Your cart is currently empty!
Pro Deep Learning with Tensorflow 2.0: A Mathematical Approach to Advanced Artif
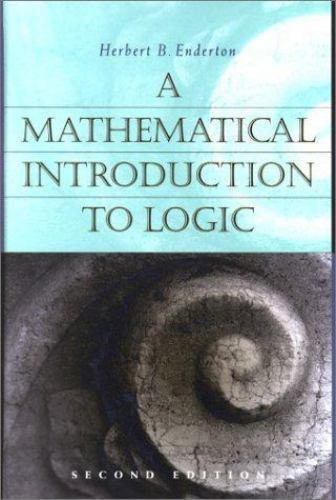
Pro Deep Learning with Tensorflow 2.0: A Mathematical Approach to Advanced Artif
Price : 59.88 – 49.90
Ends on : N/A
View on eBay
icial Intelligence
In this post, we will dive deep into the world of deep learning using Tensorflow 2.0, a powerful framework for building and training neural networks. We will take a mathematical approach to understanding the intricacies of advanced artificial intelligence and how it can be applied to various real-world problems.
Tensorflow 2.0 offers a wide range of tools and functionalities that make it easier than ever to build complex neural networks. With its intuitive API and improved performance, it has become the go-to choice for many deep learning practitioners.
To truly master deep learning with Tensorflow 2.0, one must have a solid understanding of the underlying mathematical principles. This includes topics such as linear algebra, calculus, probability theory, and optimization algorithms. By grasping these concepts, one can effectively design and train neural networks for a wide range of applications, from image recognition to natural language processing.
In this post, we will cover key mathematical concepts that are essential for deep learning, such as gradient descent, backpropagation, activation functions, and loss functions. We will also explore how these concepts are implemented in Tensorflow 2.0 and how they can be used to build and train advanced neural networks.
By taking a mathematical approach to deep learning with Tensorflow 2.0, you will gain a deeper understanding of how neural networks work and how they can be applied to solve complex problems. Whether you are a beginner or an experienced practitioner, this post will provide you with the knowledge and tools needed to take your AI skills to the next level.
#Pro #Deep #Learning #Tensorflow #Mathematical #Approach #Advanced #Artif
Leave a Reply