Your cart is currently empty!
LSTM Networks: The Key to Unlocking Time Series Data
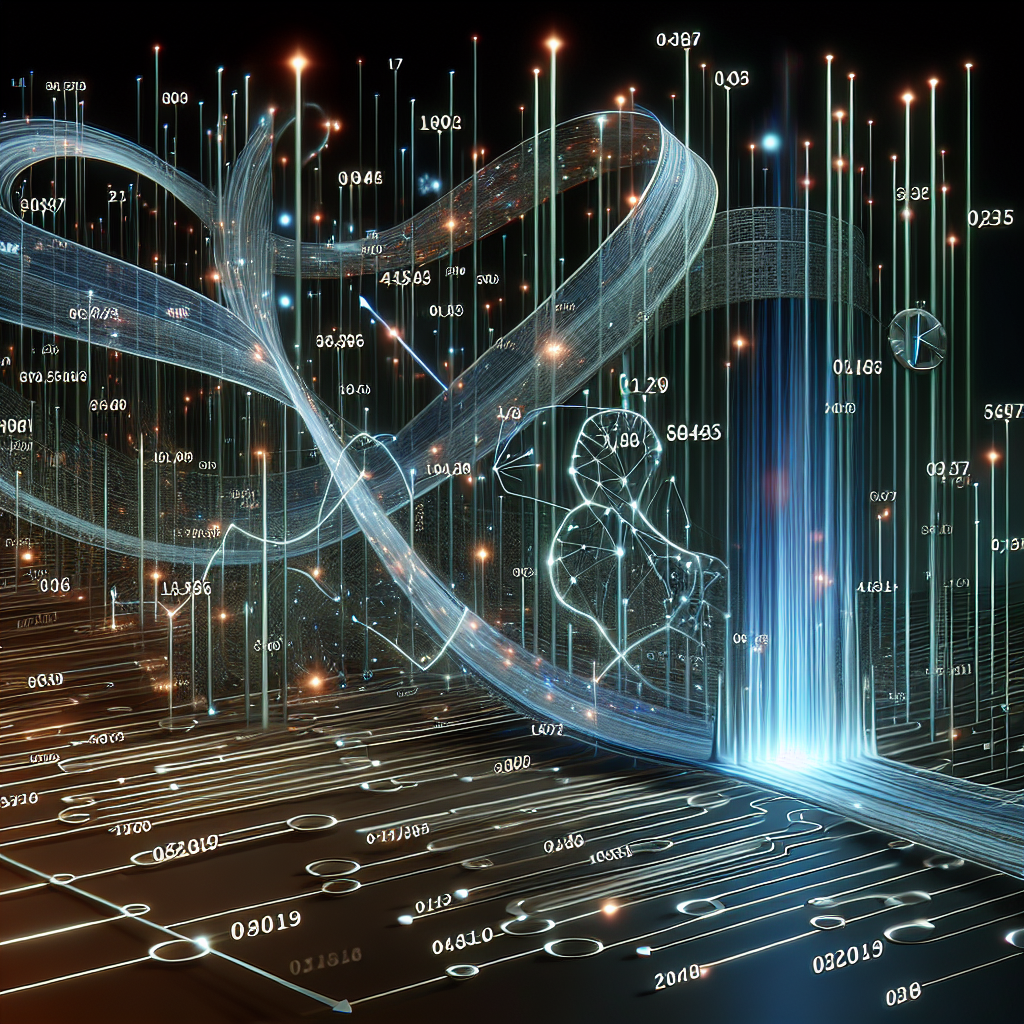
In recent years, the use of LSTM (Long Short-Term Memory) networks has gained popularity in the field of machine learning, particularly in the analysis of time series data. LSTM networks are a type of recurrent neural network (RNN) that are capable of learning long-term dependencies in data, making them well-suited for tasks such as time series forecasting, speech recognition, and natural language processing.
One of the key advantages of LSTM networks is their ability to retain information over long periods of time. Traditional RNNs can struggle with this task due to the vanishing gradient problem, where gradients become increasingly small as they are propagated back through time. LSTM networks address this issue by introducing a set of gating mechanisms that control the flow of information within the network, allowing it to remember important information while discarding irrelevant details.
This ability to capture long-term dependencies makes LSTM networks particularly well-suited for analyzing time series data, which often exhibit complex patterns and trends that evolve over time. By incorporating LSTM networks into their models, researchers and practitioners have been able to achieve significant improvements in accuracy and performance across a wide range of time series forecasting tasks.
One common application of LSTM networks in time series analysis is in the prediction of stock prices. By feeding historical price data into an LSTM network, researchers can train the model to learn patterns and trends in the data, allowing it to make accurate predictions about future price movements. This can be incredibly valuable for investors and traders looking to make informed decisions about their portfolios.
Another area where LSTM networks excel is in the analysis of sensor data, such as temperature readings or accelerometer measurements. By leveraging the network’s ability to capture long-term dependencies, researchers can extract valuable insights from these data streams, such as identifying anomalies or predicting future trends.
In conclusion, LSTM networks are a powerful tool for unlocking the potential of time series data. By leveraging their ability to capture long-term dependencies, researchers and practitioners can gain valuable insights and make more accurate predictions in a wide range of applications. As the field of machine learning continues to advance, LSTM networks are sure to play a key role in shaping the future of time series analysis.
#LSTM #Networks #Key #Unlocking #Time #Series #Data,lstm
Leave a Reply