Your cart is currently empty!
Harnessing the Power of LSTM: Applications in Time Series Forecasting
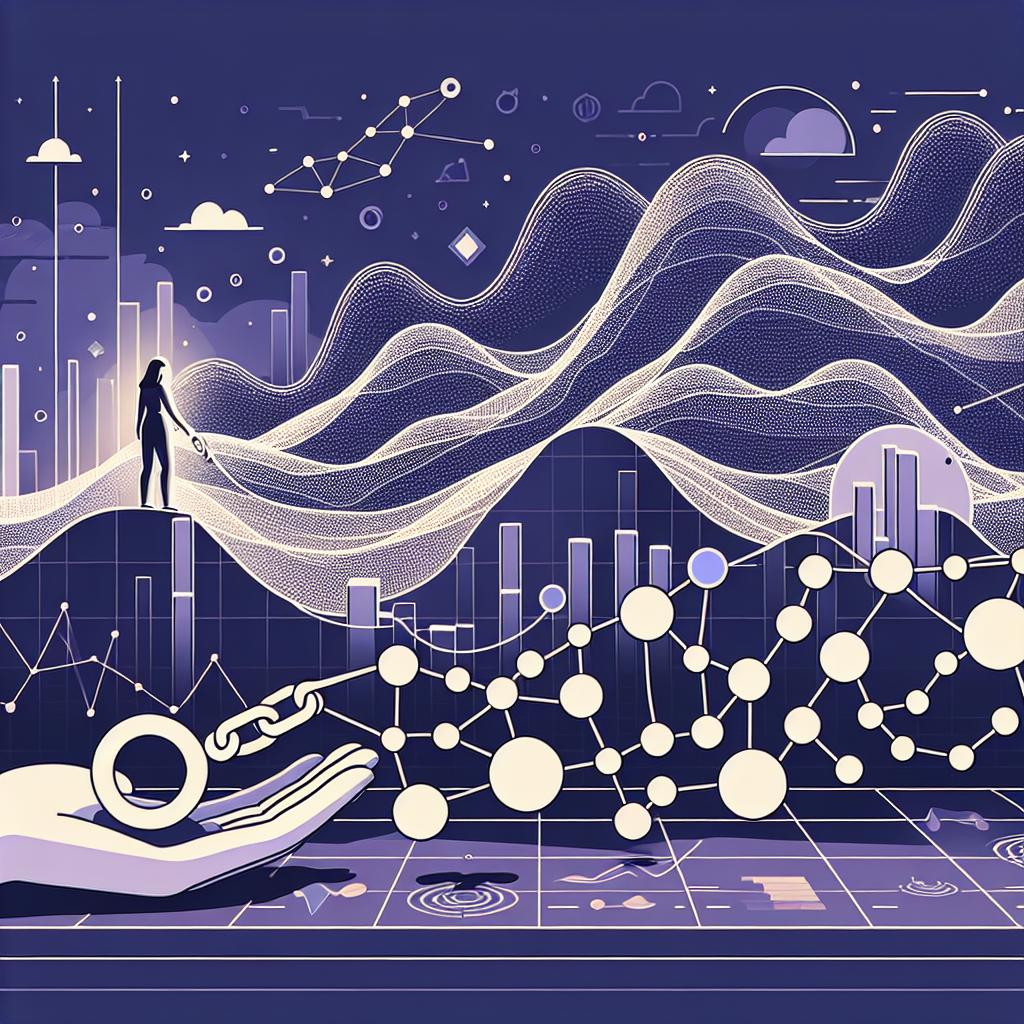
Long Short-Term Memory (LSTM) is a type of recurrent neural network architecture that has gained popularity in recent years for its ability to handle long-term dependencies in data sequences. LSTM networks are particularly well-suited for time series forecasting, as they can capture complex patterns and relationships in sequential data.
One of the key advantages of LSTM networks is their ability to retain information over long periods of time. This is achieved through the use of a memory cell, which can store information over multiple time steps and selectively update or forget this information based on the input data. This enables LSTM networks to learn from past data points and make more accurate predictions about future data points.
In the context of time series forecasting, LSTM networks have been successfully applied to a wide range of applications, including stock market prediction, weather forecasting, energy demand forecasting, and traffic prediction. By analyzing historical data and identifying patterns in the data sequence, LSTM networks can make accurate predictions about future values in the time series.
One of the key challenges in time series forecasting is the presence of noise and irregularities in the data. LSTM networks are able to handle noisy data and learn from the underlying patterns in the time series, making them well-suited for forecasting tasks in noisy environments.
In addition to their ability to handle long-term dependencies and noisy data, LSTM networks also have the advantage of being able to capture non-linear relationships in the data. This allows them to model complex patterns and relationships in the time series, leading to more accurate forecasts.
Overall, LSTM networks have proven to be a powerful tool for time series forecasting, with applications in a wide range of industries and domains. By harnessing the power of LSTM, organizations can improve their forecasting accuracy and make more informed decisions based on predictive analytics.
#Harnessing #Power #LSTM #Applications #Time #Series #Forecasting,lstm
Leave a Reply