Your cart is currently empty!
The Power of GANs in Text Generation for Natural Language Processing (NLP)
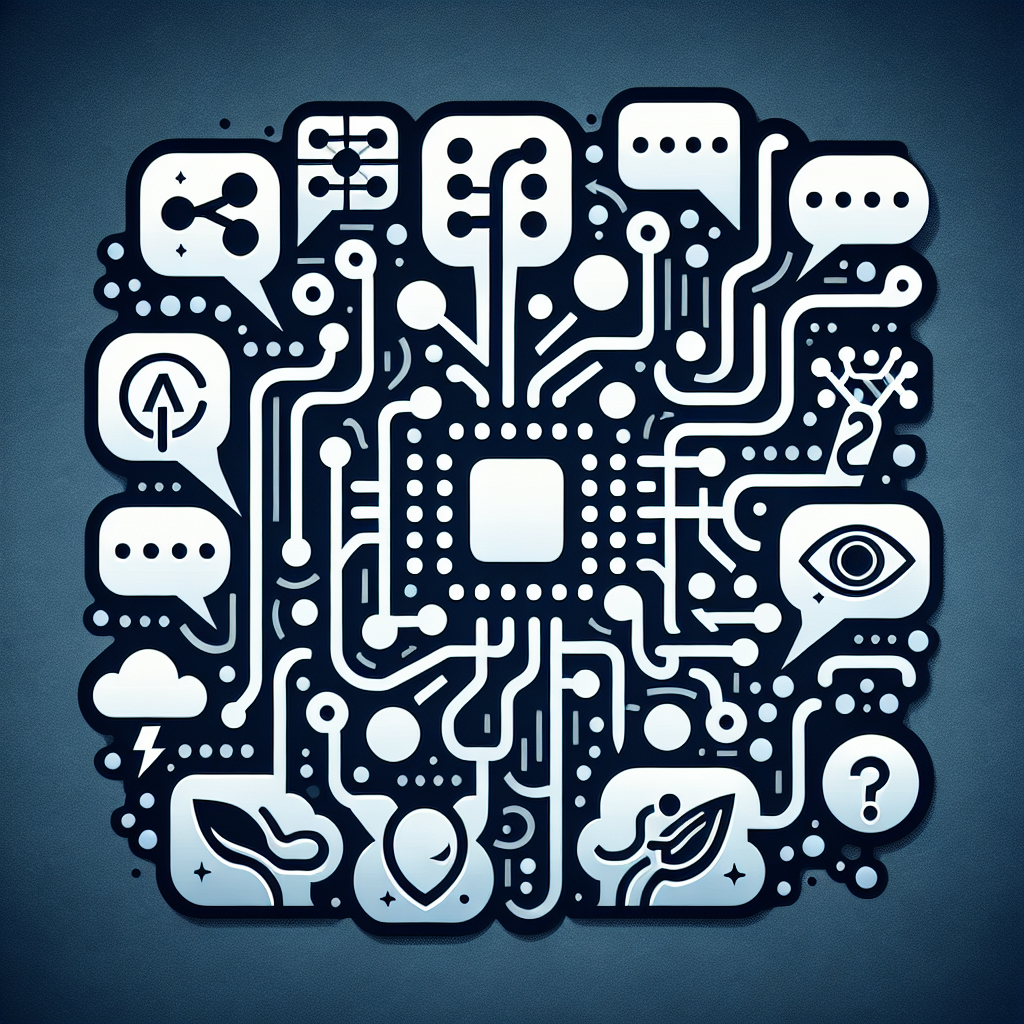
Generative Adversarial Networks (GANs) have revolutionized the field of artificial intelligence by providing a powerful framework for generating realistic and high-quality data. While GANs are most commonly associated with the generation of images and videos, they have also shown great promise in the field of natural language processing (NLP), particularly in text generation.
Text generation is a key task in NLP, with applications ranging from chatbots and virtual assistants to machine translation and content creation. Traditional methods of text generation often rely on rule-based systems or statistical models, which can produce somewhat predictable and repetitive results. GANs, on the other hand, offer a more sophisticated approach by training two neural networks – a generator and a discriminator – in a competitive manner.
The generator network is tasked with creating new text samples, while the discriminator network evaluates the generated samples and provides feedback to the generator. Through this adversarial training process, the generator learns to produce realistic and coherent text that closely resembles human-written content. This iterative process of generating, evaluating, and refining text samples allows GANs to capture the complex patterns and structures of natural language.
One of the key advantages of GANs in text generation is their ability to generate diverse and creative output. Traditional language models often struggle with producing varied and innovative text, leading to repetitive and monotonous results. GANs, on the other hand, can generate a wide range of text styles, tones, and topics, making them ideal for tasks that require creativity and originality.
Furthermore, GANs have shown great potential in improving the quality and fluency of generated text. By training on large datasets of human-written text, GANs can learn to mimic the style and structure of natural language, producing text that is grammatically correct, coherent, and contextually relevant. This makes GANs particularly well-suited for tasks such as text summarization, paraphrasing, and dialogue generation.
Despite their many advantages, GANs also present challenges in text generation, such as mode collapse (where the generator produces repetitive or limited output) and training instability. Researchers are actively working to address these issues and improve the performance of GANs in NLP tasks.
In conclusion, the power of GANs in text generation for NLP is undeniable. Their ability to generate diverse, creative, and high-quality text makes them a valuable tool for a wide range of applications in natural language processing. As researchers continue to explore and refine the capabilities of GANs in text generation, we can expect to see even more exciting advancements in the field of NLP.
#Power #GANs #Text #Generation #Natural #Language #Processing #NLP,gan)
to natural language processing (nlp) pdf
Leave a Reply