Your cart is currently empty!
Enhancing Text Analysis with GANs: An Overview of Current Trends and Developments
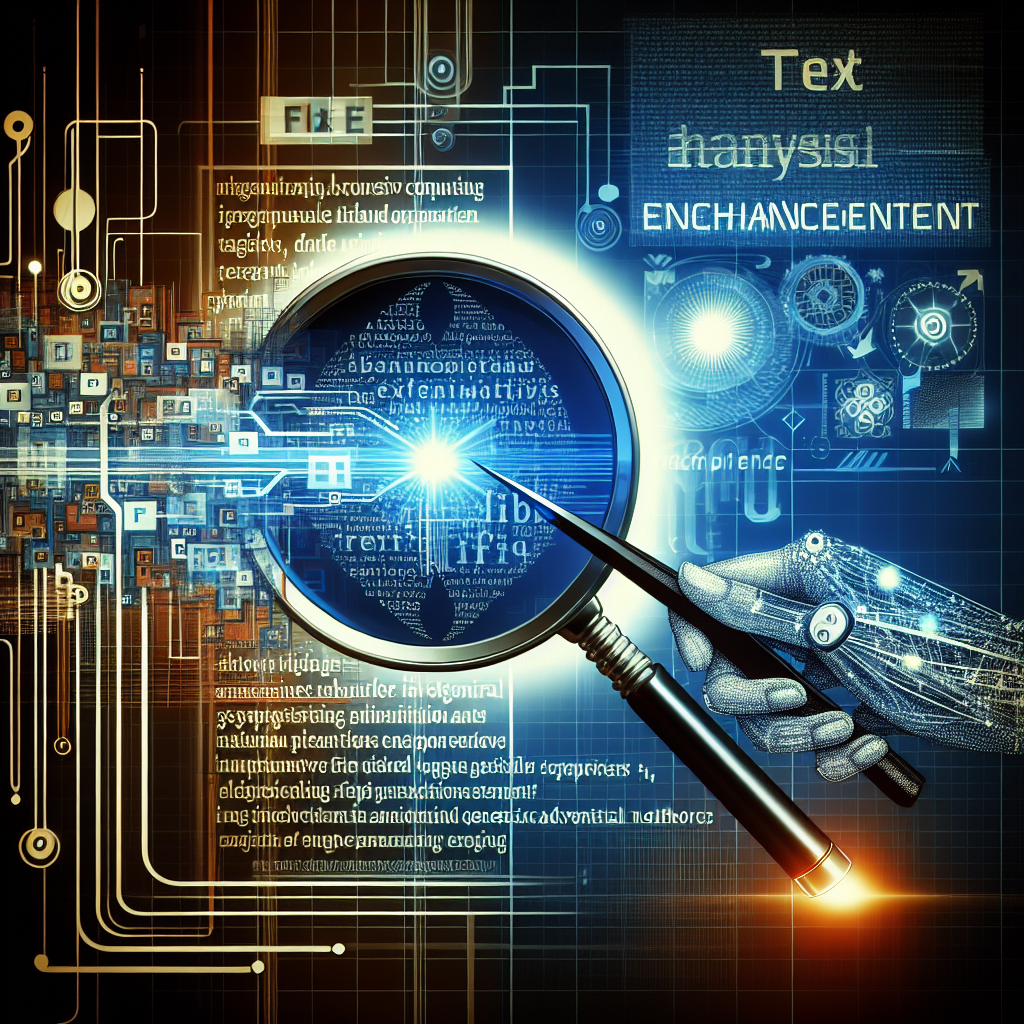
Text analysis has become an increasingly important tool in various industries, from marketing to healthcare to finance. With the explosion of data available online, businesses and organizations are turning to text analysis to extract valuable insights and make informed decisions. However, traditional text analysis techniques have their limitations, often struggling with complex, nuanced language and context.
To address these challenges, researchers and developers are turning to Generative Adversarial Networks (GANs) to enhance text analysis capabilities. GANs are a type of machine learning model that consists of two neural networks – a generator and a discriminator – that work together to generate realistic data. In the context of text analysis, GANs can be used to generate and analyze text data, improving the accuracy and efficiency of text analysis tasks.
One of the key benefits of using GANs for text analysis is their ability to generate synthetic text data that closely resembles real text data. This can be particularly useful in scenarios where there is a limited amount of labeled text data available, as GANs can generate additional data to train text analysis models. By generating synthetic data, GANs can also help improve the performance of text analysis models by providing them with more diverse and representative training data.
Another advantage of using GANs for text analysis is their ability to capture the underlying structure and patterns in text data. Traditional text analysis techniques often struggle with capturing the nuances and complexities of language, but GANs can learn to generate text that is more coherent and contextually relevant. This can help improve the accuracy of text analysis tasks such as sentiment analysis, topic modeling, and text classification.
In recent years, there has been a growing interest in using GANs for text analysis, with researchers developing a variety of novel approaches and techniques. For example, some researchers have explored the use of conditional GANs for text generation, where the generator is conditioned on specific input data to generate text that is more relevant to a given context. Others have investigated the use of adversarial training techniques to improve the robustness and generalization of text analysis models.
Overall, the use of GANs for text analysis shows great promise in enhancing the capabilities of text analysis models. By generating synthetic text data and capturing the underlying structure of text data, GANs can help improve the accuracy, efficiency, and robustness of text analysis tasks. As the field of text analysis continues to evolve, we can expect to see further advancements and developments in the use of GANs to enhance text analysis capabilities.
#Enhancing #Text #Analysis #GANs #Overview #Current #Trends #Developments,gan)
to natural language processing (nlp) pdf
Leave a Reply