Your cart is currently empty!
Hardware Architectures for Deep Learning (Materials, Circuits and Devices)
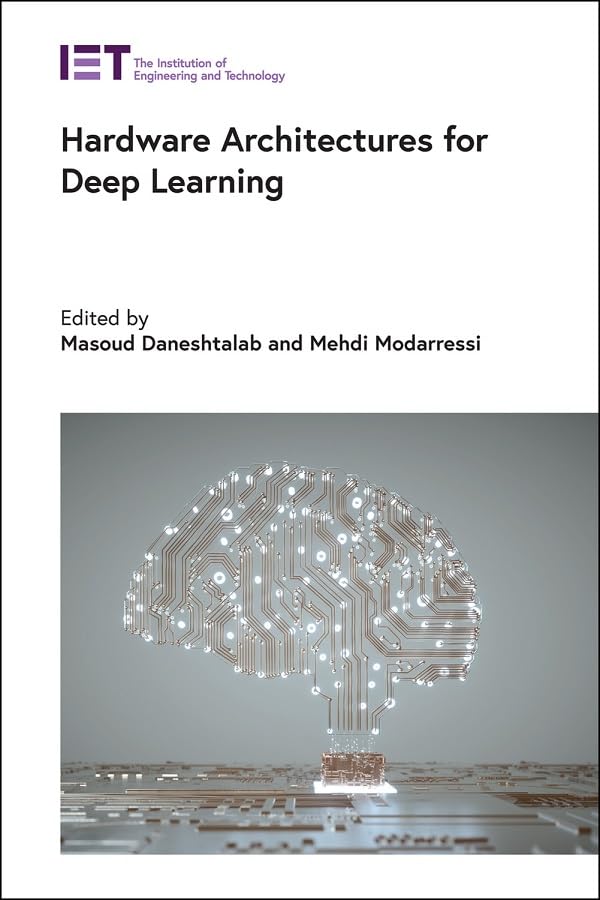
Price: $155.00 – $129.33
(as of Dec 26,2024 20:36:18 UTC – Details)
Publisher : The Institution of Engineering and Technology (April 24, 2020)
Language : English
Hardcover : 328 pages
ISBN-10 : 1785617680
ISBN-13 : 978-1785617683
Item Weight : 1.5 pounds
Dimensions : 6.3 x 0.8 x 9.4 inches
Hardware Architectures for Deep Learning: Exploring Materials, Circuits, and Devices
Deep learning has revolutionized the field of artificial intelligence, enabling computers to learn from vast amounts of data and make decisions without human intervention. One key factor in the success of deep learning algorithms is the hardware architecture on which they run.
In this post, we will explore the different materials, circuits, and devices that are used in hardware architectures for deep learning. From the silicon transistors in traditional CPUs to the specialized GPUs and TPUs designed specifically for deep learning tasks, there are a wide variety of hardware options available to researchers and practitioners in the field.
Materials such as silicon, gallium arsenide, and graphene have unique properties that make them well-suited for use in deep learning hardware. These materials can be used to create transistors, memory cells, and interconnects that are faster, more energy-efficient, and more reliable than traditional silicon-based components.
Circuits play a crucial role in the performance of deep learning hardware architectures. From the design of parallel processing units to the optimization of memory bandwidth and latency, circuit designers must carefully consider the trade-offs between performance, power consumption, and cost.
Devices such as GPUs (graphics processing units) and TPUs (tensor processing units) have been specifically designed to accelerate deep learning tasks. These devices typically feature thousands of processing cores that can perform matrix multiplication operations in parallel, making them ideal for tasks such as training neural networks.
As researchers continue to push the boundaries of deep learning, hardware architectures will play an increasingly important role in enabling new breakthroughs. By exploring the materials, circuits, and devices that underpin these architectures, we can better understand the challenges and opportunities in the field of deep learning hardware design.
#Hardware #Architectures #Deep #Learning #Materials #Circuits #Devices
Leave a Reply