Your cart is currently empty!
Machine Learning for Engineers: Introduction to Physics-Informed, Explainable Learning Methods for AI in Engineering Applications
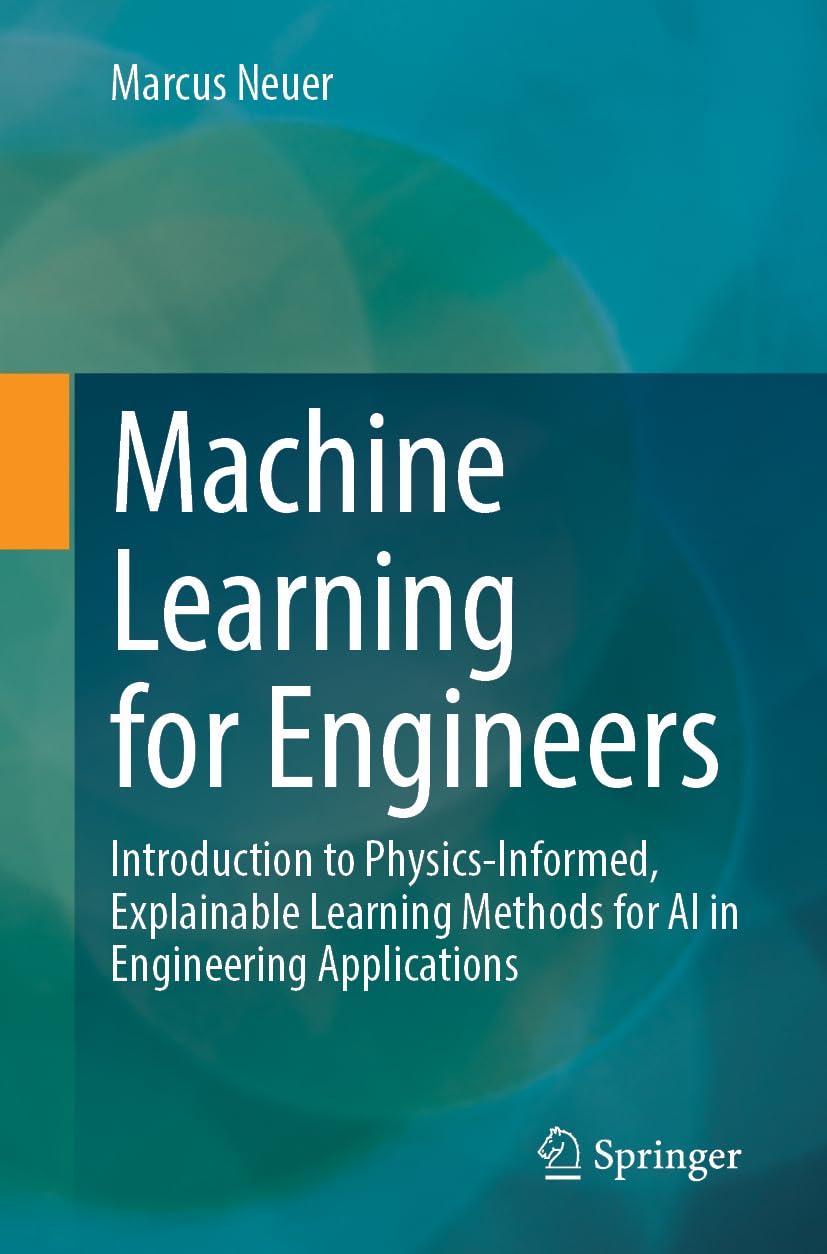
Price: $54.99
(as of Nov 29,2024 07:49:44 UTC – Details)
Publisher : Springer; 2024th edition (December 31, 2024)
Language : English
Paperback : 258 pages
ISBN-10 : 366269994X
ISBN-13 : 978-3662699942
Item Weight : 1.11 pounds
Machine Learning for Engineers: Introduction to Physics-Informed, Explainable Learning Methods for AI in Engineering Applications
In recent years, machine learning has become an indispensable tool for engineers in various fields, including aerospace, automotive, civil, and mechanical engineering. With the advancement of AI technologies, engineers are now able to leverage machine learning algorithms to solve complex engineering problems more efficiently and accurately.
One of the emerging trends in machine learning for engineering applications is the use of physics-informed and explainable learning methods. These methods combine the power of traditional machine learning algorithms with domain-specific knowledge of physics and engineering principles to improve the performance and interpretability of AI models.
Physics-informed learning methods aim to incorporate known physical laws and constraints into the machine learning models, making them more robust and reliable in predicting real-world phenomena. By integrating physics-based constraints into the training process, engineers can ensure that the AI models are not only accurate but also physically meaningful and interpretable.
Explainable learning methods, on the other hand, focus on providing insights into how the AI models make predictions and decisions. These methods aim to enhance the transparency and trustworthiness of machine learning models by enabling engineers to understand the underlying logic and reasoning behind the predictions. This is crucial in engineering applications where the stakes are high, and decisions based on AI models can have significant implications.
Overall, physics-informed and explainable learning methods offer a promising approach to harness the power of AI in engineering applications while ensuring the reliability, interpretability, and trustworthiness of the models. As engineers continue to explore the potential of machine learning in their field, incorporating these advanced techniques will be essential for pushing the boundaries of what is possible and driving innovation in engineering.
#Machine #Learning #Engineers #Introduction #PhysicsInformed #Explainable #Learning #Methods #Engineering #Applications
Leave a Reply