Your cart is currently empty!
The Evolution of GANs in NLP: A Survey of Recent Developments
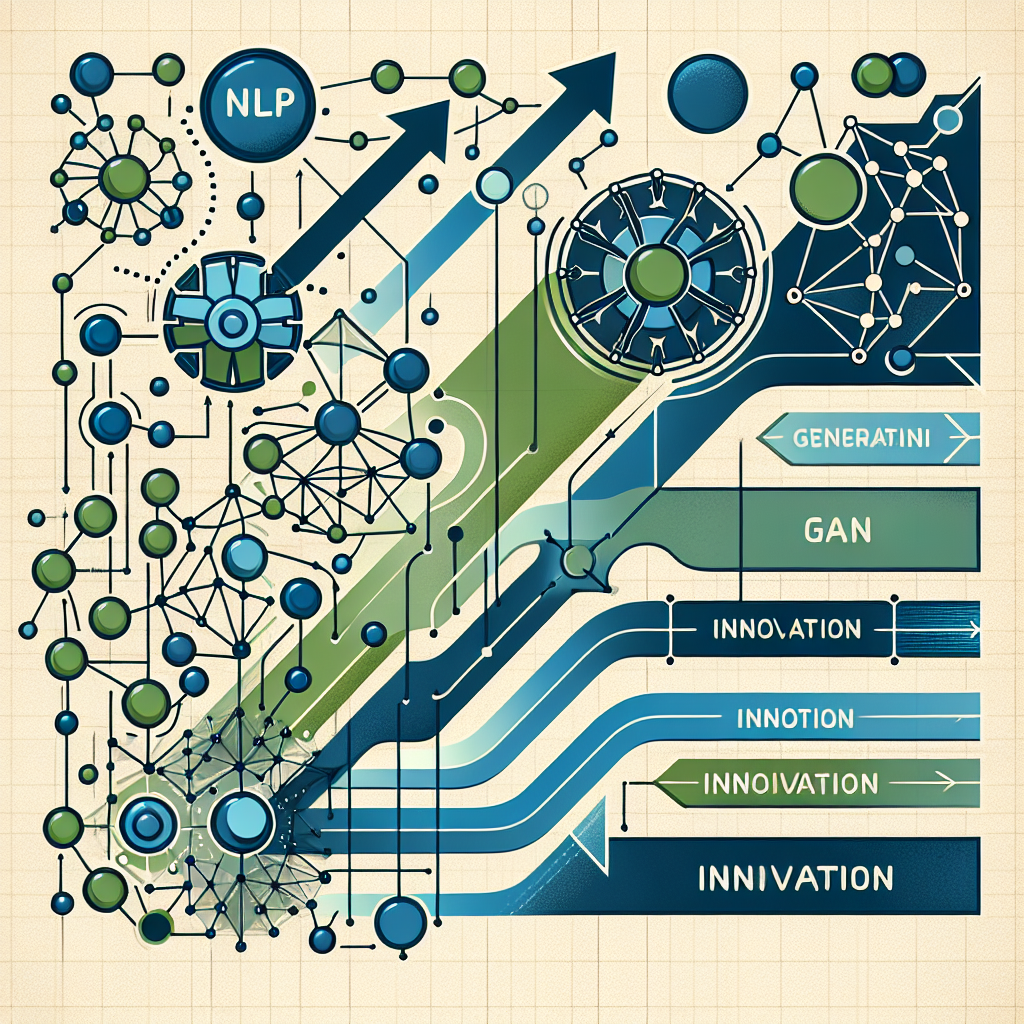
Generative Adversarial Networks (GANs) have been revolutionizing the field of Natural Language Processing (NLP) in recent years, offering novel approaches to text generation, translation, and other tasks. In this article, we will explore the evolution of GANs in NLP and survey some of the recent developments in the field.
GANs were originally introduced by Ian Goodfellow and his colleagues in 2014 as a novel framework for training generative models. In a typical GAN setup, two neural networks – a generator and a discriminator – are pitted against each other in a game-theoretic setting. The generator is trained to generate realistic samples, while the discriminator is trained to distinguish between real and fake samples. Through this adversarial training process, the generator learns to produce more realistic samples over time.
In the context of NLP, GANs have been applied to a wide range of tasks, including text generation, paraphrasing, machine translation, and style transfer. One of the key advantages of using GANs in NLP is their ability to generate diverse and high-quality text samples, which can be particularly useful in creative writing, dialogue systems, and data augmentation.
Recent developments in GANs for NLP have focused on overcoming some of the limitations of early models, such as mode collapse and training instability. One promising approach is the use of self-attention mechanisms, which allow the generator to focus on different parts of the input sequence and capture long-range dependencies. Another key development is the use of reinforcement learning techniques to guide the generator towards producing more coherent and fluent text.
In addition to improving the quality of generated text, researchers have also explored ways to incorporate GANs into downstream NLP tasks, such as text classification, sentiment analysis, and machine comprehension. By leveraging the generative capabilities of GANs, researchers have been able to enhance the performance of traditional NLP models and achieve state-of-the-art results on various benchmark datasets.
Looking ahead, the future of GANs in NLP looks promising, with ongoing research efforts focusing on scalability, interpretability, and robustness. By addressing these challenges, GANs have the potential to further advance the capabilities of NLP systems and enable new applications in areas such as conversational AI, content generation, and personalized recommendation.
In conclusion, the evolution of GANs in NLP has opened up new possibilities for text generation and understanding. With continued research and development, we can expect to see even more exciting advancements in the field in the years to come.
#Evolution #GANs #NLP #Survey #Developments,gan)
to natural language processing (nlp) pdf
Leave a Reply