Your cart is currently empty!
Linking and Mining Heterogeneous and Multi-view Data (Unsupervised and
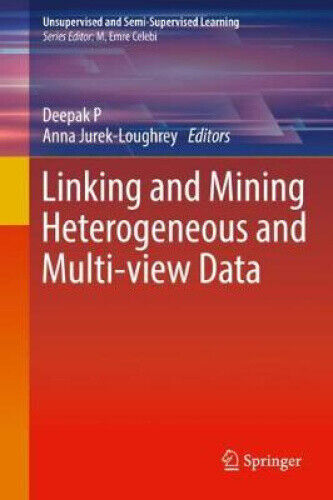
Linking and Mining Heterogeneous and Multi-view Data (Unsupervised and
Price : 139.03
Ends on : N/A
View on eBay
Semi-Supervised Approaches)
Data is becoming increasingly complex and diverse, with information spread across multiple sources and in various formats. Linking and mining heterogeneous and multi-view data has therefore become a crucial task in order to extract valuable insights and knowledge.
In this post, we will explore the challenges and opportunities in linking and mining heterogeneous and multi-view data using both unsupervised and semi-supervised approaches. Unsupervised methods, such as clustering and dimensionality reduction, can help discover hidden patterns and relationships within the data without the need for labeled examples. On the other hand, semi-supervised techniques leverage a small amount of labeled data to guide the learning process and improve the accuracy of the models.
By integrating data from different sources and perspectives, we can gain a more comprehensive understanding of the underlying patterns and structures in the data. This can lead to more accurate predictions, better decision-making, and ultimately, improved performance in various applications such as recommendation systems, fraud detection, and healthcare analytics.
Overall, linking and mining heterogeneous and multi-view data is a challenging yet rewarding task that can provide valuable insights and knowledge. By utilizing unsupervised and semi-supervised approaches, we can effectively extract meaningful information from diverse and complex data sources.
#Linking #Mining #Heterogeneous #Multiview #Data #Unsupervised
Leave a Reply