Your cart is currently empty!
Maximizing the Potential of Foundation Models in AI Engineering Building Applications
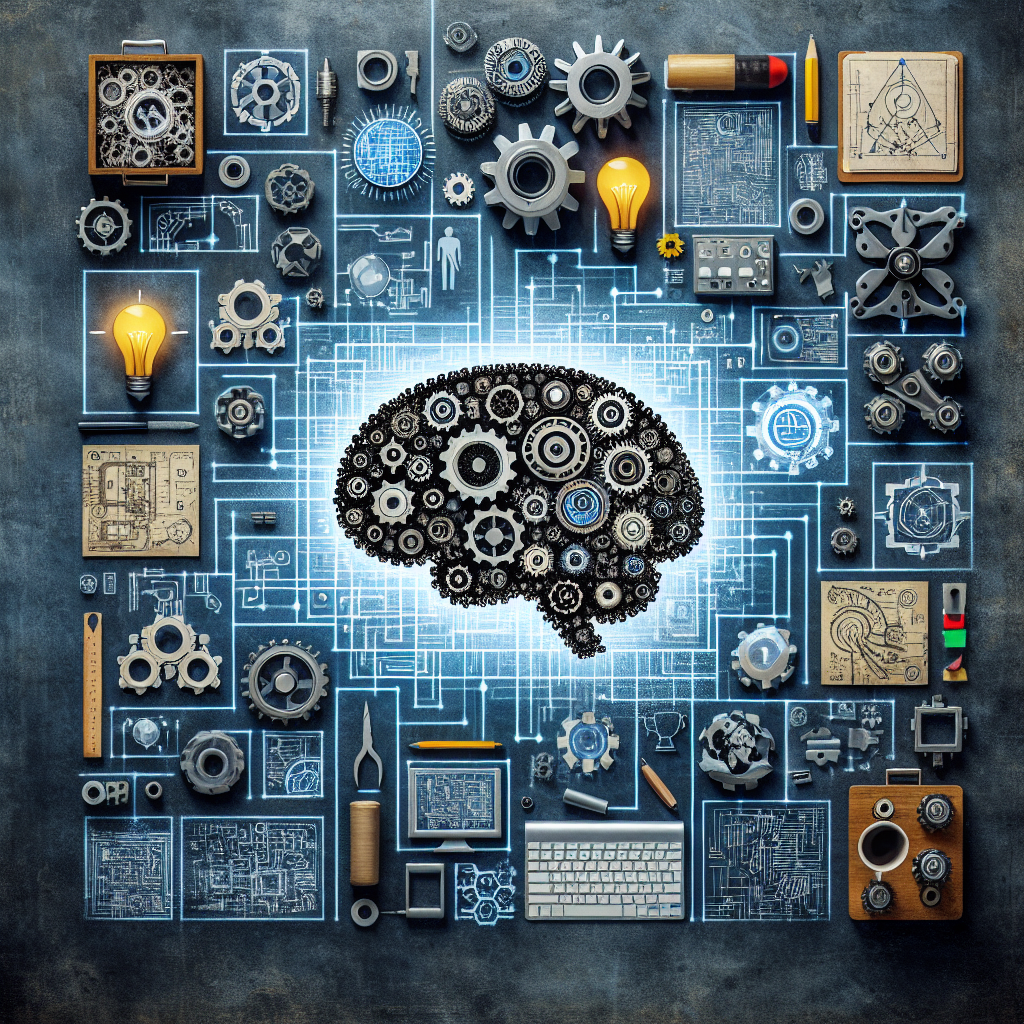
Foundation models are the backbone of artificial intelligence engineering, serving as the building blocks for a wide range of applications. These models, such as OpenAI’s GPT-3 or Google’s BERT, are pre-trained on vast amounts of data and can be fine-tuned for specific tasks, making them incredibly versatile and powerful tools in the field of AI.
To maximize the potential of foundation models in AI engineering building applications, it is essential to understand how they work and how they can be effectively utilized. Here are some key strategies for harnessing the power of foundation models in your AI projects:
1. Fine-tuning for specific tasks: One of the key advantages of foundation models is their ability to be fine-tuned for specific tasks. By training the model on a smaller dataset that is relevant to the task at hand, you can tailor it to perform well on that particular task. This can significantly improve the model’s performance and accuracy, making it more effective in real-world applications.
2. Transfer learning: Transfer learning is another powerful technique for maximizing the potential of foundation models. By starting with a pre-trained model and then fine-tuning it on a new dataset, you can leverage the knowledge and skills already present in the model to quickly adapt it to new tasks. This can save time and resources, allowing you to build more sophisticated AI applications more efficiently.
3. Data augmentation: Data augmentation is a technique that involves artificially increasing the size of your training dataset by applying transformations to the existing data. This can help improve the generalization and robustness of your model, making it more effective in real-world scenarios. By augmenting your data before fine-tuning your foundation model, you can enhance its performance and make it more versatile in different applications.
4. Hyperparameter tuning: Hyperparameters are parameters that are set before the training process begins and can significantly impact the performance of your model. By carefully tuning these hyperparameters, you can optimize the performance of your foundation model and improve its accuracy on specific tasks. Experimenting with different hyperparameter values and techniques can help you find the optimal configuration for your model, maximizing its potential in AI engineering building applications.
5. Monitoring and evaluation: Finally, it is crucial to continuously monitor and evaluate the performance of your foundation model in real-world applications. By tracking key metrics and analyzing the model’s behavior, you can identify areas for improvement and make necessary adjustments to enhance its performance. Regularly testing and evaluating your model in different scenarios can help you optimize its performance and ensure that it continues to meet the needs of your AI applications.
In conclusion, foundation models are powerful tools in AI engineering building applications, offering a versatile and efficient way to leverage the power of artificial intelligence. By employing strategies such as fine-tuning, transfer learning, data augmentation, hyperparameter tuning, and monitoring and evaluation, you can maximize the potential of foundation models in your AI projects and build more effective and sophisticated applications. With the right approach and techniques, you can unlock the full potential of foundation models and take your AI engineering projects to the next level.
#Maximizing #Potential #Foundation #Models #Engineering #Building #Applications,ai engineering building applications with foundation models
Leave a Reply