Your cart is currently empty!
How Recurrent Neural Networks Are Revolutionizing Time Series Analysis
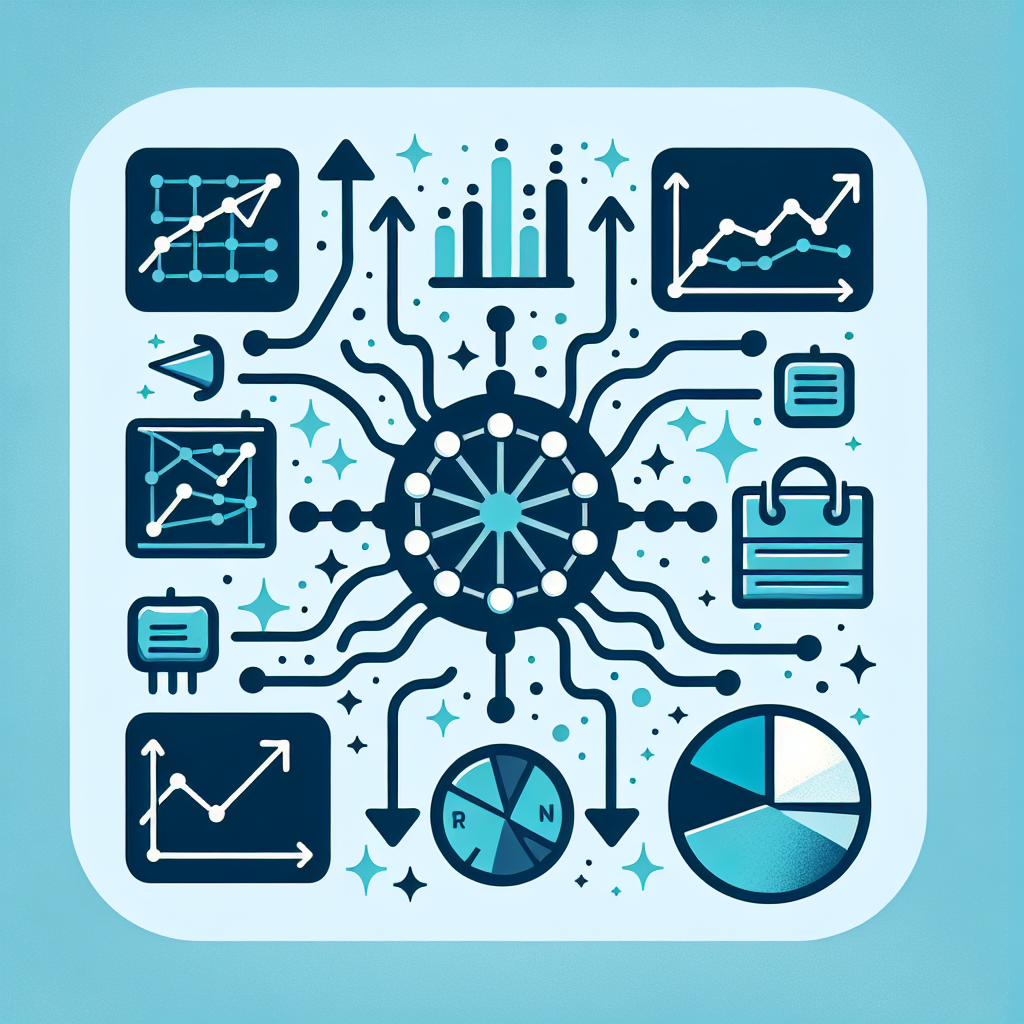
Time series analysis is a method used in various fields such as finance, economics, weather forecasting, and many others to make predictions based on historical data. Traditionally, time series analysis relied on statistical methods such as moving averages, autoregressive integrated moving average (ARIMA) models, and exponential smoothing techniques. However, with the advancement of artificial intelligence and machine learning, a new breed of models called recurrent neural networks (RNNs) are revolutionizing time series analysis.
RNNs are a type of artificial neural network designed to recognize patterns in sequential data, making them ideal for analyzing time series data. Unlike traditional feedforward neural networks, RNNs have connections that form loops, allowing them to retain information about previous inputs. This ability to remember past information makes RNNs well-suited for analyzing time series data, where the order of the data points is crucial.
One of the key advantages of RNNs in time series analysis is their ability to capture long-term dependencies in the data. Traditional statistical methods often struggle to capture complex patterns in time series data that span across multiple time steps. RNNs, on the other hand, excel at capturing these long-term dependencies, making them more accurate and reliable for making predictions on time series data.
Another advantage of RNNs in time series analysis is their flexibility and adaptability. RNNs can be trained on a variety of different time series data, from financial markets to weather patterns, and can adapt to different patterns and trends in the data. This adaptability makes RNNs a versatile tool for analyzing a wide range of time series data, making them a valuable asset for researchers and analysts in various fields.
In addition to their accuracy and flexibility, RNNs also offer the advantage of being able to handle missing data and irregular time intervals. Traditional statistical methods often struggle with missing data or irregular time intervals, leading to inaccurate predictions. RNNs, however, can handle missing data and irregular time intervals with ease, making them a robust and reliable tool for time series analysis.
Overall, recurrent neural networks are revolutionizing time series analysis by offering a more accurate, flexible, and robust approach to analyzing sequential data. With their ability to capture long-term dependencies, adapt to different patterns, and handle missing data, RNNs are becoming an essential tool for researchers and analysts looking to make predictions based on historical data. As the field of artificial intelligence continues to advance, we can expect to see even more advancements in time series analysis driven by the power of recurrent neural networks.
#Recurrent #Neural #Networks #Revolutionizing #Time #Series #Analysis,rnn
Leave a Reply