Your cart is currently empty!
The Intersection of Gan and NLP: Advancements in Text Generation
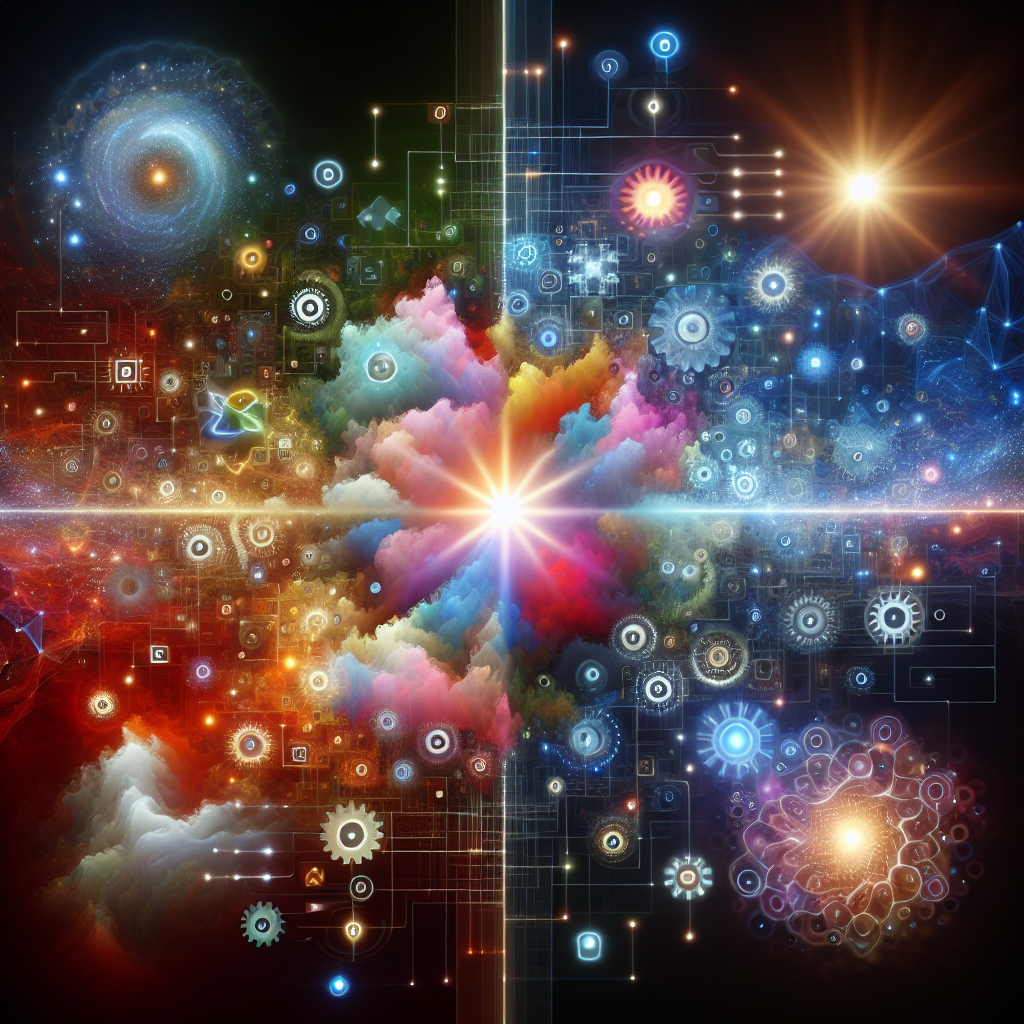
The Intersection of GAN and NLP: Advancements in Text Generation
Generative Adversarial Networks (GANs) and Natural Language Processing (NLP) are two cutting-edge technologies that have been making waves in the field of artificial intelligence. While GANs are primarily used for generating realistic images, NLP focuses on understanding and generating human language. In recent years, researchers have started exploring the intersection of these two technologies to create advanced text generation models that can produce coherent and contextually relevant text.
One of the key challenges in text generation is maintaining coherence and context throughout the generated text. Traditional language models, such as recurrent neural networks (RNNs) and transformers, often struggle with generating text that is both grammatically correct and contextually relevant. This is where GANs come in – by incorporating a discriminator network that evaluates the text generated by the generator network, GAN-based text generation models can produce more coherent and contextually relevant text.
One of the most popular approaches to GAN-based text generation is the use of adversarial training, where the generator network is trained to generate text that is indistinguishable from human-written text, while the discriminator network is trained to differentiate between human-written and machine-generated text. This adversarial training process helps the generator network learn to generate text that is not only grammatically correct but also contextually relevant.
Another key advancement in GAN-based text generation is the use of conditional GANs, where the generator network is conditioned on a specific input, such as a prompt or a topic. This allows the generator network to generate text that is relevant to the input, making it more suitable for tasks such as text summarization or dialogue generation.
Researchers have also explored the use of pre-trained language models, such as GPT-3, in conjunction with GANs to improve text generation performance. By fine-tuning a pre-trained language model using GAN-based training techniques, researchers have been able to achieve state-of-the-art results in text generation tasks.
Overall, the intersection of GANs and NLP has led to significant advancements in text generation technology. By leveraging the strengths of both GANs and NLP, researchers have been able to create text generation models that are more coherent, contextually relevant, and human-like. As these technologies continue to evolve, we can expect even more exciting developments in the field of text generation.
#Intersection #Gan #NLP #Advancements #Text #Generation,gan)
to natural language processing (nlp) pdf
Leave a Reply