:
1. Establish data quality metrics: Define and monitor key data quality metrics, such as completeness, accuracy, consistency, and timeliness, to ensure that data pipelines are functioning as expected. By establishing these metrics, data engineers can quickly identify any deviations or anomalies in the data and take corrective actions to address them.
2. Implement automated monitoring and alerting: Set up automated monitoring tools and alerts to detect issues in data pipelines in real-time. By leveraging monitoring tools, data engineers can proactively identify potential bottlenecks, failures, or performance issues in data pipelines and take immediate actions to resolve them before they impact the business.
3. Conduct regular data validation and testing: Perform regular data validation and testing to ensure the accuracy and integrity of data in the pipelines. By validating data against predefined rules, data engineers can identify discrepancies, missing values, or inconsistencies in the data and address them before they lead to downstream issues.
4. Document data lineage and dependencies: Document data lineage and dependencies across different stages of the data pipeline to understand the flow of data and identify potential points of failure. By mapping out data lineage, data engineers can trace data back to its source and pinpoint any issues or discrepancies that may arise along the way.
5. Collaborate cross-functionally: Foster collaboration and communication among data engineering, data science, and business teams to ensure alignment on data requirements, expectations, and priorities. By working together, teams can proactively address data quality issues, optimize data pipelines, and drive better outcomes for the organization.
In conclusion, data observability is a critical aspect of data engineering that requires proactive strategies to ensure data accuracy and reliability. By implementing these proactive strategies, data engineers can detect and address issues in data pipelines before they impact the business, ultimately driving better decision-making and business outcomes.
#Data #Observability #Data #Engineering #Proactive #strategies #ensuring #data #accuracy #addressing #broken #data #pipelines
Leave a Reply