Your cart is currently empty!
The Role of Gated Architectures in Overcoming the Vanishing Gradient Problem in RNNs
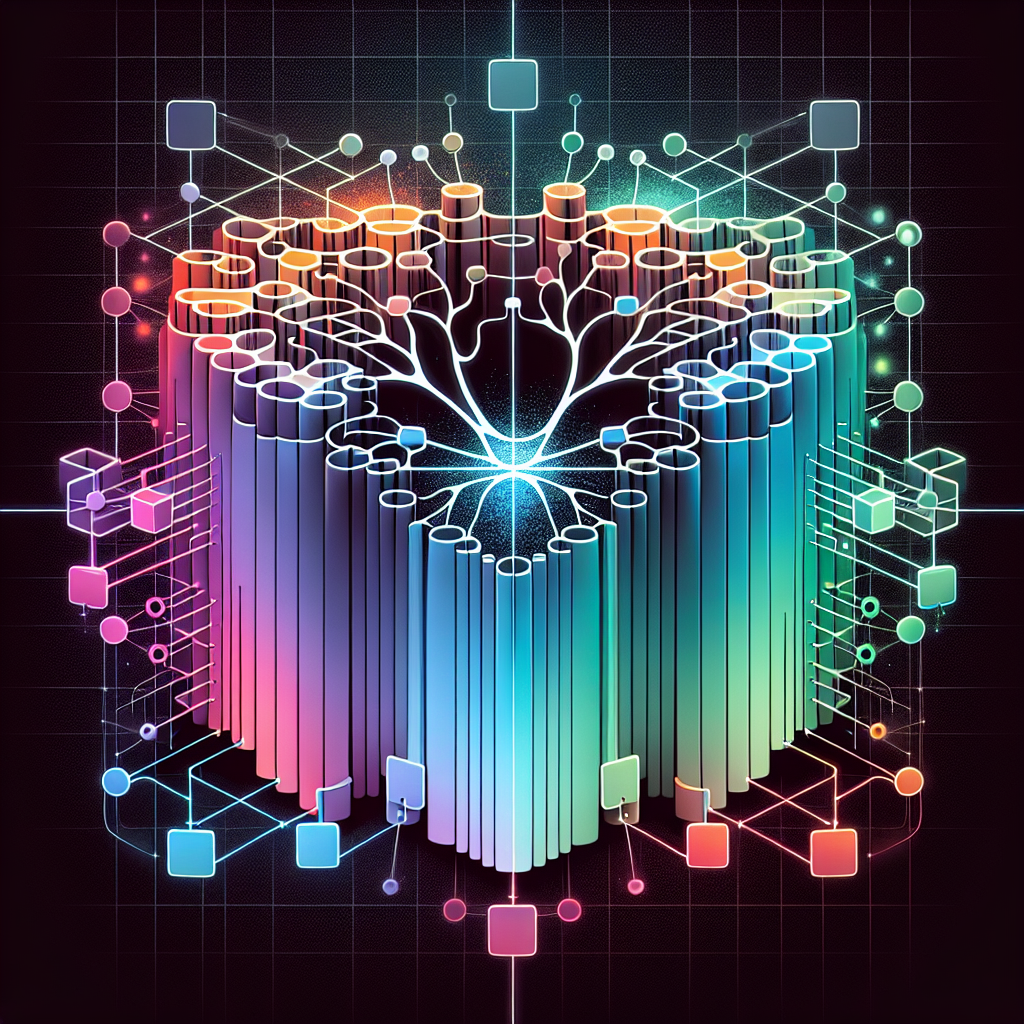
Recurrent Neural Networks (RNNs) are a powerful type of artificial neural network that is commonly used in natural language processing, speech recognition, and other sequential data analysis tasks. However, RNNs are prone to the vanishing gradient problem, which can hinder their ability to learn long-term dependencies in data.
The vanishing gradient problem occurs when the gradients of the loss function with respect to the network’s parameters become very small as they are backpropagated through time. This can cause the weights of the network to become stagnant or even vanish altogether, making it difficult for the network to learn from distant past information.
One approach to overcoming the vanishing gradient problem in RNNs is the use of gated architectures, such as Long Short-Term Memory (LSTM) and Gated Recurrent Unit (GRU) networks. These architectures incorporate specialized gating mechanisms that control the flow of information through the network, allowing them to better capture long-term dependencies in data.
In LSTM networks, for example, each cell has three gates – the input gate, forget gate, and output gate – that regulate the flow of information. The input gate controls the flow of new information into the cell, the forget gate determines which information to discard from the cell’s memory, and the output gate decides which information to pass on to the next time step.
Similarly, GRU networks have two gates – the reset gate and update gate – that control the flow of information in a similar manner. The reset gate determines how much of the past information to forget, while the update gate decides how much of the new information to incorporate.
By incorporating these gating mechanisms, LSTM and GRU networks are able to maintain a more stable gradient flow during training, allowing them to effectively learn long-term dependencies in data. This makes them well-suited for tasks that require capturing complex sequential patterns, such as machine translation and speech recognition.
In conclusion, gated architectures play a crucial role in overcoming the vanishing gradient problem in RNNs. By incorporating specialized gating mechanisms, such as those found in LSTM and GRU networks, RNNs are able to effectively capture long-term dependencies in data and achieve superior performance in various sequential data analysis tasks.
#Role #Gated #Architectures #Overcoming #Vanishing #Gradient #Problem #RNNs,recurrent neural networks: from simple to gated architectures
Leave a Reply