Your cart is currently empty!
Optimizing Data Center Storage for Big Data Analytics
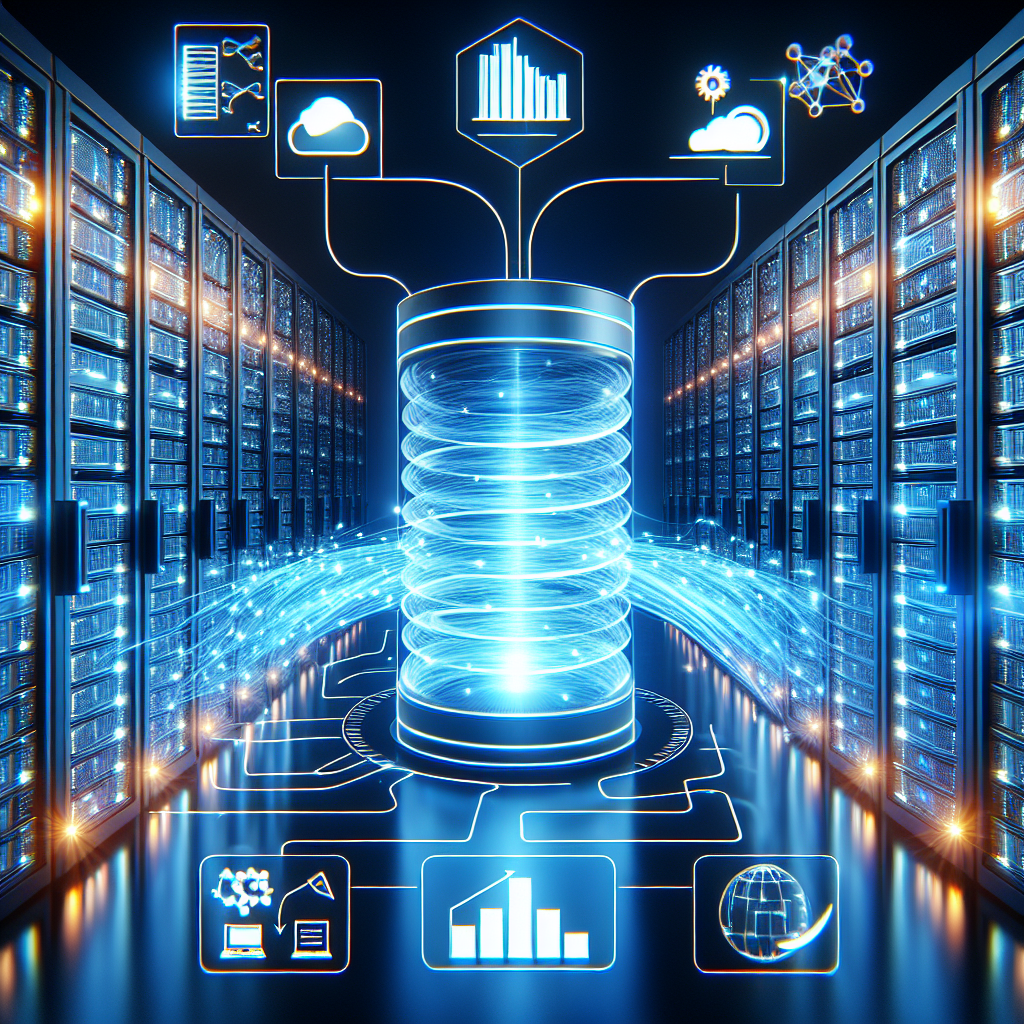
In today’s digital age, the amount of data being generated and collected is skyrocketing. From social media interactions to online transactions, businesses are inundated with massive amounts of data that can provide valuable insights and drive decision-making. To effectively harness this data and derive meaningful insights, organizations are turning to big data analytics.
Big data analytics involves processing and analyzing large sets of data to uncover patterns, trends, and correlations that can help businesses make informed decisions and improve operations. However, the success of big data analytics relies heavily on the storage and management of data within a data center.
Optimizing data center storage for big data analytics is crucial to ensure that data is easily accessible, reliable, and secure. Here are some key strategies to optimize data center storage for big data analytics:
1. Scale-out storage architecture: Traditional storage architectures may not be able to handle the massive amounts of data generated by big data analytics. Scale-out storage architectures allow organizations to easily expand storage capacity by adding more storage nodes, ensuring that performance and capacity can grow with the demands of big data analytics.
2. High-performance storage devices: Big data analytics require high-speed access to data to ensure timely insights. Utilizing high-performance storage devices such as solid-state drives (SSDs) or NVMe drives can significantly improve data processing speeds and reduce latency.
3. Data tiering: Not all data generated by big data analytics is equally important. Implementing data tiering strategies can help organizations prioritize and store critical data on high-performance storage devices, while less critical data can be stored on lower-cost, slower storage devices.
4. Data compression and deduplication: Big data analytics can generate huge amounts of redundant data. Implementing data compression and deduplication techniques can help organizations reduce storage costs and optimize storage capacity by eliminating duplicate data.
5. Data protection and security: Data security is paramount in big data analytics, especially when dealing with sensitive or confidential information. Implementing robust data protection measures such as encryption and access controls can help organizations safeguard their data and ensure compliance with data privacy regulations.
6. Monitoring and analytics: Monitoring storage performance and capacity is essential to ensure optimal performance for big data analytics. Utilizing storage monitoring tools and analytics can help organizations identify bottlenecks, optimize storage utilization, and proactively address storage issues before they impact data analytics operations.
In conclusion, optimizing data center storage for big data analytics is essential for organizations looking to leverage data-driven insights to drive business success. By implementing scalable storage architectures, high-performance storage devices, data tiering strategies, data compression and deduplication techniques, data protection measures, and storage monitoring tools, organizations can ensure that their data center storage infrastructure is capable of supporting the demands of big data analytics. Ultimately, a well-optimized data center storage environment can help organizations unlock the full potential of their data and gain a competitive edge in today’s data-driven marketplace.
Leave a Reply