Your cart is currently empty!
Optimizing Data Center Storage for AI and Machine Learning Workloads
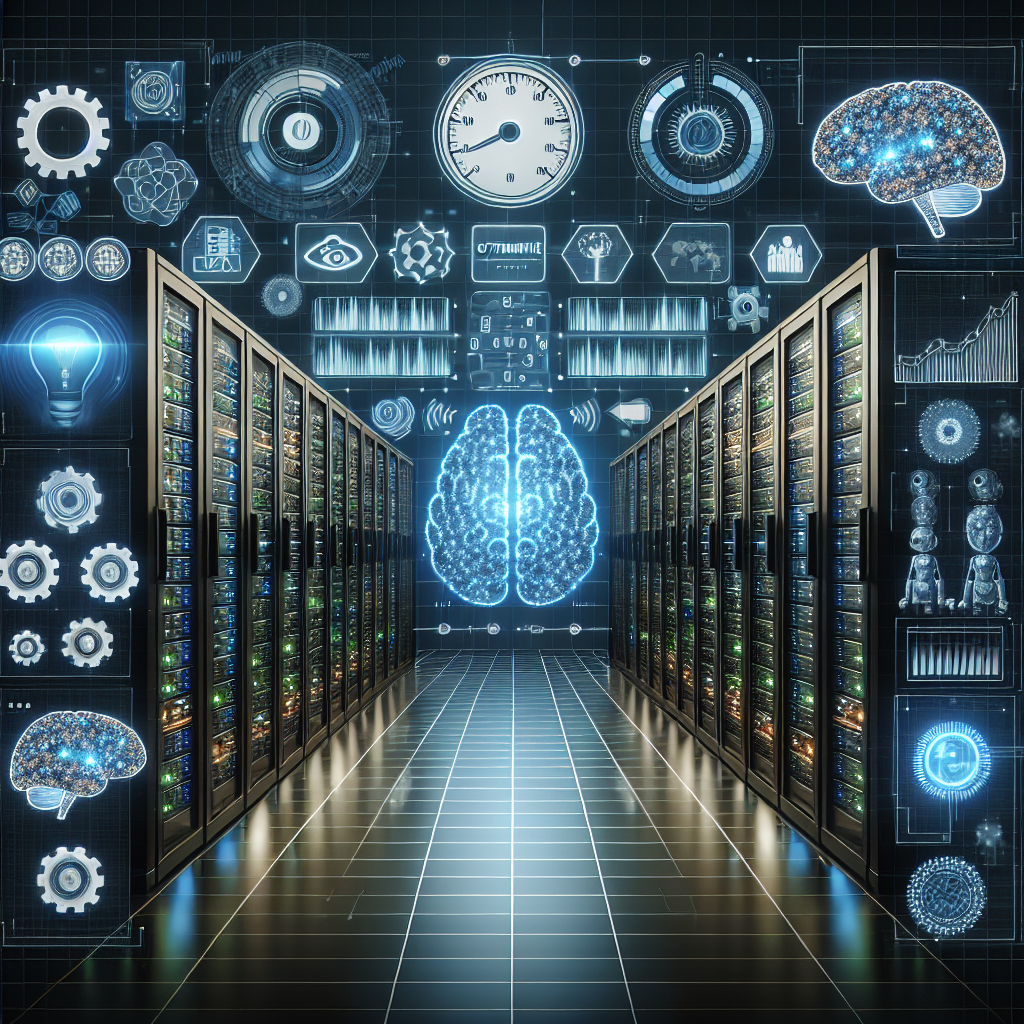
With the increasing adoption of artificial intelligence (AI) and machine learning (ML) technologies across various industries, data centers are facing new challenges in optimizing storage solutions to support these demanding workloads. AI and ML applications require large amounts of data to be processed quickly and efficiently, making data center storage a critical component in ensuring smooth and reliable operations.
To optimize data center storage for AI and ML workloads, organizations can consider the following strategies:
1. Utilize high-performance storage technologies: Traditional storage solutions may not be able to keep up with the performance requirements of AI and ML workloads. Organizations should consider investing in high-performance storage technologies such as solid-state drives (SSDs) or non-volatile memory express (NVMe) storage to ensure fast data access and processing speeds.
2. Implement scalable storage solutions: AI and ML workloads often involve processing large datasets, which can quickly outgrow storage capacities. Organizations should implement scalable storage solutions that can easily expand to accommodate growing data requirements. This can include technologies such as storage area networks (SAN) or network-attached storage (NAS) systems.
3. Optimize data placement and tiering: Data center administrators can optimize storage for AI and ML workloads by strategically placing data based on access patterns and performance requirements. By implementing storage tiering, organizations can move frequently accessed data to high-performance storage tiers, while less frequently accessed data can be stored on lower-cost, high-capacity storage tiers.
4. Implement data compression and deduplication: Data compression and deduplication techniques can help organizations reduce storage costs and improve data processing speeds for AI and ML workloads. By eliminating redundant data and compressing data before storage, organizations can maximize storage efficiency and reduce the amount of data that needs to be processed.
5. Leverage cloud storage services: Organizations can also consider leveraging cloud storage services for AI and ML workloads. Cloud storage offers scalability, flexibility, and cost-effectiveness, allowing organizations to easily scale storage resources based on workload requirements. Additionally, cloud storage providers often offer advanced data management and analytics tools that can help organizations optimize storage for AI and ML workloads.
In conclusion, optimizing data center storage for AI and ML workloads is crucial for ensuring smooth and efficient operations. By investing in high-performance storage technologies, implementing scalable storage solutions, optimizing data placement and tiering, implementing data compression and deduplication, and leveraging cloud storage services, organizations can effectively support the demanding storage requirements of AI and ML workloads. By implementing these strategies, organizations can ensure that their data center storage infrastructure is well-equipped to handle the challenges of AI and ML workloads and drive innovation and growth in their organizations.
Leave a Reply