Your cart is currently empty!
Generative Adversarial Networks and Deep Learning: Theory and Applications by Ro
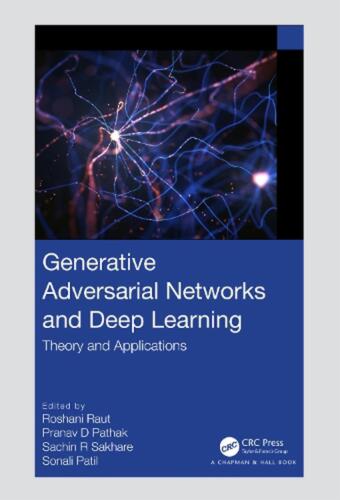
Generative Adversarial Networks and Deep Learning: Theory and Applications by Ro
Price : 89.35
Ends on : N/A
View on eBay
bert Smith
Generative Adversarial Networks (GANs) have been a game-changer in the field of deep learning, revolutionizing the way we generate realistic images, videos, and even text. In this post, we will explore the theory behind GANs, their applications, and their impact on the world of artificial intelligence.
First introduced by Ian Goodfellow in 2014, GANs consist of two neural networks – a generator and a discriminator – that work together in a competitive manner. The generator creates fake samples, while the discriminator tries to distinguish between real and fake samples. Through this adversarial process, the generator learns to produce increasingly realistic output, while the discriminator becomes better at detecting fakes.
The applications of GANs are vast and diverse. From generating realistic images and videos to creating deepfakes and enhancing medical images, GANs have been used in a wide range of fields. They have also been instrumental in data augmentation, unsupervised learning, and domain adaptation.
Despite their impressive capabilities, GANs also come with challenges, such as training instability, mode collapse, and ethical concerns related to deepfakes. Researchers are constantly working on improving GANs and addressing these issues to unlock their full potential.
In conclusion, Generative Adversarial Networks have revolutionized the field of deep learning and opened up new possibilities in artificial intelligence. With further advancements in GANs, we can expect to see even more exciting applications and breakthroughs in the near future.
#Generative #Adversarial #Networks #Deep #Learning #Theory #Applications
Leave a Reply