Your cart is currently empty!
GANs and NLP: A Winning Combination for Text Generation and Analysis
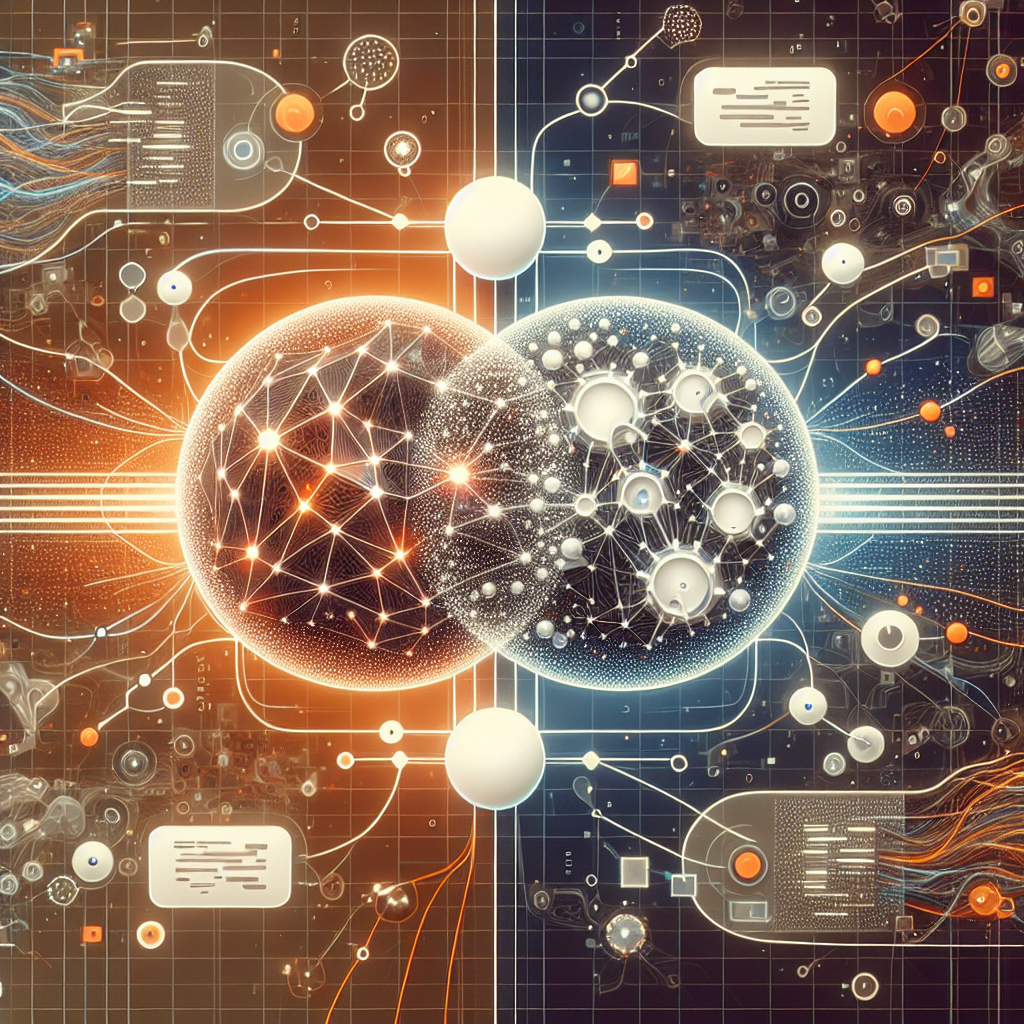
Generative Adversarial Networks (GANs) have been revolutionizing the field of artificial intelligence with their ability to generate realistic images, music, and even text. When combined with Natural Language Processing (NLP) techniques, GANs offer a powerful tool for text generation and analysis.
GANs consist of two neural networks – a generator and a discriminator – that work together in a competitive game. The generator creates samples that are then evaluated by the discriminator, which tries to distinguish between real and generated data. Through this process of competition and collaboration, GANs can produce high-quality outputs that are indistinguishable from real data.
In the context of NLP, GANs can be used to generate text that mimics the style and content of a given dataset. For example, GANs can be trained on a corpus of Shakespearean sonnets to generate new poems in the style of the Bard. This ability to create new text based on existing patterns is invaluable for tasks such as language translation, summarization, and dialogue generation.
Furthermore, GANs can also be used for text analysis and classification. By training a discriminator on a labeled dataset, GANs can learn to differentiate between different categories of text, such as spam emails or fake news articles. This can help in automating the process of content moderation and ensuring the quality and authenticity of textual data.
One of the key advantages of using GANs for text generation and analysis is their ability to capture intricate patterns and nuances in language. Traditional NLP models, such as recurrent neural networks (RNNs) and transformers, often struggle with generating coherent and contextually accurate text. GANs, on the other hand, excel at capturing the underlying structure of a dataset and producing outputs that are both realistic and diverse.
In conclusion, the combination of GANs and NLP holds great promise for advancing the field of text generation and analysis. By harnessing the power of generative adversarial networks, researchers and developers can create more sophisticated and human-like text models that can be used for a wide range of applications, from content generation to sentiment analysis. As this technology continues to evolve, we can expect to see even more exciting developments in the field of natural language processing.
#GANs #NLP #Winning #Combination #Text #Generation #Analysis,gan)
to natural language processing (nlp) pdf
Leave a Reply