Your cart is currently empty!
Modern Deep Learning for Tabular Data: Novel Approaches to Common Modeling
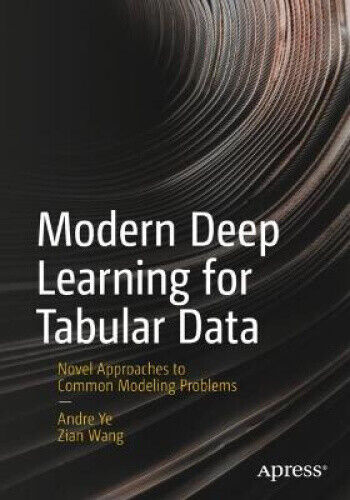
Modern Deep Learning for Tabular Data: Novel Approaches to Common Modeling
Price : 61.98
Ends on : N/A
View on eBay
Deep learning has revolutionized the field of machine learning, offering powerful tools for modeling complex data. While deep learning has traditionally been applied to tasks such as image recognition and natural language processing, recent advancements have shown that it can also be highly effective for tabular data.
In this post, we will explore some novel approaches to applying deep learning to tabular data, focusing on techniques that can improve model performance and generalization.
One approach that has gained traction in recent years is the use of neural networks with attention mechanisms for tabular data. Attention mechanisms allow the model to focus on certain parts of the input data that are most relevant for making predictions, leading to improved performance and interpretability. By incorporating attention mechanisms into neural networks, researchers have been able to achieve state-of-the-art results on a variety of tabular datasets.
Another promising approach is the use of graph neural networks for modeling tabular data. Graph neural networks are well-suited for capturing complex relationships and dependencies between different features in a dataset, making them particularly effective for tasks such as fraud detection or recommender systems. By representing tabular data as a graph and applying graph neural networks, researchers have been able to achieve significant improvements in model performance.
Finally, transfer learning has also emerged as a powerful technique for leveraging pre-trained deep learning models for tabular data. By fine-tuning a pre-trained model on a new dataset, researchers can quickly adapt the model to new tasks and achieve high performance with limited data. This approach has been particularly effective for tasks such as time series forecasting and anomaly detection.
In conclusion, modern deep learning techniques offer exciting opportunities for modeling tabular data. By incorporating attention mechanisms, graph neural networks, and transfer learning, researchers can achieve state-of-the-art results on a variety of tasks. These novel approaches not only improve model performance but also provide valuable insights into the underlying relationships within the data. As deep learning continues to evolve, we can expect to see even more innovative approaches for modeling tabular data in the future.
#Modern #Deep #Learning #Tabular #Data #Approaches #Common #Modeling
Leave a Reply