Your cart is currently empty!
LSTM Networks for Sentiment Analysis: A Game-Changer in Text Classification
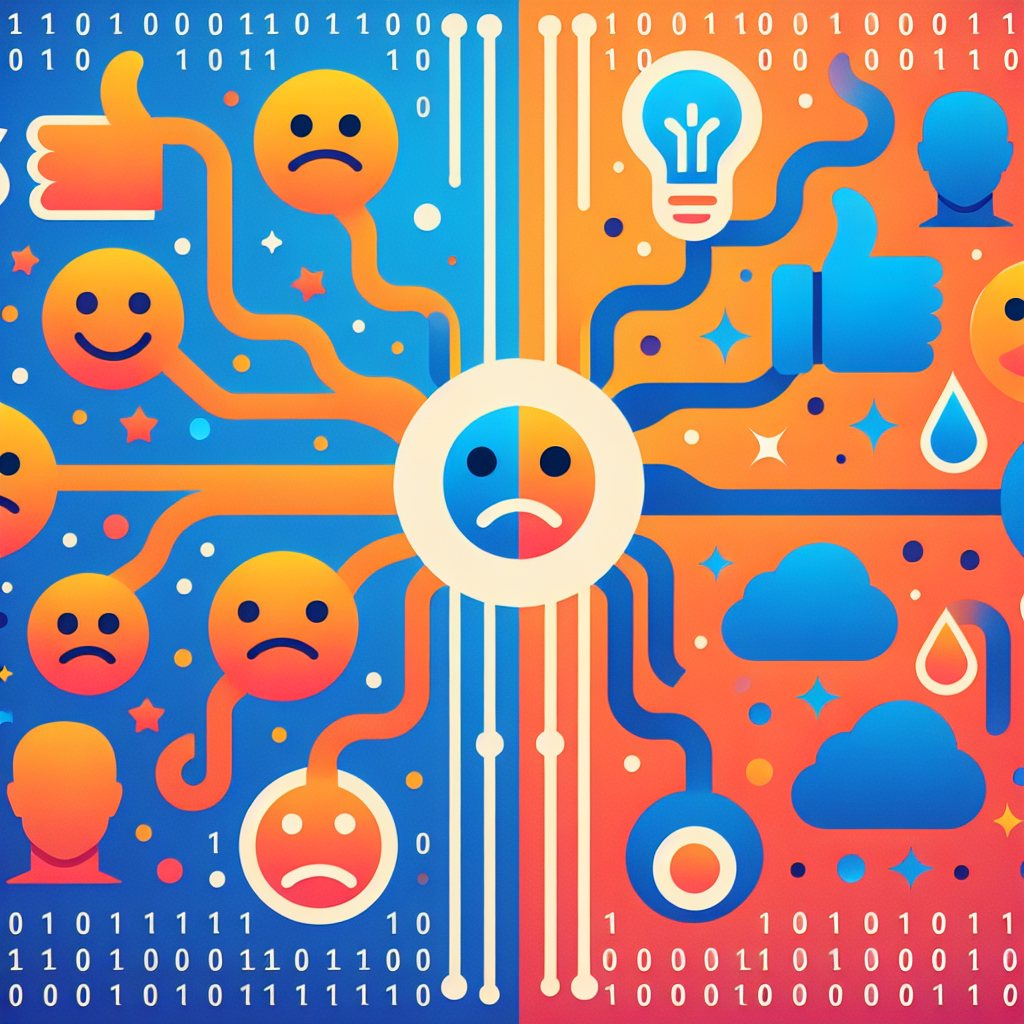
In recent years, the field of natural language processing (NLP) has seen significant advancements in the use of deep learning techniques for text analysis. One such breakthrough is the Long Short-Term Memory (LSTM) network, which has proven to be a game-changer in sentiment analysis and text classification tasks.
LSTMs are a type of recurrent neural network (RNN) that are designed to overcome the limitations of traditional RNNs, which struggle to capture long-range dependencies in sequential data. This makes LSTMs particularly well-suited for tasks like sentiment analysis, where the context of a word or phrase can greatly influence its sentiment.
In sentiment analysis, the goal is to determine the sentiment or opinion expressed in a piece of text, such as a review, tweet, or comment. This is a challenging task, as sentiments can be expressed in a variety of ways and can be influenced by the context in which they are expressed.
LSTMs excel at capturing the sequential nature of text data, allowing them to model complex relationships between words and phrases in a sentence. This enables them to learn patterns in the data that can be used to predict the sentiment of a given piece of text.
One of the key advantages of LSTMs for sentiment analysis is their ability to remember important information from earlier parts of the text, even as new information is being processed. This allows them to capture long-range dependencies in the data and make more accurate predictions about the sentiment of a piece of text.
In addition to their ability to capture long-range dependencies, LSTMs are also highly flexible and can be easily adapted to different types of text classification tasks. This makes them a versatile tool for sentiment analysis across a wide range of applications, from social media monitoring to customer feedback analysis.
Overall, LSTM networks have proven to be a game-changer in sentiment analysis and text classification tasks. Their ability to capture long-range dependencies, flexibility, and adaptability make them a powerful tool for analyzing and understanding text data. As the field of NLP continues to evolve, LSTM networks are likely to play an increasingly important role in sentiment analysis and other text classification tasks.
#LSTM #Networks #Sentiment #Analysis #GameChanger #Text #Classification,lstm
Leave a Reply