Your cart is currently empty!
Machine Learning Security with Azure: Best practices for assessing, securing,…
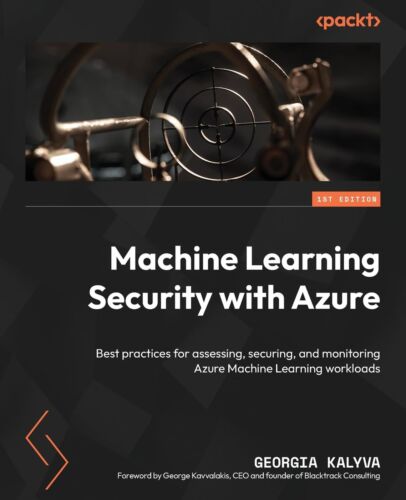
Machine Learning Security with Azure: Best practices for assessing, securing,…
Price : 43.00
Ends on : N/A
View on eBay
In today’s digital world, machine learning is playing an increasingly important role in various industries, from healthcare to finance to manufacturing. While machine learning offers countless benefits, it also brings new security challenges that must be addressed to protect sensitive data and ensure the integrity of machine learning models.
Azure, Microsoft’s cloud platform, offers a range of tools and services to help organizations secure their machine learning workflows. In this post, we’ll explore some best practices for assessing and securing machine learning models in Azure.
1. Data protection: One of the key considerations in securing machine learning models is protecting the data used to train and test the models. Azure offers a range of data protection tools, including encryption, access control, and data masking, to help organizations protect their data from unauthorized access.
2. Model validation: Before deploying a machine learning model in a production environment, it’s essential to validate the model to ensure it performs as expected and is not vulnerable to attacks. Azure provides tools for model validation, such as model explainability and fairness assessments, to help organizations identify and address potential security risks.
3. Secure deployment: Once a machine learning model has been validated, it’s important to deploy it securely in a production environment. Azure offers tools for secure model deployment, such as containerization, authentication, and authorization, to help organizations protect their models from unauthorized access and tampering.
4. Monitoring and detection: Even after a machine learning model has been deployed, it’s crucial to continuously monitor and detect any security threats or anomalies. Azure provides tools for monitoring and detection, such as anomaly detection and threat intelligence, to help organizations identify and respond to security incidents in real-time.
By following these best practices for assessing, securing, and monitoring machine learning models in Azure, organizations can effectively protect their data and ensure the integrity of their machine learning workflows. With Azure’s robust security tools and services, organizations can confidently leverage machine learning to drive innovation and achieve their business goals.
#Machine #Learning #Security #Azure #practices #assessing #securing.., Intelligent data infrastructure
Leave a Reply