Your cart is currently empty!
The Impact of Deep Neural Networks on Machine Learning and Data Science
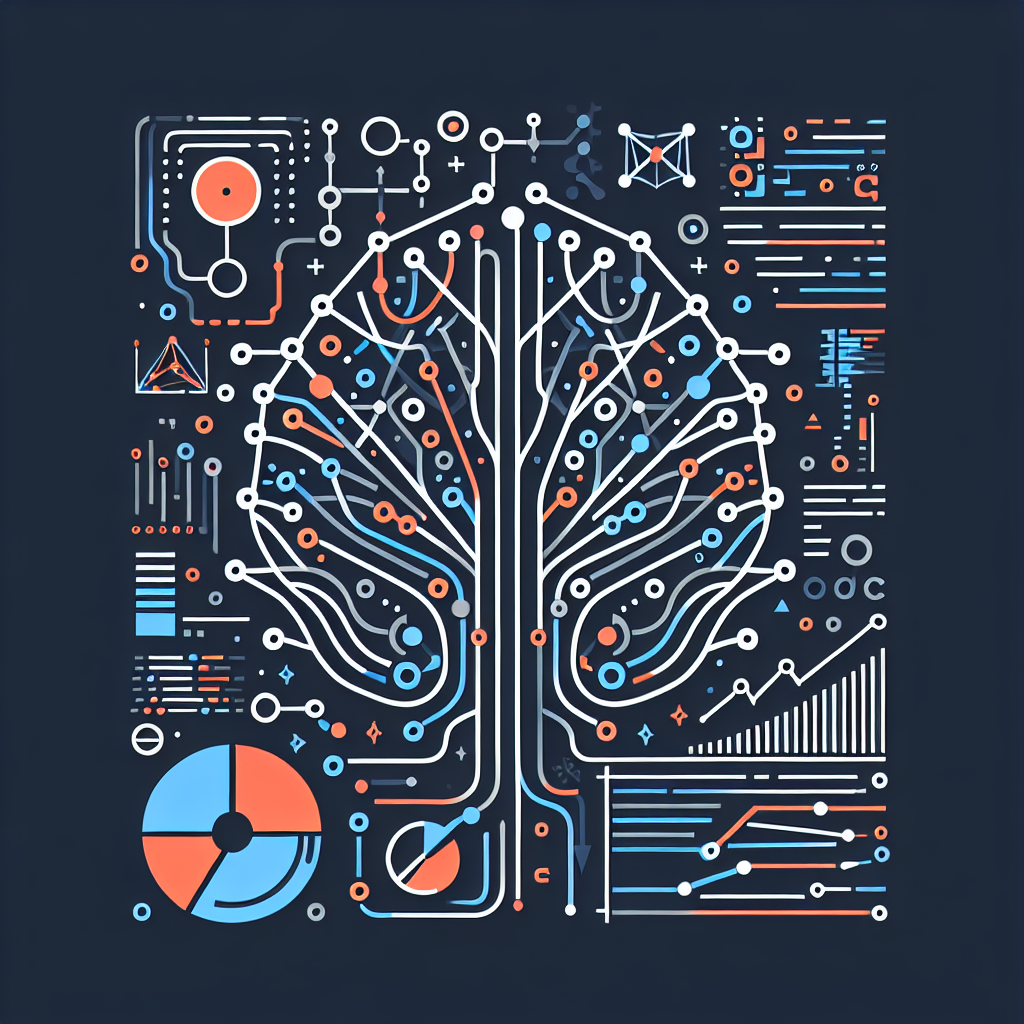
Deep neural networks have revolutionized the field of machine learning and data science, offering unprecedented levels of accuracy and efficiency in solving complex problems. These powerful algorithms have had a significant impact on various industries, from healthcare to finance, by enabling more advanced data analysis and predictive modeling.
One of the key advantages of deep neural networks is their ability to learn complex patterns and relationships in data, making them particularly effective for tasks such as image recognition, natural language processing, and speech recognition. Traditional machine learning algorithms, such as linear regression or decision trees, often struggle with high-dimensional data or non-linear relationships. Deep neural networks, on the other hand, can automatically extract features from raw data and learn intricate patterns that may be difficult to discern with human eyes.
Moreover, deep neural networks have also improved the accuracy and performance of machine learning models. By using multiple layers of interconnected neurons, these networks can capture hierarchical representations of data, allowing them to make more accurate predictions and classifications. This has led to significant advancements in areas such as medical diagnosis, fraud detection, and personalized recommendations.
In addition, deep neural networks have made it easier to work with unstructured data, such as images, text, and audio. By leveraging convolutional neural networks (CNNs) and recurrent neural networks (RNNs), researchers and data scientists can extract meaningful information from these types of data and use it to make informed decisions. For example, CNNs have been instrumental in improving the accuracy of image recognition systems, while RNNs have been used to generate human-like text and speech.
Despite their many advantages, deep neural networks also pose some challenges. One of the main issues is their complexity and black-box nature, which can make it difficult to interpret and explain their decisions. This has raised concerns about the potential biases and errors that may arise from using these algorithms in critical applications, such as criminal justice or healthcare.
Another challenge is the need for large amounts of labeled data to train deep neural networks effectively. Collecting and annotating data can be time-consuming and expensive, especially for tasks that require specialized knowledge or expertise. Additionally, deep neural networks are computationally intensive and require powerful hardware, such as GPUs or TPUs, to train and deploy.
Despite these challenges, the impact of deep neural networks on machine learning and data science cannot be overstated. These algorithms have enabled breakthroughs in areas such as computer vision, natural language processing, and reinforcement learning, paving the way for new applications and innovations. As researchers continue to explore the capabilities of deep neural networks, we can expect to see even more exciting developments in the field of artificial intelligence.
#Impact #Deep #Neural #Networks #Machine #Learning #Data #Science,dnn
Leave a Reply