Your cart is currently empty!
Introduction to Data Governance for Machine Learning Systems: Fundamental Principles, Critical Practices, and Future Trends
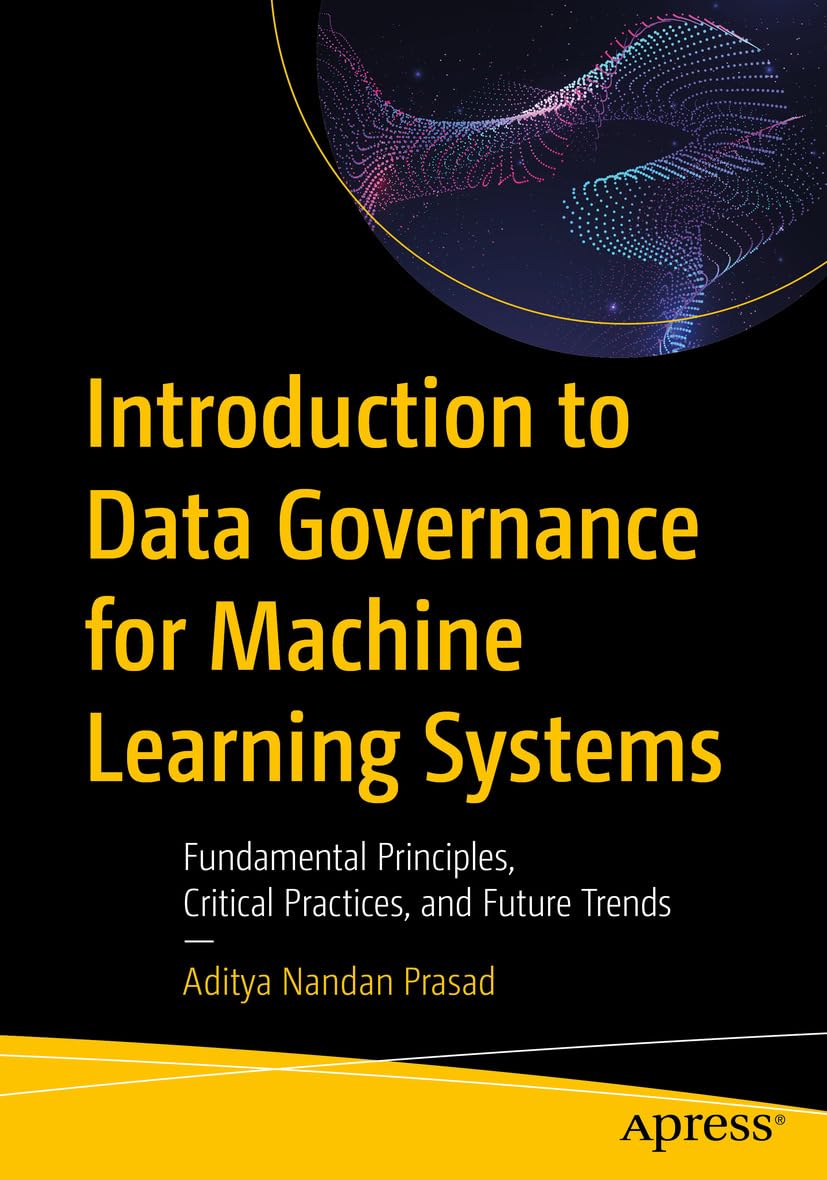
Price: $8.25
(as of Dec 25,2024 00:31:50 UTC – Details)
ASIN : B0DQG95B4F
Publisher : Apress (December 13, 2024)
Publication date : December 13, 2024
Language : English
File size : 1664 KB
Text-to-Speech : Enabled
Screen Reader : Supported
Enhanced typesetting : Enabled
X-Ray : Not Enabled
Word Wise : Not Enabled
Print length : 1317 pages
Data governance is a critical component in ensuring the success and reliability of machine learning systems. In this post, we will explore the fundamental principles, critical practices, and future trends in data governance for machine learning.
Fundamental Principles:
1. Data Quality: Ensuring the accuracy, completeness, and consistency of data is essential for training reliable machine learning models. This involves data profiling, cleansing, and monitoring to maintain high-quality data.
2. Data Privacy and Security: Protecting sensitive data and ensuring compliance with regulations such as GDPR is crucial in machine learning systems. Implementing encryption, access controls, and data masking techniques can help safeguard data privacy.
3. Data Lineage and Traceability: Understanding the origin and transformation of data is important for ensuring transparency and accountability in machine learning models. Maintaining a clear record of data lineage can help identify errors and biases in the model.
Critical Practices:
1. Data Classification and Tagging: Categorizing data based on its sensitivity and importance can help prioritize data governance efforts. Tagging data with metadata can provide valuable insights into data usage and lineage.
2. Data Governance Framework: Establishing a data governance framework that defines roles, responsibilities, and processes for managing data can help ensure consistency and compliance in machine learning systems.
3. Data Monitoring and Auditing: Implementing tools and processes for monitoring data usage, access, and quality can help detect anomalies and ensure data governance policies are being followed.
Future Trends:
1. Automated Data Governance: With the rise of AI and machine learning, automated tools for data governance are becoming increasingly important. These tools can help streamline data governance processes and improve efficiency.
2. Ethical AI: As concerns around bias and fairness in AI continue to grow, ethical considerations will play a larger role in data governance for machine learning systems. Implementing ethical guidelines and standards can help ensure responsible AI deployment.
3. Data Governance as a Service: As organizations continue to adopt cloud-based solutions, data governance as a service is expected to become more prevalent. This can help organizations leverage external expertise and resources to enhance their data governance practices.
In conclusion, data governance is a foundational element in building trustworthy and reliable machine learning systems. By following fundamental principles, implementing critical practices, and staying ahead of future trends, organizations can ensure the success of their machine learning initiatives.
#Introduction #Data #Governance #Machine #Learning #Systems #Fundamental #Principles #Critical #Practices #Future #Trends
Leave a Reply