Your cart is currently empty!
Machine Learning from Weak Supervision: An Empirical Risk Minimization Approach
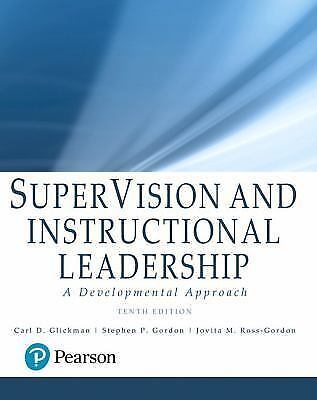
Machine Learning from Weak Supervision: An Empirical Risk Minimization Approach
Price : 32.97
Ends on : N/A
View on eBay
Machine Learning from Weak Supervision: An Empirical Risk Minimization Approach
In the field of machine learning, the traditional approach to training models involves providing them with labeled data that accurately reflects the true underlying distribution of the data. However, in many real-world scenarios, obtaining labeled data can be expensive, time-consuming, or simply impractical.
This is where weak supervision comes in. Weak supervision refers to the use of noisy, incomplete, or imprecise labels to train machine learning models. While weak supervision can introduce challenges such as increased noise and uncertainty in the training data, it also offers the potential to scale up machine learning applications by reducing the need for manually labeled data.
One approach to learning from weak supervision is through Empirical Risk Minimization (ERM). ERM is a fundamental principle in machine learning that aims to minimize the expected loss of a model on a training dataset. By leveraging weak supervision and ERM, researchers and practitioners can train models on large-scale, noisy datasets and still achieve good generalization performance.
In recent years, there has been a growing interest in developing algorithms and techniques that can effectively leverage weak supervision for training machine learning models. These approaches include methods for handling noisy labels, learning from multiple weak supervision sources, and incorporating domain knowledge to improve model performance.
Overall, the use of weak supervision and ERM in machine learning represents an exciting area of research with the potential to revolutionize how models are trained and deployed in real-world applications. By embracing the challenges and opportunities that weak supervision presents, researchers and practitioners can unlock new possibilities for building more robust and scalable machine learning systems.
#Machine #Learning #Weak #Supervision #Empirical #Risk #Minimization #Approach
Leave a Reply