Your cart is currently empty!
Panorama of Deep Learning Based Recommender System: Development & Challenges by
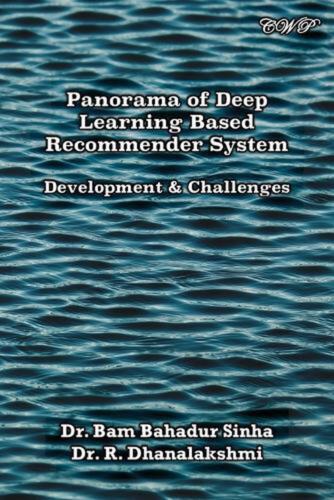
Panorama of Deep Learning Based Recommender System: Development & Challenges by
Price : 85.84
Ends on : N/A
View on eBay
Panorama of Deep Learning Based Recommender System: Development & Challenges
Deep learning has revolutionized the way recommender systems work, enabling them to provide more accurate and personalized recommendations to users. In this post, we will explore the development of deep learning-based recommender systems and the challenges they face.
Development of Deep Learning Based Recommender Systems:
Deep learning-based recommender systems have gained popularity due to their ability to extract complex patterns from large amounts of data, leading to more accurate recommendations. These systems use neural networks to learn from user preferences and item characteristics, allowing them to make personalized recommendations.
One of the key advantages of deep learning-based recommender systems is their ability to handle different types of data, such as structured and unstructured data. This enables them to incorporate various features, such as user demographics, item attributes, and user-item interactions, to improve recommendation accuracy.
Challenges of Deep Learning Based Recommender Systems:
Despite their many advantages, deep learning-based recommender systems face several challenges. One of the main challenges is the need for large amounts of data to train the models effectively. Deep learning models require a substantial amount of data to learn complex patterns, which can be a limiting factor for smaller companies with less data.
Another challenge is the interpretability of deep learning models. Deep learning models are often seen as black boxes, making it difficult to understand how they arrive at their recommendations. This lack of transparency can be a concern for users who want to know why a particular recommendation was made.
Additionally, deep learning-based recommender systems may suffer from issues such as overfitting, cold start problems, and scalability issues. Overfitting occurs when a model learns the noise in the training data, leading to poor generalization on new data. Cold start problems occur when the system cannot make recommendations for new users or items with little to no interaction data. Scalability can be a challenge for deep learning models as they require significant computational resources to train and deploy.
In conclusion, deep learning-based recommender systems have made significant advancements in providing personalized recommendations to users. However, they are not without challenges, including the need for large amounts of data, interpretability issues, and scalability concerns. Addressing these challenges will be crucial for the continued development and adoption of deep learning-based recommender systems.
#Panorama #Deep #Learning #Based #Recommender #System #Development #Challenges, deep learning
Leave a Reply