Your cart is currently empty!
The Evolution of DNN: From Theory to Real-world Applications
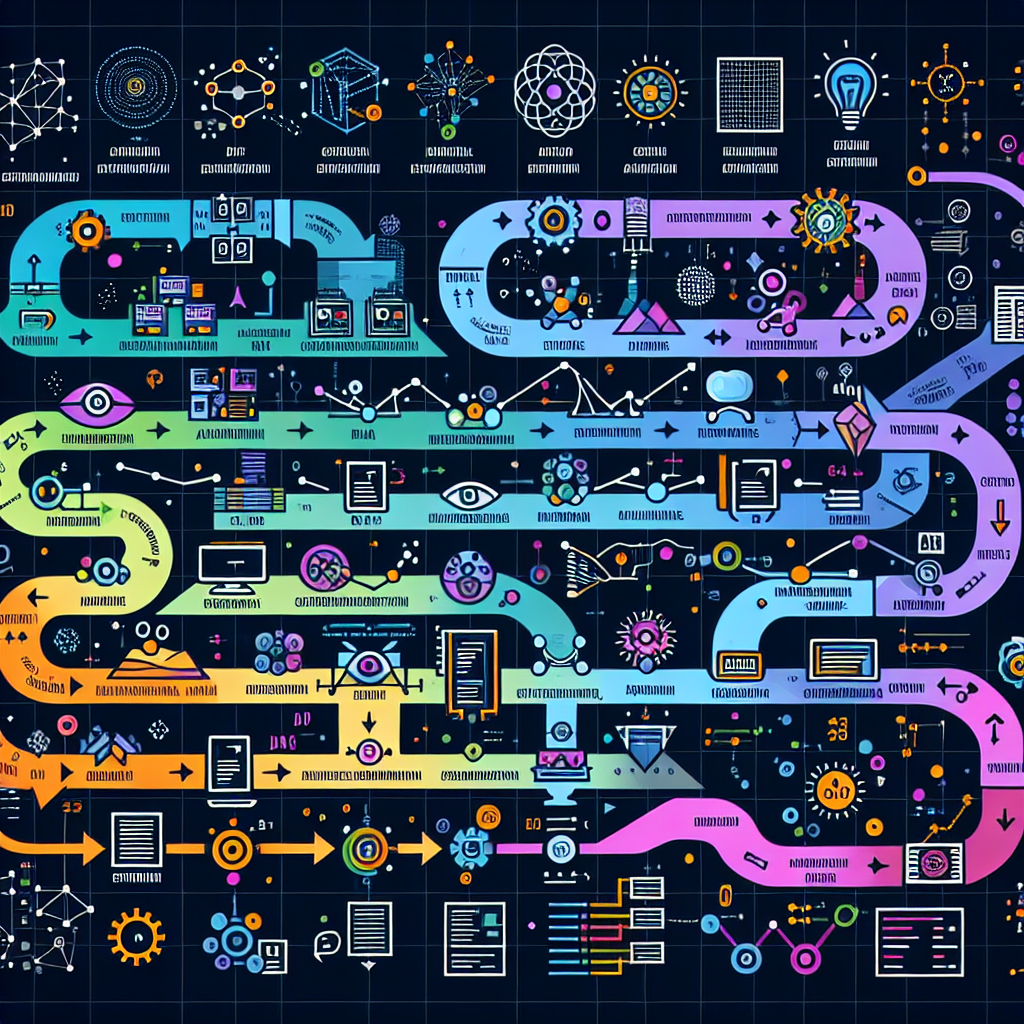
Deep Neural Networks (DNNs) have come a long way since their inception, evolving from theoretical concepts to powerful tools with real-world applications across various industries. In this article, we will explore the evolution of DNNs and how they have transformed the way we approach complex problems in fields such as image recognition, natural language processing, and healthcare.
DNNs, also known as deep learning models, are a type of artificial neural network with multiple layers of interconnected nodes that can learn complex patterns in data. The idea of using multiple layers of neurons in a neural network dates back to the 1940s, but it wasn’t until the early 2010s that DNNs gained widespread popularity due to advancements in computational power and the availability of large datasets.
One of the key breakthroughs in the evolution of DNNs was the development of the convolutional neural network (CNN) architecture, which has revolutionized the field of computer vision. CNNs are specifically designed to process visual data by extracting features at different levels of abstraction, making them ideal for tasks such as image classification, object detection, and facial recognition.
Another significant milestone in the evolution of DNNs was the introduction of recurrent neural networks (RNNs) and long short-term memory (LSTM) networks, which excel at processing sequential data such as natural language text and time-series data. These models have revolutionized the field of natural language processing, enabling advancements in machine translation, sentiment analysis, and speech recognition.
In recent years, DNNs have been applied to a wide range of real-world problems, demonstrating their effectiveness in areas such as healthcare, finance, and autonomous driving. For example, DNNs have been used to analyze medical images for early detection of diseases, predict stock prices based on historical data, and navigate self-driving cars through complex environments.
The evolution of DNNs has been driven by a combination of theoretical advancements in deep learning algorithms and practical insights gained from applying these models to real-world datasets. Researchers and practitioners continue to push the boundaries of what is possible with DNNs, exploring new architectures, optimization techniques, and applications to tackle some of the most challenging problems facing society today.
As DNNs continue to evolve, it is clear that they will play an increasingly important role in shaping the future of artificial intelligence and machine learning. From theory to real-world applications, the journey of DNNs has been a remarkable one, and the possibilities for future advancements are truly exciting.
#Evolution #DNN #Theory #Realworld #Applications,dnn
Leave a Reply