Your cart is currently empty!
Designing Machine Learning Systems: An Iterative Process for Production-Ready Ap
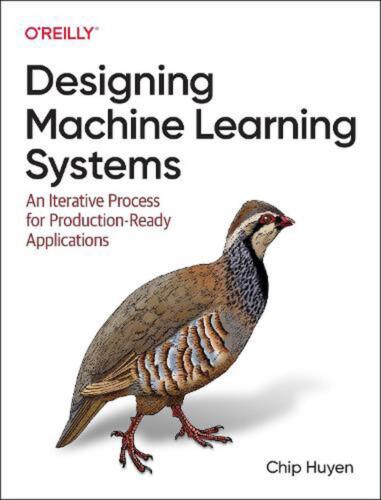
Designing Machine Learning Systems: An Iterative Process for Production-Ready Ap
Price : 55.67
Ends on : N/A
View on eBay
Machine learning systems have become an integral part of numerous industries, from healthcare to finance to marketing. However, designing and implementing these systems for production-ready applications can be a complex and challenging task. In this post, we will explore the iterative process of designing machine learning systems that are ready for production deployment.
The first step in designing a production-ready machine learning system is to clearly define the problem statement and objectives. This involves understanding the business requirements, identifying the target metrics, and defining the scope of the project. By clearly defining the problem statement, you can ensure that the machine learning system is aligned with the goals of the organization.
Once the problem statement is defined, the next step is to gather and preprocess the data. Data is the fuel that powers machine learning systems, so it is crucial to collect high-quality data that is relevant to the problem at hand. This may involve cleaning the data, handling missing values, and performing feature engineering to extract meaningful insights from the data.
After the data is preprocessed, the next step is to select and train a machine learning model. This involves choosing the appropriate algorithm, tuning hyperparameters, and evaluating the model’s performance using validation techniques such as cross-validation. It is important to iterate on the model selection and tuning process to ensure that the final model is robust and generalizes well to unseen data.
Once a satisfactory model is trained, the next step is to deploy the machine learning system into production. This may involve setting up a scalable infrastructure, monitoring the performance of the model in real-time, and integrating the system with existing software applications. It is important to thoroughly test the system before deployment to ensure that it meets the requirements of the organization.
After the machine learning system is deployed, the final step is to monitor and maintain the system on an ongoing basis. This involves monitoring the performance of the model, retraining the model with new data periodically, and making updates to the system as needed. By following this iterative process of designing machine learning systems, you can ensure that your system is production-ready and delivers value to the organization.
#Designing #Machine #Learning #Systems #Iterative #Process #ProductionReady
Leave a Reply