Your cart is currently empty!
Practical Deep Learning at Scale with MLflow: Bridge the gap between offline experimentation and online production
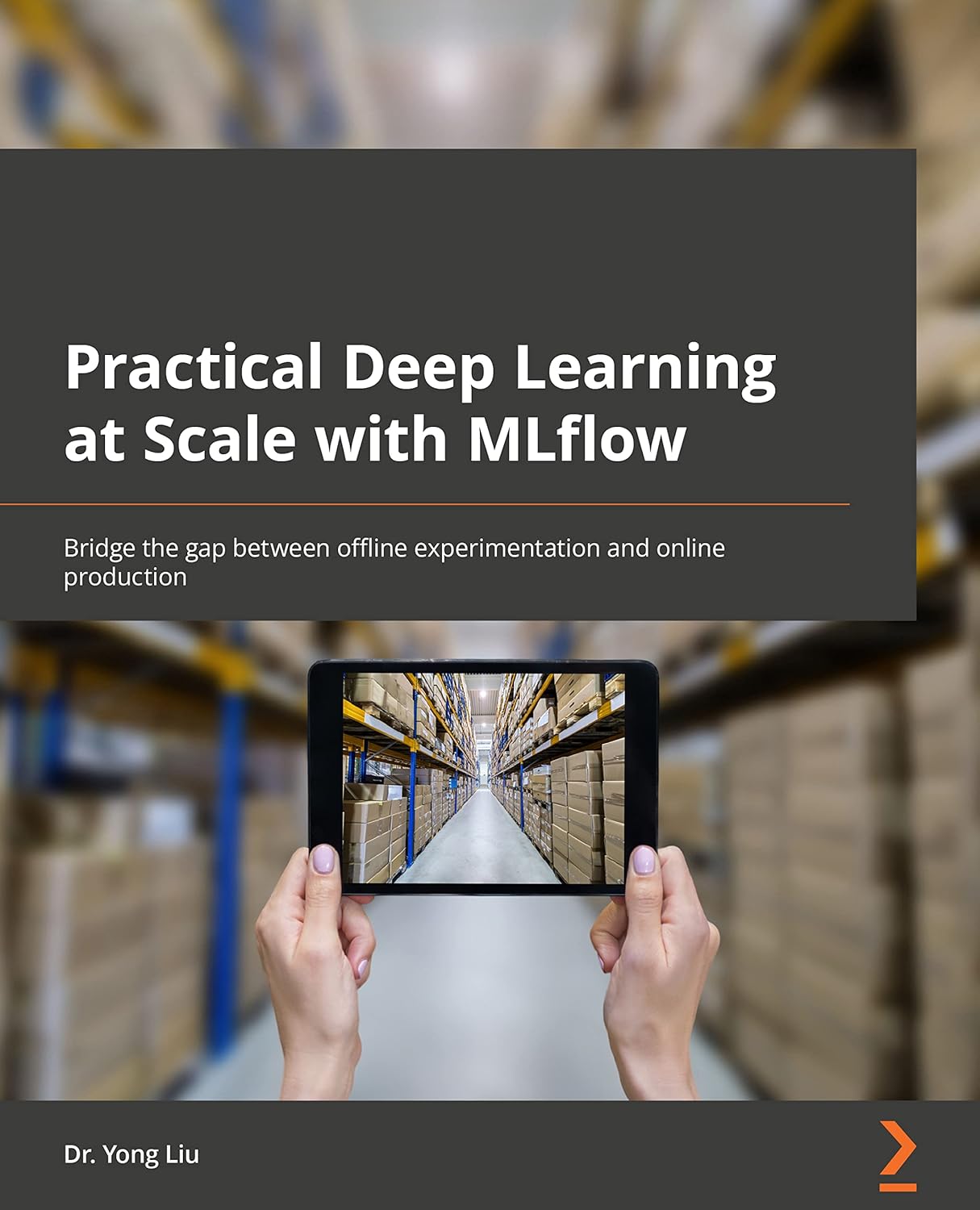
Price: $45.09
(as of Dec 24,2024 22:32:46 UTC – Details)
Publisher : Packt Publishing (July 8, 2022)
Language : English
Paperback : 288 pages
ISBN-10 : 1803241330
ISBN-13 : 978-1803241333
Item Weight : 1.12 pounds
Dimensions : 9.25 x 7.52 x 0.61 inches
Deep learning has revolutionized the field of artificial intelligence, enabling remarkable advancements in areas such as image recognition, natural language processing, and speech recognition. However, deploying deep learning models at scale in production environments remains a significant challenge for many organizations.
MLflow, an open-source platform for managing the end-to-end machine learning lifecycle, provides a practical solution for bridging the gap between offline experimentation and online production. By enabling data scientists to track experiments, package code and dependencies, and deploy models in a reproducible and scalable manner, MLflow empowers organizations to streamline their machine learning workflows and accelerate the deployment of deep learning models.
In this post, we will explore how MLflow can be used to facilitate practical deep learning at scale. We will discuss key features of MLflow, such as tracking experiments, managing models, and deploying models to production. Additionally, we will provide real-world examples of how organizations have successfully implemented MLflow to improve their deep learning workflows.
By leveraging MLflow, organizations can effectively manage their machine learning pipelines, reduce time-to-market for new models, and ensure reproducibility and scalability in their deep learning deployments. With MLflow, the gap between offline experimentation and online production can be bridged, enabling organizations to realize the full potential of deep learning at scale.
#Practical #Deep #Learning #Scale #MLflow #Bridge #gap #offline #experimentation #online #production
Leave a Reply