Your cart is currently empty!
From GANs to NLP: Harnessing the Potential of Generative Adversarial Networks
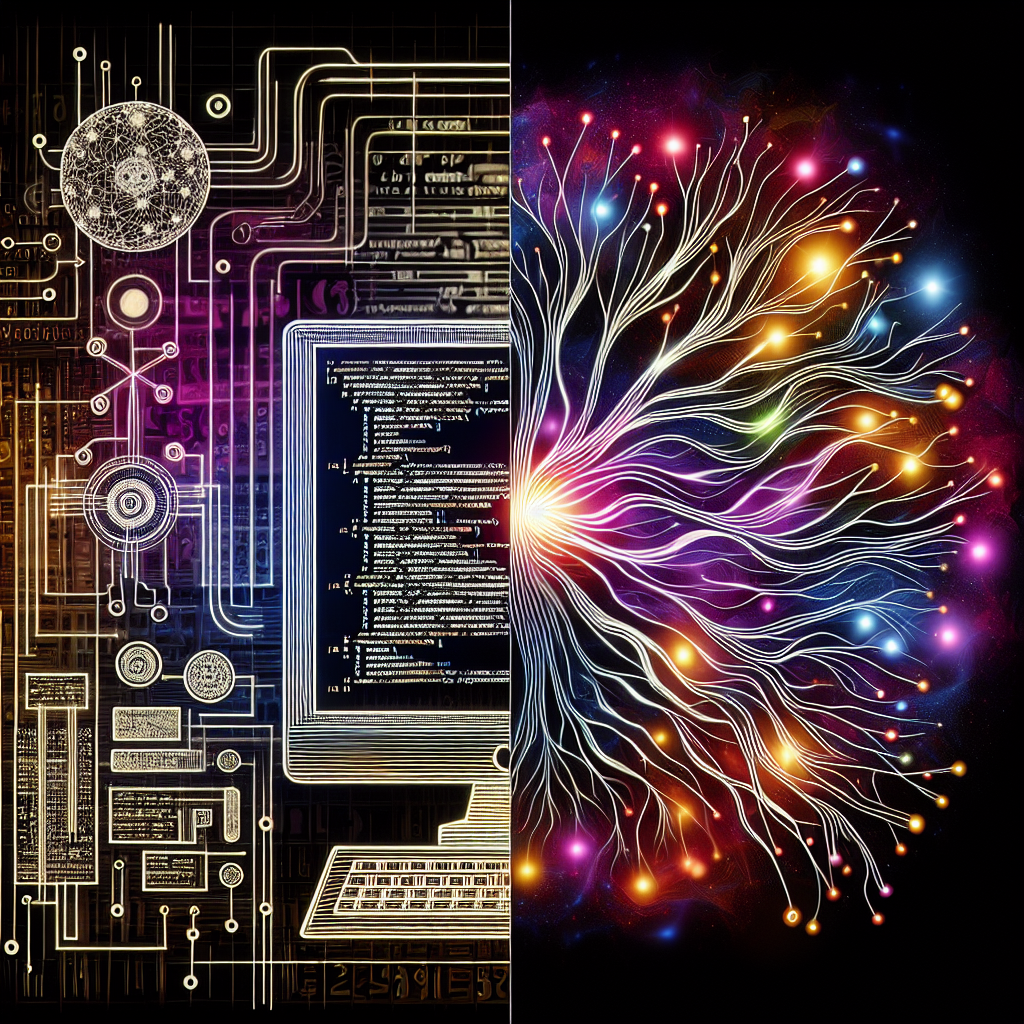
Generative Adversarial Networks (GANs) have been a revolutionary technology in the field of artificial intelligence, enabling the generation of realistic images, videos, and even text. However, their applications extend far beyond just creating visually appealing content. In recent years, researchers have been exploring the potential of GANs in the field of Natural Language Processing (NLP), opening up a whole new realm of possibilities.
One of the key advantages of using GANs in NLP is their ability to generate text that closely resembles human-written content. Traditional language models like LSTM and Transformer can generate coherent sentences, but they often lack the nuanced understanding of language that humans possess. GANs, on the other hand, can be trained to generate text that is indistinguishable from human-written content, making them ideal for tasks like text generation, summarization, and even translation.
Another advantage of using GANs in NLP is their ability to learn from unstructured data. Traditional NLP models require large amounts of annotated data to perform well, which can be a time-consuming and costly process. GANs, however, can be trained on unlabelled data, allowing them to learn from the vast amounts of text available on the internet. This makes them particularly well-suited for tasks like sentiment analysis, where large amounts of unlabelled data are readily available.
One of the most exciting applications of GANs in NLP is in the field of dialogue generation. By training GANs on conversational data, researchers have been able to create chatbots that can hold engaging and natural conversations with users. This has important implications for customer service, where chatbots can be used to provide instant support to customers, saving time and resources for businesses.
Despite their many advantages, GANs in NLP are not without their challenges. One of the main issues is the lack of interpretability in GAN-generated text. Unlike traditional language models, which can be easily analyzed and debugged, GAN-generated text is often opaque and difficult to understand. This makes it challenging to ensure that the text generated by GANs is accurate and reliable.
Another challenge is the potential for bias in GAN-generated text. Like all machine learning models, GANs are susceptible to biases in the data they are trained on. This can lead to the generation of biased or discriminatory text, which can have serious consequences in real-world applications.
Despite these challenges, the potential of GANs in NLP is vast. By harnessing the power of GANs, researchers have the opportunity to create truly human-like text generation models that can revolutionize the way we interact with machines. As the field of NLP continues to evolve, GANs will undoubtedly play a key role in shaping the future of artificial intelligence.
#GANs #NLP #Harnessing #Potential #Generative #Adversarial #Networks,gan)
to natural language processing (nlp) pdf
Leave a Reply