Your cart is currently empty!
The Future of LSTM: Emerging Trends and Innovations in Deep Learning
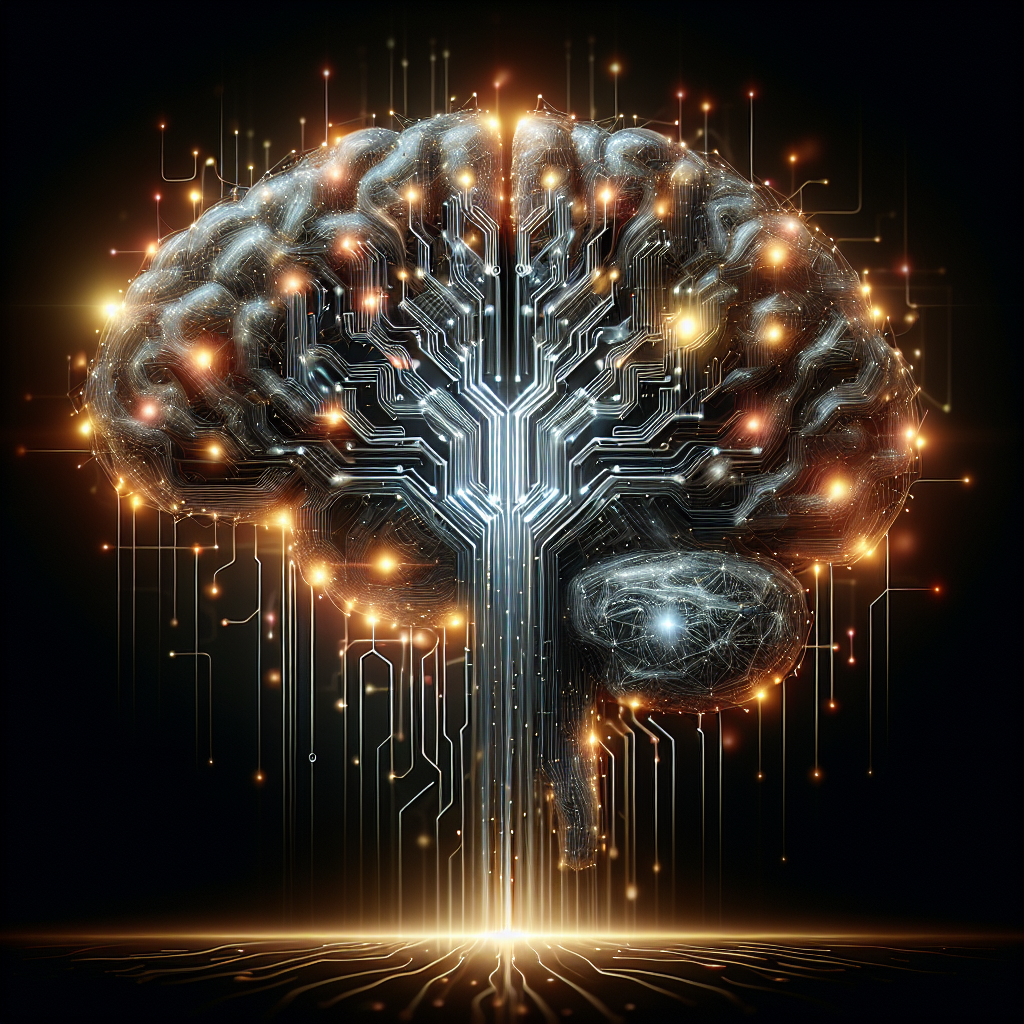
Long Short-Term Memory (LSTM) is a type of recurrent neural network (RNN) that has gained popularity in the field of deep learning for its ability to learn long-term dependencies in sequential data. Originally introduced in 1997 by Sepp Hochreiter and Jürgen Schmidhuber, LSTM has since become a key component in many state-of-the-art models for tasks such as speech recognition, machine translation, and time series prediction.
As deep learning continues to evolve, researchers are constantly exploring new ways to improve the performance and efficiency of LSTM models. One emerging trend in LSTM research is the development of more sophisticated architectures that can capture complex patterns in data more effectively. For example, researchers have been experimenting with attention mechanisms, which allow the model to focus on specific parts of the input sequence that are most relevant to the task at hand. This has led to significant improvements in the performance of LSTM models on tasks such as machine translation and image captioning.
Another area of innovation in LSTM research is the use of reinforcement learning to train the model. Reinforcement learning involves training the model to maximize a reward signal by interacting with its environment, which can lead to more robust and adaptive models. Researchers have shown that combining LSTM with reinforcement learning can lead to better performance on tasks such as video game playing and robotic control.
In addition to these architectural improvements, researchers are also exploring ways to make LSTM models more efficient and scalable. One approach is to use techniques such as pruning, quantization, and distillation to reduce the size of the model while maintaining its performance. This can be particularly important for deploying LSTM models on resource-constrained devices such as mobile phones and IoT devices.
Overall, the future of LSTM looks bright, with researchers continuing to push the boundaries of what is possible with this powerful deep learning technique. By exploring new architectures, training methods, and optimization techniques, we can expect to see even more impressive applications of LSTM in the years to come. Whether it’s improving speech recognition accuracy, enhancing machine translation capabilities, or enabling more efficient robotic control, LSTM is sure to play a key role in shaping the future of deep learning.
#Future #LSTM #Emerging #Trends #Innovations #Deep #Learning,lstm
Leave a Reply