Your cart is currently empty!
Reliable Machine Learning Applying SRE Principles to ML in Production New Stock
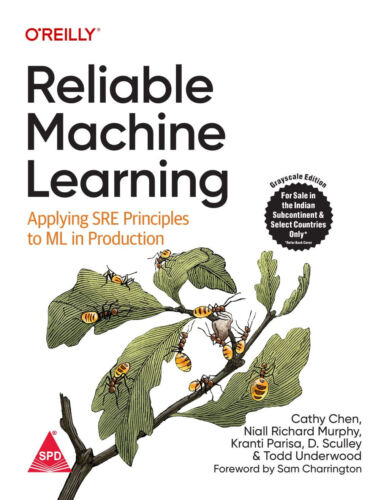
Reliable Machine Learning Applying SRE Principles to ML in Production New Stock
Price : 57.99
Ends on : N/A
View on eBay
Introduction:
Machine learning (ML) is revolutionizing industries by enabling companies to leverage data-driven insights for decision-making. However, deploying ML models into production environments can be challenging due to the complexity of managing and maintaining these models over time. Site Reliability Engineering (SRE) principles can help ensure the reliability and scalability of machine learning systems in production.
Reliable Machine Learning with SRE Principles:
SRE principles, popularized by Google, focus on ensuring the reliability and scalability of systems in production environments. By applying these principles to machine learning systems, companies can improve the performance and stability of their ML models. Some key SRE principles that can be applied to machine learning in production include:
1. Monitoring and alerting: Implementing robust monitoring and alerting systems for machine learning models can help detect issues early and prevent system failures. By monitoring key metrics such as model performance, data quality, and resource utilization, teams can proactively address potential problems before they impact users.
2. Incident response: Developing a comprehensive incident response plan for machine learning systems can help teams quickly identify and resolve issues when they occur. By establishing clear protocols for responding to incidents, teams can minimize downtime and maintain system reliability.
3. Capacity planning: Proper capacity planning is essential for ensuring the scalability of machine learning systems in production. By forecasting resource requirements and scaling infrastructure accordingly, teams can avoid performance bottlenecks and maintain system reliability under varying workloads.
New Stock:
Incorporating SRE principles into machine learning systems can help companies deploy and maintain reliable ML models in production. By leveraging monitoring and alerting, incident response, and capacity planning strategies, teams can ensure the performance and scalability of their ML systems over time. Investing in reliable machine learning powered by SRE principles can help companies gain a competitive edge in today’s data-driven landscape.
Conclusion:
As companies increasingly rely on machine learning for critical business decisions, ensuring the reliability and scalability of ML systems in production is essential. By applying SRE principles to machine learning, companies can build robust and resilient systems that deliver reliable performance over time. Investing in reliable machine learning powered by SRE principles is a strategic move that can help companies unlock the full potential of their data and drive business growth.
#Reliable #Machine #Learning #Applying #SRE #Principles #Production #Stock
Leave a Reply