Your cart is currently empty!
LSTM Networks in Action: Real-World Use Cases and Success Stories
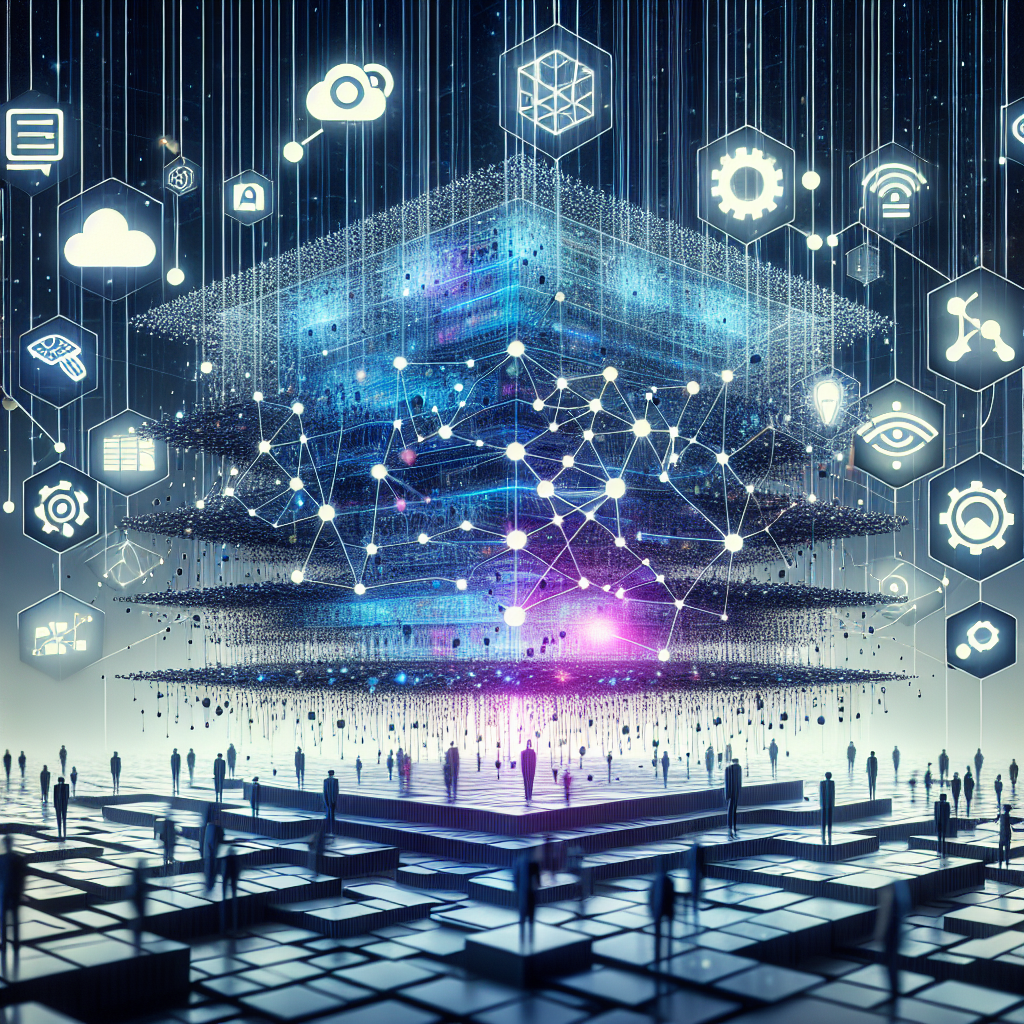
Long Short-Term Memory (LSTM) networks are a type of recurrent neural network (RNN) that is particularly well-suited for dealing with sequences of data. LSTM networks have gained popularity in recent years due to their ability to effectively capture long-term dependencies in sequential data, making them ideal for tasks such as natural language processing, time series prediction, and speech recognition.
In this article, we will explore some real-world use cases and success stories of LSTM networks in action.
1. Natural Language Processing (NLP): One of the most common applications of LSTM networks is in the field of natural language processing. LSTM networks are well-suited for tasks such as language modeling, sentiment analysis, and machine translation. For example, companies like Google and Facebook have used LSTM networks to improve the performance of their machine translation systems, resulting in more accurate and fluent translations.
2. Time Series Prediction: LSTM networks are also widely used for time series prediction tasks, such as forecasting stock prices, weather patterns, and energy consumption. By training an LSTM network on historical time series data, businesses can make more accurate predictions about future trends and make informed decisions based on these forecasts.
3. Speech Recognition: LSTM networks have been successfully applied to speech recognition tasks, where the goal is to convert spoken language into text. By using LSTM networks to model the temporal dependencies in speech signals, companies like Apple and Amazon have been able to develop more accurate and reliable speech recognition systems, enabling users to interact with their devices using voice commands.
4. Anomaly Detection: LSTM networks are also used for anomaly detection in various industries, such as cybersecurity, manufacturing, and healthcare. By training an LSTM network on normal operational data, businesses can detect deviations from the expected behavior and identify potential anomalies or anomalies in real-time, allowing them to take proactive measures to prevent potential issues.
Overall, LSTM networks have proven to be a powerful tool for a wide range of real-world applications, from natural language processing to time series prediction and anomaly detection. By effectively capturing long-term dependencies in sequential data, LSTM networks are enabling businesses to make more accurate predictions, improve decision-making, and enhance the overall performance of their systems. As technology continues to advance, we can expect to see even more innovative use cases and success stories of LSTM networks in action.
#LSTM #Networks #Action #RealWorld #Cases #Success #Stories,lstm
Leave a Reply