Your cart is currently empty!
Harnessing the Power of GANs for Natural Language Generation in NLP
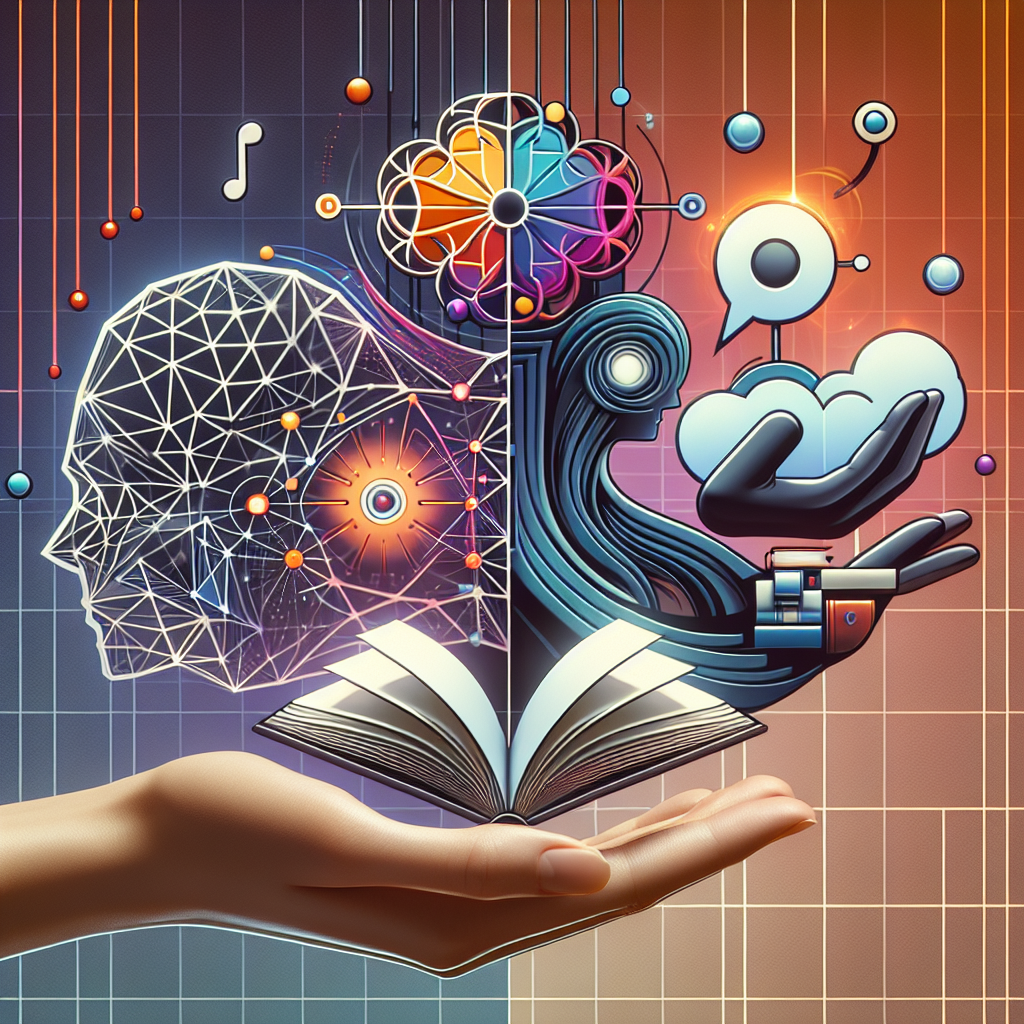
Generative Adversarial Networks (GANs) have revolutionized the field of artificial intelligence in recent years, particularly in the realm of image generation. However, their potential for natural language generation in NLP has only recently begun to be explored. Harnessing the power of GANs for NLP tasks has the potential to significantly improve the quality and diversity of generated text, making it a promising avenue for future research.
GANs consist of two neural networks – a generator and a discriminator – that are trained simultaneously in a competitive manner. The generator generates samples of data, such as images or text, while the discriminator evaluates the authenticity of these samples. Through this adversarial training process, the generator learns to produce increasingly realistic data, while the discriminator learns to distinguish between real and generated data.
In the context of NLP, GANs can be used for tasks such as text generation, machine translation, and dialogue generation. By generating text samples that are indistinguishable from real human-written text, GANs have the potential to improve the fluency, coherence, and diversity of generated text.
One of the key challenges in natural language generation is generating text that is both grammatically correct and semantically meaningful. Traditional language models, such as recurrent neural networks (RNNs) and transformers, often struggle with generating coherent and diverse text. GANs offer a promising solution to this problem by leveraging the adversarial training process to improve the quality of generated text.
In recent years, researchers have made significant progress in leveraging GANs for natural language generation. For example, researchers have developed GAN-based models for text generation that can generate realistic and diverse text samples. These models have been used for tasks such as dialogue generation, story generation, and image captioning, demonstrating the potential of GANs for improving the quality of generated text.
In addition to improving the quality of generated text, GANs can also be used to enhance the diversity and creativity of generated text. By training the generator to produce diverse text samples, GANs can generate text that is more engaging and interesting to read. This can be particularly useful for tasks such as dialogue generation and story generation, where diversity and creativity are important factors.
Overall, harnessing the power of GANs for natural language generation in NLP has the potential to significantly improve the quality, diversity, and creativity of generated text. As researchers continue to explore the capabilities of GANs for NLP tasks, we can expect to see further advancements in the field of natural language generation. By leveraging the adversarial training process of GANs, we can unlock new possibilities for generating high-quality text that is both grammatically correct and semantically meaningful.
#Harnessing #Power #GANs #Natural #Language #Generation #NLP,gan)
to natural language processing (nlp) pdf
Leave a Reply