Your cart is currently empty!
The Future of Recommendation Systems: A Deep Dive into GNN
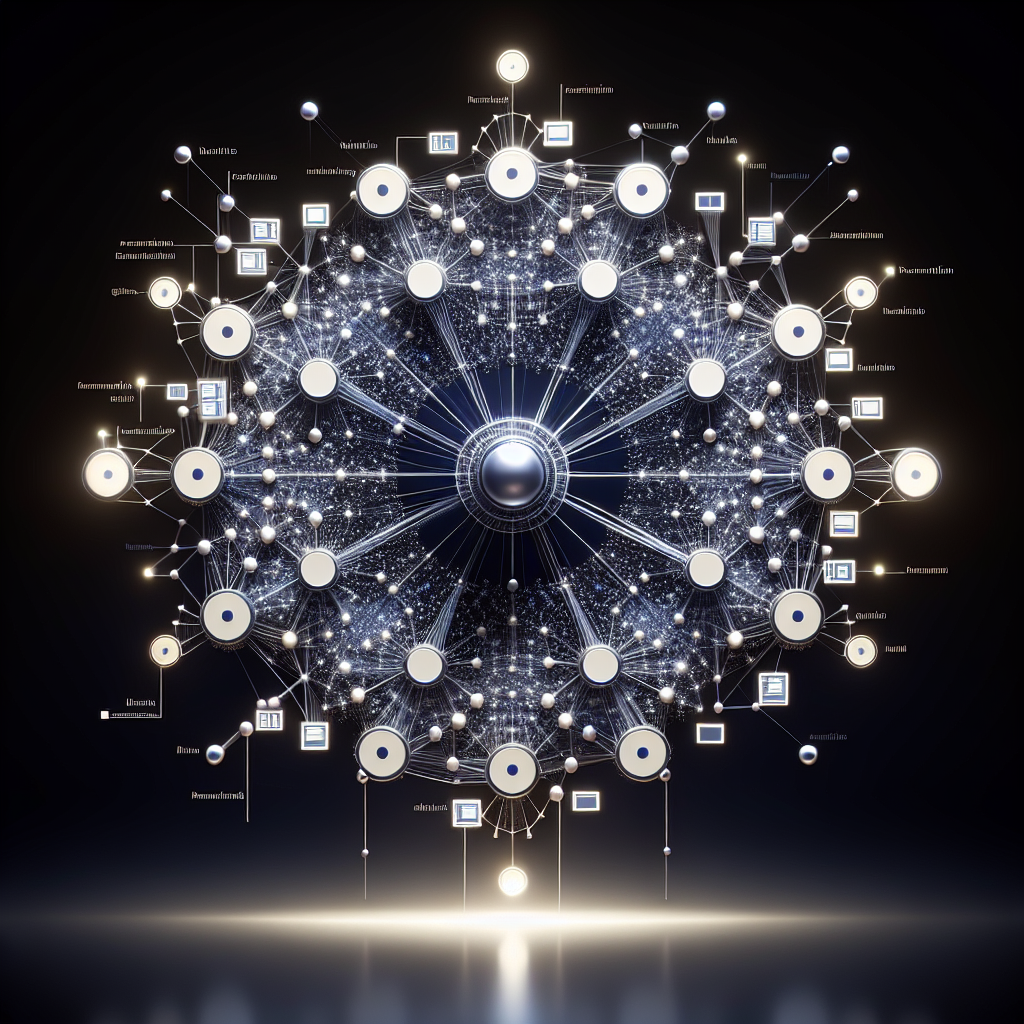
Recommendation systems have become an integral part of our daily lives, helping us discover new products, services, and content based on our preferences and past interactions. These systems leverage machine learning algorithms to analyze user behavior and make personalized recommendations. However, traditional recommendation systems often face challenges in capturing complex user preferences and relationships.
Graph Neural Networks (GNNs) are a cutting-edge technology that holds promise for revolutionizing recommendation systems. GNNs are a type of neural network that can effectively model relationships between entities in a graph structure, such as users and items in a recommendation system. By leveraging the power of graph-based representations, GNNs can capture intricate user-item interactions and provide more accurate and personalized recommendations.
One of the key advantages of GNNs is their ability to learn from both the structure of the graph and the features of the nodes. This enables GNNs to capture higher-order relationships and dependencies between entities, leading to more comprehensive and context-aware recommendations. Additionally, GNNs can adapt to dynamic graphs, making them suitable for recommendation systems that evolve over time.
Another benefit of GNNs is their ability to incorporate side information and auxiliary data sources. By leveraging additional features such as user demographics, social connections, and item attributes, GNNs can enhance the recommendation process and provide more relevant suggestions to users. This multi-modal approach enables GNNs to handle diverse data types and improve recommendation accuracy.
Furthermore, GNNs can address the cold-start problem, which arises when new items or users have limited interaction data. By leveraging the graph structure and side information, GNNs can effectively generalize recommendations for unseen entities and mitigate the impact of data sparsity. This enables recommendation systems to provide personalized suggestions even for new users or niche items.
In conclusion, Graph Neural Networks offer a promising future for recommendation systems by leveraging graph-based representations, incorporating side information, and addressing the cold-start problem. As GNNs continue to advance, we can expect more accurate, personalized, and context-aware recommendations that enhance user experience and drive business success. The integration of GNNs into recommendation systems represents a significant step towards unlocking the full potential of machine learning in personalized content discovery.
#Future #Recommendation #Systems #Deep #Dive #GNN,gnn
Leave a Reply