Your cart is currently empty!
Exploring the Power of GNN: How Graph Neural Networks Are Revolutionizing Machine Learning
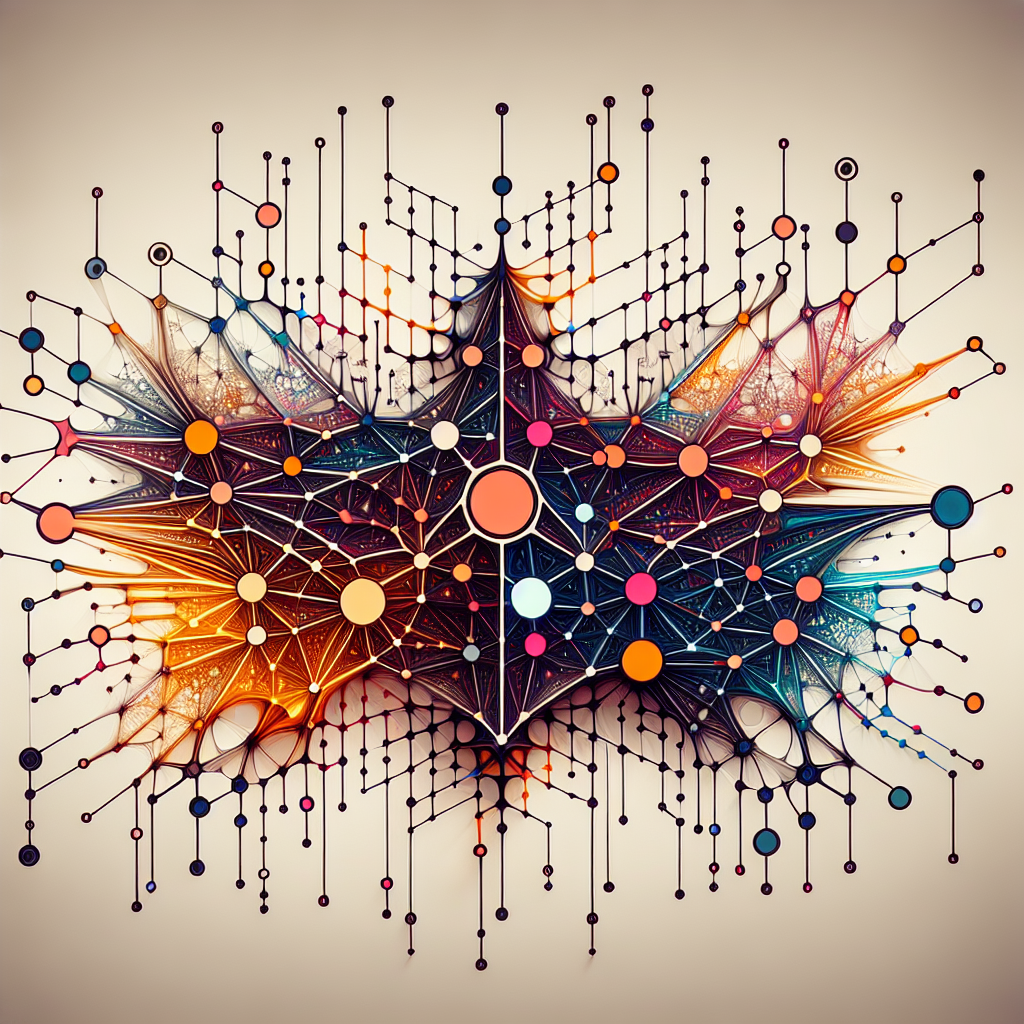
Graph Neural Networks (GNNs) have been gaining increasing attention in the field of machine learning for their ability to model complex relationships and dependencies in data. This revolutionary approach to machine learning is opening up new possibilities for solving a wide range of real-world problems.
At its core, a Graph Neural Network is a type of neural network that operates on graph-structured data, such as social networks, citation networks, or molecular structures. Traditional neural networks are designed to work with grid-structured data like images or text, making them less effective at capturing the inherent relationships between data points in a graph.
GNNs, on the other hand, are designed to leverage the graph structure of the data to learn and generalize patterns, making them well-suited for tasks like node classification, link prediction, and graph classification. By incorporating information about the relationships between nodes, GNNs can make more informed predictions and decisions based on the context of the entire graph.
One of the key advantages of GNNs is their ability to capture both local and global information in a graph. Traditional machine learning models often struggle with capturing long-range dependencies in data, but GNNs excel at learning from the entire graph structure, allowing them to make more accurate predictions.
Furthermore, GNNs can be easily adapted to different types of graph data and tasks, making them a versatile tool for a wide range of applications. From predicting protein structures in biology to recommending products in e-commerce, GNNs are revolutionizing the way we approach machine learning problems.
As the field of machine learning continues to evolve, the power of GNNs is becoming increasingly evident. Researchers and practitioners are exploring new ways to leverage the capabilities of GNNs to solve complex problems and drive innovation in various industries.
In conclusion, Graph Neural Networks are revolutionizing machine learning by enabling more effective modeling of complex relationships and dependencies in graph-structured data. With their ability to capture both local and global information, GNNs are opening up new possibilities for solving a wide range of real-world problems and driving innovation in the field of machine learning.
#Exploring #Power #GNN #Graph #Neural #Networks #Revolutionizing #Machine #Learning,gnn
Leave a Reply