Your cart is currently empty!
Generative Adversarial Networks and Deep Learning: Theory and Applications by Ro
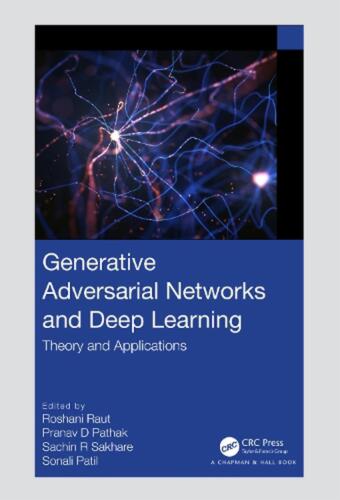
Generative Adversarial Networks and Deep Learning: Theory and Applications by Ro
Price : 100.71
Ends on : N/A
View on eBay
Generative Adversarial Networks (GANs) have revolutionized the field of deep learning by introducing a new framework for training generative models. In this post, we will explore the theory behind GANs and their applications in various domains.
GANs consist of two neural networks – a generator and a discriminator – that are trained simultaneously in a competitive manner. The generator learns to generate realistic samples from random noise, while the discriminator learns to distinguish between real and fake samples. Through this adversarial training process, GANs can learn to generate highly realistic data, such as images, text, and even music.
One of the key advantages of GANs is their ability to generate data that is indistinguishable from real data, making them ideal for tasks such as image generation, image editing, and data augmentation. GANs have been successfully applied in a wide range of domains, including computer vision, natural language processing, and healthcare.
In computer vision, GANs have been used to generate high-quality images, enhance image resolution, and perform image-to-image translation tasks. For example, GANs have been used to generate realistic faces, transform images from one style to another, and remove unwanted objects from images.
In natural language processing, GANs have been used to generate text, improve language models, and perform text-to-image generation tasks. GANs have been used to generate realistic text, translate text between languages, and create captions for images.
In healthcare, GANs have been used to generate medical images, predict patient outcomes, and perform data augmentation tasks. GANs have been used to generate realistic medical images, predict disease progression, and generate synthetic data for training deep learning models.
Overall, Generative Adversarial Networks have opened up new possibilities for deep learning and have shown great promise in a wide range of applications. As researchers continue to explore and refine GANs, we can expect to see even more exciting developments in the field of artificial intelligence.
#Generative #Adversarial #Networks #Deep #Learning #Theory #Applications
Leave a Reply