Your cart is currently empty!
Exploring the Applications of Recurrent Neural Networks in Time Series Analysis
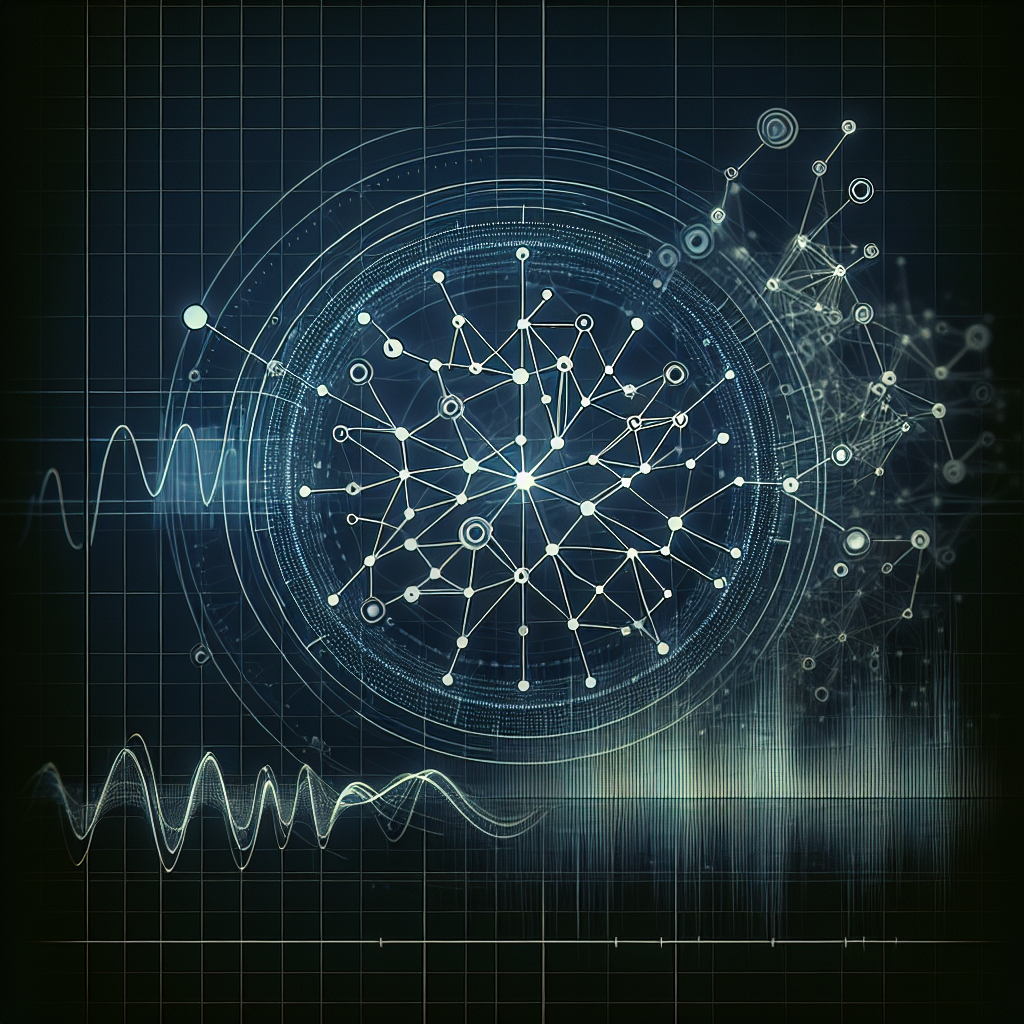
Recurrent Neural Networks (RNNs) have become increasingly popular in the field of time series analysis due to their ability to capture temporal dependencies in sequential data. Time series data, which is a sequence of observations recorded at regular time intervals, can be found in a wide range of fields such as finance, healthcare, weather forecasting, and more. RNNs are well-suited for analyzing this type of data as they are designed to handle sequences of variable length and can learn patterns and relationships within the data over time.
One of the main advantages of using RNNs for time series analysis is their ability to model complex temporal dynamics. Traditional machine learning models, such as linear regression or decision trees, assume that each data point is independent of the others. However, in time series data, each observation is often dependent on previous observations. RNNs address this issue by maintaining a state or memory of past inputs and using it to make predictions about future data points.
Applications of RNNs in time series analysis are diverse and have shown promising results in various domains. In finance, RNNs have been used to predict stock prices, market trends, and financial indicators. By analyzing historical data, RNNs can identify patterns and trends that can help investors make informed decisions. In healthcare, RNNs have been applied to predict patient outcomes, disease progression, and medical diagnoses. By analyzing patient data over time, RNNs can assist healthcare professionals in making accurate predictions and improving patient care.
Weather forecasting is another area where RNNs have been successfully applied. By analyzing historical weather data, RNNs can predict future weather patterns, such as temperature, precipitation, and wind speed. This information is crucial for various industries, including agriculture, transportation, and energy, as it can help them make informed decisions and adapt to changing weather conditions.
Overall, the applications of RNNs in time series analysis are vast and continue to grow as researchers and practitioners explore new ways to leverage the power of these neural networks. With their ability to model complex temporal dependencies, RNNs have the potential to revolutionize how we analyze and interpret time series data in various fields. As technology advances and more data becomes available, RNNs will play an increasingly important role in extracting valuable insights from time series data and driving innovation across industries.
#Exploring #Applications #Recurrent #Neural #Networks #Time #Series #Analysis,rnn
Leave a Reply