Your cart is currently empty!
Predictive Analytics with Microsoft Azure Machine Learning: Build and Deploy Ac
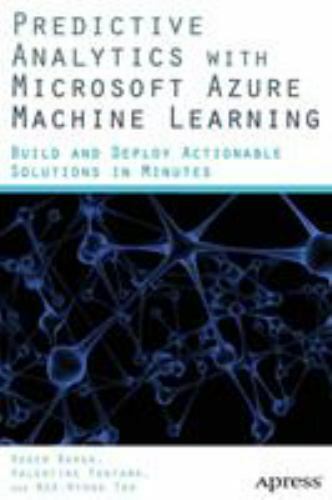
Predictive Analytics with Microsoft Azure Machine Learning: Build and Deploy Ac
Price : 12.11
Ends on : N/A
View on eBay
Predictive Analytics with Microsoft Azure Machine Learning: Build and Deploy Accurate Models
In today’s data-driven world, businesses are constantly seeking ways to gain insights and make informed decisions based on data. Predictive analytics is a powerful tool that enables organizations to forecast future trends, identify patterns, and make data-driven predictions.
Microsoft Azure Machine Learning is a cloud-based platform that allows users to build, train, and deploy machine learning models. With built-in tools and capabilities, Azure Machine Learning makes it easy for data scientists and analysts to create accurate predictive models.
In this post, we will explore how you can leverage Azure Machine Learning to build and deploy accurate predictive analytics models. By following these steps, you can harness the power of machine learning to make informed decisions and drive business growth.
Step 1: Data Preparation
Before you can build a predictive model, you need to prepare your data. This involves cleaning and formatting the data, handling missing values, and selecting relevant features. Azure Machine Learning provides tools to help you streamline this process and ensure your data is ready for analysis.
Step 2: Model Building
Once your data is prepared, you can start building your predictive model. Azure Machine Learning offers a variety of algorithms and techniques to help you train your model on historical data and generate predictions. You can experiment with different algorithms and parameters to find the best model for your specific use case.
Step 3: Model Evaluation
After training your model, it’s important to evaluate its performance to ensure accuracy and reliability. Azure Machine Learning provides tools to help you evaluate your model’s performance, such as metrics like accuracy, precision, recall, and F1 score. By analyzing these metrics, you can fine-tune your model and improve its predictive capabilities.
Step 4: Model Deployment
Once you have a well-performing predictive model, you can deploy it to make predictions on new data. Azure Machine Learning offers a seamless deployment process, allowing you to easily integrate your model into your existing applications and workflows. This enables you to leverage the power of predictive analytics in real-time and drive actionable insights.
By following these steps and leveraging the capabilities of Microsoft Azure Machine Learning, you can build and deploy accurate predictive analytics models to make informed decisions and drive business growth. Whether you’re forecasting sales, predicting customer behavior, or optimizing operations, predictive analytics can help you unlock valuable insights and stay ahead of the competition.
#Predictive #Analytics #Microsoft #Azure #Machine #Learning #Build #Deploy, machine learning
Leave a Reply