Your cart is currently empty!
Explainable AI for Practitioners: Designing and Implementing Explainable ML
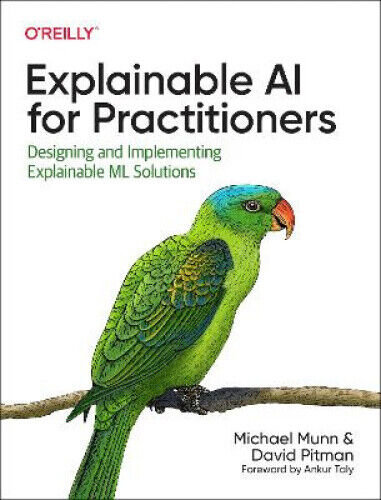
Explainable AI for Practitioners: Designing and Implementing Explainable ML
Price : 81.44 – 44.86
Ends on : N/A
View on eBay
Explainable AI for Practitioners: Designing and Implementing Explainable ML
Explainable AI, also known as XAI, is a critical component of machine learning systems that aims to make the decision-making process of AI models more transparent and understandable to humans. In recent years, there has been a growing interest in developing explainable machine learning (ML) techniques to address the “black box” nature of many AI systems.
Designing and implementing explainable ML models require a thoughtful approach that balances the need for accuracy and complexity with the need for transparency and interpretability. In this post, we will discuss some key principles and best practices for practitioners looking to incorporate explainable AI into their ML projects.
1. Start with a clear objective: Before diving into the design and implementation of an explainable ML model, it is essential to define the specific goals and requirements for explainability. Are you looking to understand how a model makes predictions, identify biases or errors, or provide insights to end-users? Having a clear objective will help guide the design process and ensure that the model meets the desired outcomes.
2. Choose the right explainability technique: There are various techniques available for explaining ML models, such as feature importance analysis, local interpretable model-agnostic explanations (LIME), and Shapley values. It is important to select the right technique based on the specific requirements of your project and the complexity of your model.
3. Validate and test the explainable model: Once you have designed and implemented an explainable ML model, it is crucial to validate and test its performance. This includes evaluating the accuracy of the explanations, testing for robustness and reliability, and assessing the impact on the overall model performance.
4. Communicate effectively: The ultimate goal of explainable AI is to make AI systems more transparent and understandable to humans. Therefore, it is essential to communicate the explanations in a clear and intuitive manner that is easily understandable to end-users. This may involve visualizations, interactive tools, or plain language explanations.
In conclusion, designing and implementing explainable ML models requires a thoughtful and systematic approach that considers the specific objectives, techniques, validation, and communication strategies. By incorporating explainable AI into ML projects, practitioners can enhance the transparency, trust, and usability of AI systems for a wide range of applications.
#Explainable #Practitioners #Designing #Implementing #Explainable
Leave a Reply