Your cart is currently empty!
Mastering Generative AI and Data Engineering: Techniques, Tools, and Practical A
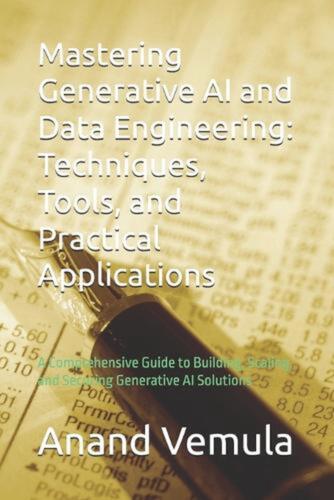
Mastering Generative AI and Data Engineering: Techniques, Tools, and Practical A
Price : 16.16
Ends on : N/A
View on eBay
pplications
In today’s data-driven world, mastering generative artificial intelligence and data engineering is becoming increasingly important for businesses looking to gain a competitive edge. From creating personalized recommendations for customers to optimizing supply chain operations, the possibilities are endless with the right tools and techniques.
In this post, we will explore some of the key concepts and tools used in generative AI and data engineering, as well as practical applications of these technologies in real-world scenarios.
1. Understanding Generative AI: Generative AI refers to algorithms and models that are capable of creating new data based on patterns and examples from existing datasets. This can be used for tasks such as generating realistic images, text, or even music. Some popular generative AI techniques include generative adversarial networks (GANs), variational autoencoders (VAEs), and transformer models.
2. Data Engineering Basics: Data engineering involves the process of collecting, storing, and processing data in a way that is efficient and scalable. This often involves working with large datasets, building data pipelines, and ensuring data quality and reliability. Tools such as Apache Spark, Hadoop, and Kafka are commonly used in data engineering projects.
3. Tools for Generative AI and Data Engineering: There are a variety of tools and frameworks available for working with generative AI and data engineering. For generative AI, popular frameworks include TensorFlow, PyTorch, and OpenAI’s GPT models. In data engineering, tools such as Apache Airflow, Databricks, and AWS Glue are commonly used for managing data pipelines and workflows.
4. Practical Applications: Generative AI and data engineering can be applied to a wide range of industries and use cases. For example, in e-commerce, generative AI can be used to generate product recommendations based on customer preferences. In healthcare, data engineering can be used to analyze patient data and improve outcomes. The possibilities are endless, and mastering these technologies can unlock new opportunities for businesses.
In conclusion, mastering generative AI and data engineering is essential for businesses looking to harness the power of data and AI. By understanding the key concepts, tools, and practical applications of these technologies, businesses can stay ahead of the competition and drive innovation in today’s data-driven world.
#Mastering #Generative #Data #Engineering #Techniques #Tools #Practical
Leave a Reply