Your cart is currently empty!
How LSTM Revolutionized Time Series Forecasting
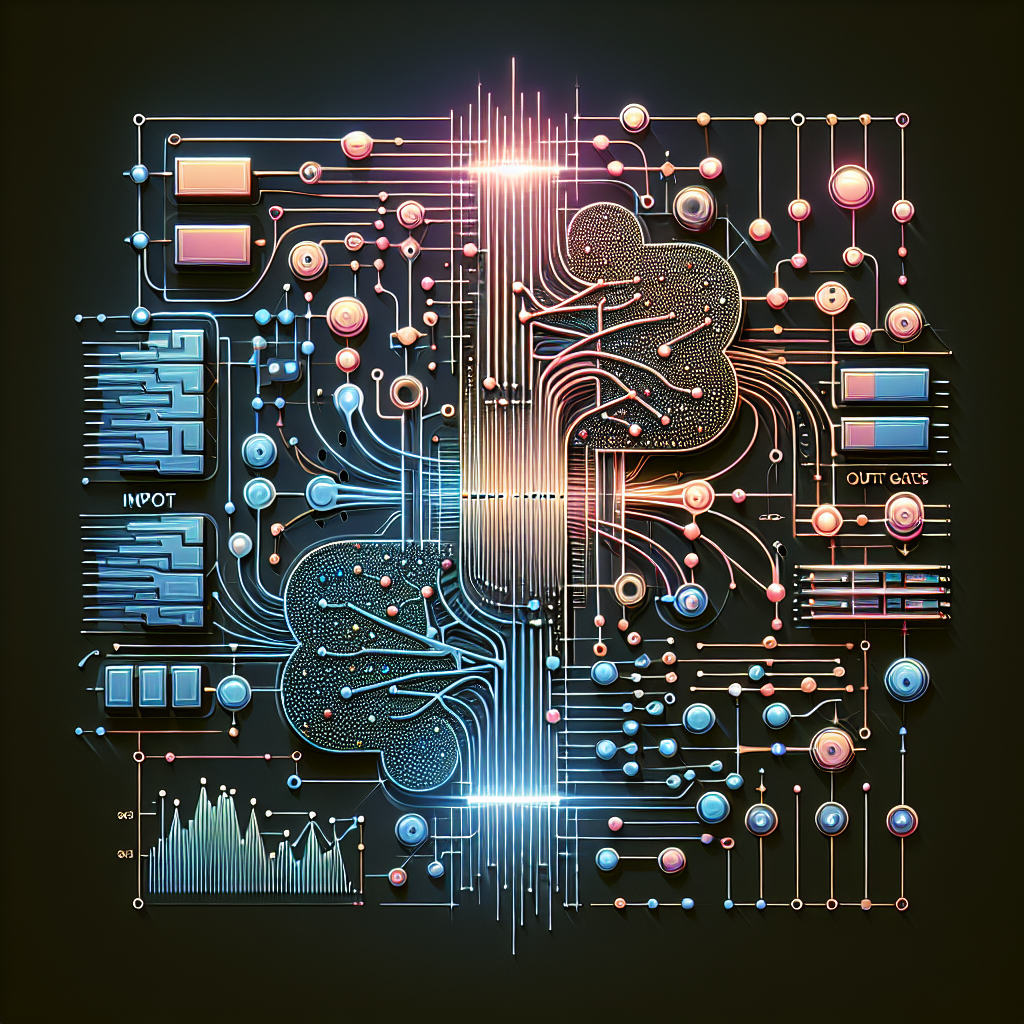
Time series forecasting is an important area of study in the field of data science and machine learning. It involves predicting future values of a time series based on past observations. In recent years, Long Short-Term Memory (LSTM) neural networks have revolutionized time series forecasting by providing more accurate and reliable predictions.
LSTMs are a type of recurrent neural network (RNN) that is designed to capture long-term dependencies in time series data. Traditional RNNs have difficulty learning long-term dependencies because they suffer from the vanishing gradient problem, where gradients become exponentially small as they are backpropagated through time. LSTMs address this issue by introducing a memory cell with self-gating mechanisms that allow it to retain information over long time periods.
One of the key advantages of LSTMs for time series forecasting is their ability to handle sequences of varying lengths. Traditional forecasting models like ARIMA or exponential smoothing require fixed-length input sequences, which can limit their effectiveness in capturing complex patterns in the data. LSTMs, on the other hand, can learn from sequences of any length and are able to capture both short-term and long-term dependencies in the data.
Another advantage of LSTMs is their ability to handle multiple input features. Time series data often contains multiple variables that can influence the forecasted values. LSTMs can easily incorporate these additional features into their model, allowing them to capture more complex relationships between the variables and improve the accuracy of the forecasts.
Additionally, LSTMs are well-suited for handling non-linear and non-stationary time series data. Traditional forecasting models assume linear relationships and stationary data, which can limit their performance on more complex and dynamic datasets. LSTMs are able to learn non-linear patterns in the data and adapt to changes in the underlying relationships over time, making them more robust and accurate for forecasting tasks.
Overall, LSTM neural networks have revolutionized time series forecasting by providing more accurate and reliable predictions. Their ability to capture long-term dependencies, handle sequences of varying lengths, incorporate multiple input features, and adapt to non-linear and non-stationary data make them a powerful tool for forecasting a wide range of time series datasets. As the field of data science continues to advance, LSTMs are likely to play an increasingly important role in shaping the future of time series forecasting.
#LSTM #Revolutionized #Time #Series #Forecasting,lstm
Leave a Reply