Your cart is currently empty!
Reinforcement Learning for Cyber-Physical Systems : With Cybersecurity Case…
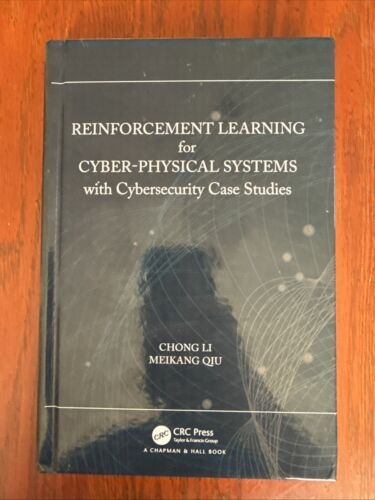
Reinforcement Learning for Cyber-Physical Systems : With Cybersecurity Case…
Price : 75.00
Ends on : N/A
View on eBay
Reinforcement Learning for Cyber-Physical Systems: With Cybersecurity Case Studies
In the world of cyber-physical systems, where physical processes are controlled and monitored by computer-based algorithms, the need for robust cybersecurity measures is more critical than ever. Traditional security approaches may not always be sufficient to protect these systems from evolving threats, leading researchers to explore new technologies such as reinforcement learning.
Reinforcement learning is a type of machine learning that enables an agent to learn how to make decisions by receiving feedback from its environment. This feedback, in the form of rewards or penalties, helps the agent to gradually improve its decision-making capabilities over time.
When applied to cybersecurity for cyber-physical systems, reinforcement learning can be used to detect and respond to anomalies in real-time, adapt to new attack strategies, and proactively defend against potential threats. By continuously learning and adjusting its strategies, an AI-powered cybersecurity system can enhance the resilience of critical infrastructure and prevent catastrophic events.
To showcase the potential of reinforcement learning in cybersecurity for cyber-physical systems, let’s consider a hypothetical case study. Imagine a smart grid that controls the distribution of electricity to homes and businesses. An attacker gains unauthorized access to the grid’s control system and attempts to manipulate the flow of electricity to cause a blackout.
Using reinforcement learning algorithms, the cybersecurity system can analyze the grid’s data in real-time, identify abnormal patterns in energy consumption, and predict potential attack scenarios. By leveraging this predictive capability, the system can take proactive measures to block unauthorized access, isolate compromised components, and prevent the attacker from causing widespread disruption.
In conclusion, reinforcement learning offers a promising approach to enhancing cybersecurity for cyber-physical systems, enabling proactive defense mechanisms and adaptive responses to emerging threats. By harnessing the power of AI, organizations can strengthen the resilience of their critical infrastructure and mitigate the risks of cyber attacks.
#Reinforcement #Learning #CyberPhysical #Systems #Cybersecurity #Case..
Leave a Reply